A Gaussian Mixture Unscented Rauch-Tung-Striebel Smoothing Framework for Trajectory Reconstruction
IEEE TRANSACTIONS ON INDUSTRIAL INFORMATICS(2024)
摘要
Trajectory reconstruction (TR) plays an important role in practical applications. The data collected for TR are often contaminated with non-Gaussian noise due to environmental factors, which reduces the accuracy of TR. This study proposes a novel method to suppress the effects of non-Gaussian measurement noise. The method consists of two steps: decomposing the measurement noise and performing a weighted fusion of the relevant states. In the first step, the measurement noise is decomposed into a weighted combination of multiple Gaussian distributions using the Gaussian mixture model. In the second step, the interacting multiple model is employed to perform the weighted fusion of the relevant states. The main idea of the proposed method is to transform the state estimation problem from a non-Gaussian and nonlinear case into a state estimation problem in the nonlinear and Gaussian case. Based on this idea, a new unscented Kalman filter and an unscented Rauch-Tung-Striebel smoother framework are developed. The TR simulations and experiments are conducted for an underwater unmanned vehicle to verify the effectiveness and superiority of the proposed algorithms. The results demonstrate that the performance of the proposed algorithms is significantly better than that of the prominent existing algorithms, especially in the presence of non-Gaussian noise.
更多查看译文
关键词
Gaussian mixture model (GMM),interacting multiple model (IMM),non-Gaussian noise,unscented Kalman filter (UKF),unscented Rauch-Tung-Striebel (URTS) smoother
AI 理解论文
溯源树
样例
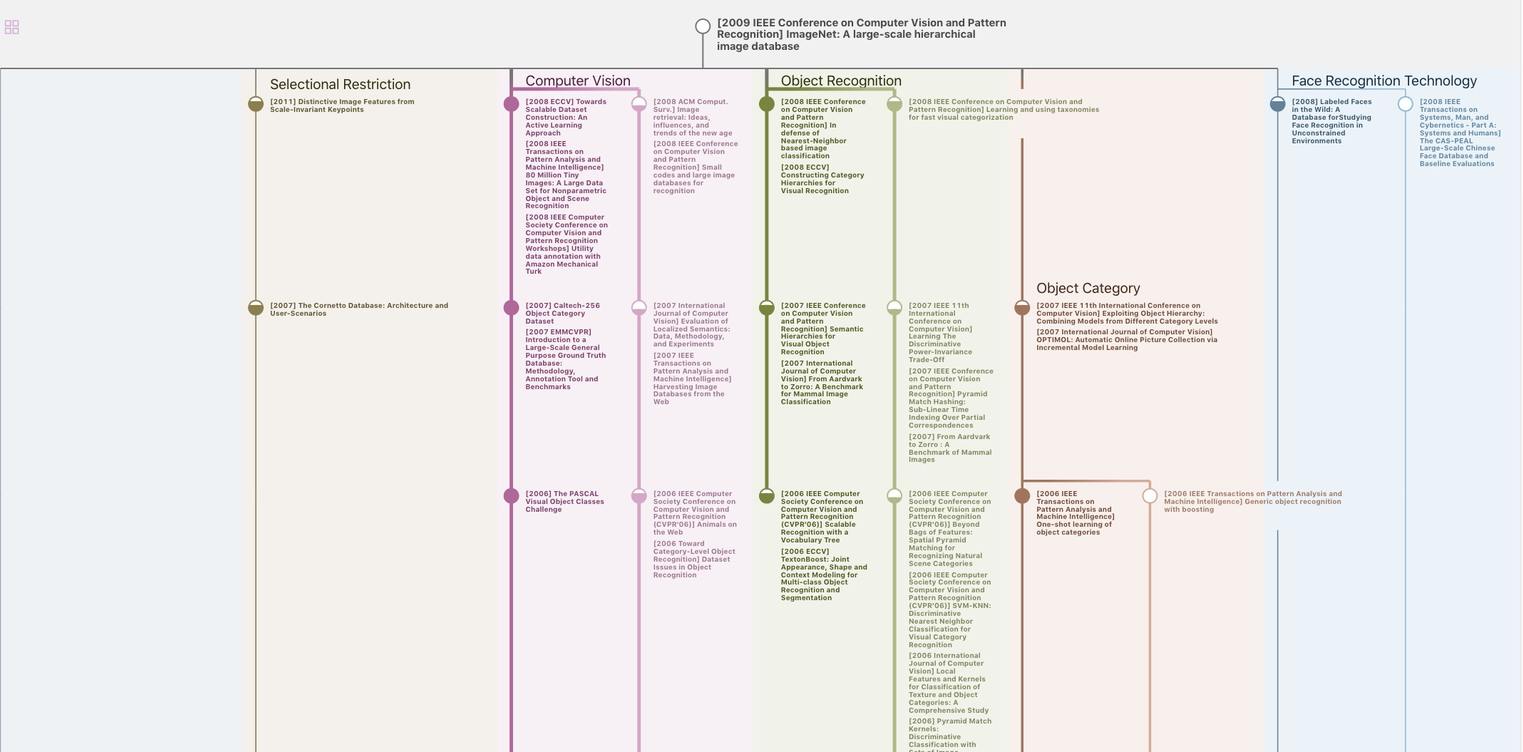
生成溯源树,研究论文发展脉络
Chat Paper
正在生成论文摘要