Convoluted mixture of deep experts for robust semantic segmentation
IEEE/RSJ International conference on intelligent robots and systems (IROS) workshop, state estimation and terrain perception for all terrain mobile robots(2016)
摘要
Robust scene understanding of outdoor environments using passive optical sensors is a critical problem characterized by changing conditions throughout the day and across seasons. The perception models on a robot should be able learn features impervious to these factors in order to be operable in the real-world. In this paper, we propose a convoluted mixture of deep experts (CMoDE) model that enables a multistream deep neural network architecture to learn features from complementary modalities and spectra that are resilient to commonly observed environmental disturbances. Our model first adaptively weighs features from each of the individual experts and then further learns fused representations that are robust to these disturbances namely shadows, snow, rain, glare and motion blur. We comprehensively evaluate the CMoDE model against several other existing fusion approaches and show that our proposed model exceeds the state-of-the-art.
更多查看译文
AI 理解论文
溯源树
样例
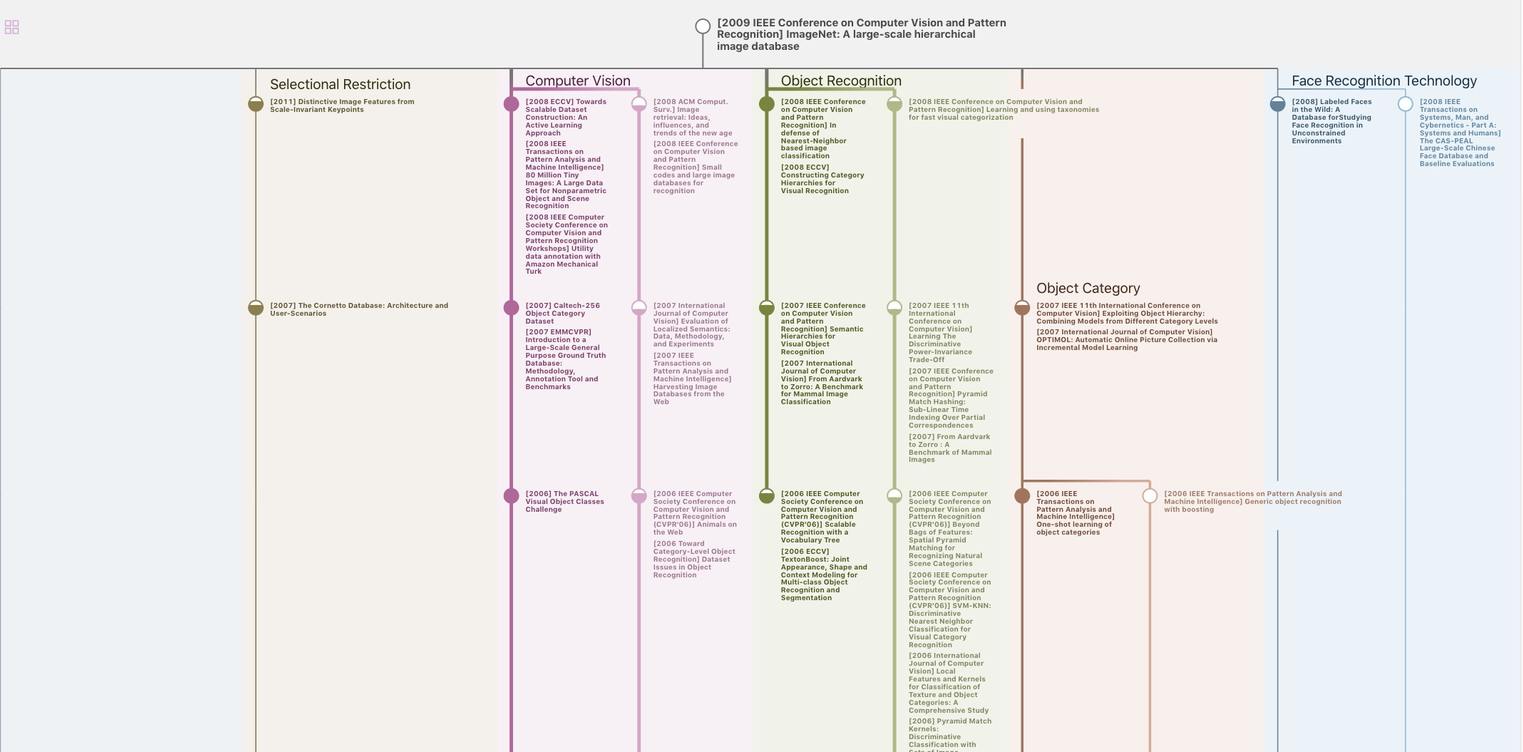
生成溯源树,研究论文发展脉络
Chat Paper
正在生成论文摘要