Unsupervised Scene Analysis Using Semiparametric Bayesian Models
semanticscholar(2012)
摘要
Robots operating in domestic environments need to deal with a variety of different objects. Usually, these objects are not placed randomly. For example, objects on a breakfast table such as plates and knives typically occur in recurrent configurations. We propose a novel hierarchical generative model to infer the latent groups of objects in a scene. The proposed model is a combination of Dirichlet processes and beta processes, which allows for a rigorous probabilistic treatment of the unknown dimensionality of the parameter space. We address a set of different tasks in scene understanding ranging from unsupervised scene segmentation to generation of a new scene and completion of a partially specified scene. We use Markov chain Monte Carlo (MCMC) techniques for inference and present experiments with simulated as well as real-world data obtained from a Kinect RGB-D camera.
更多查看译文
AI 理解论文
溯源树
样例
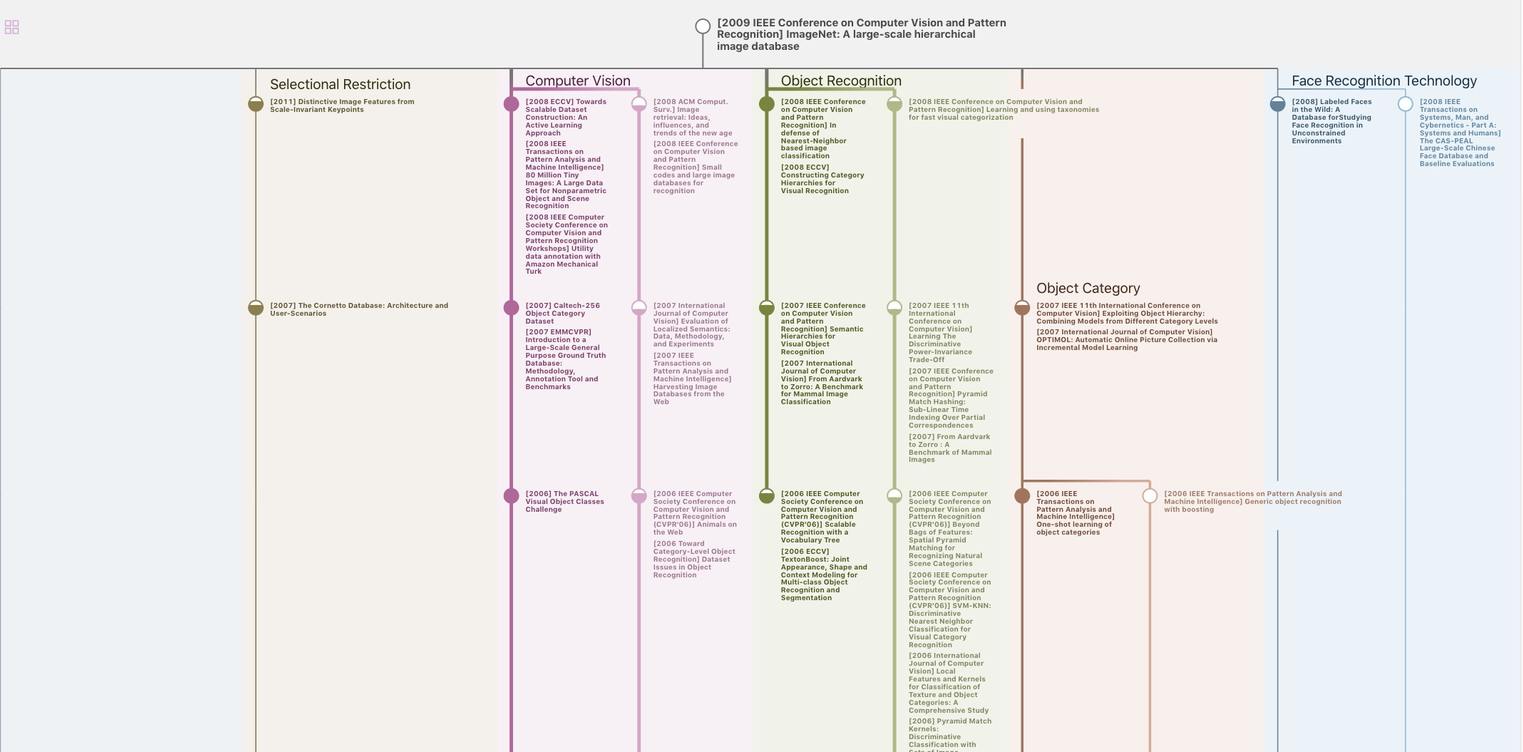
生成溯源树,研究论文发展脉络
Chat Paper
正在生成论文摘要