Regularized Content-Aware Tensor Factorization Meets Temporal-Aware Location Recommendation
2016 IEEE 16th International Conference on Data Mining (ICDM)(2016)
摘要
Although weighted tensor factorization tailored to implicit feedback has shown its superior performance in temporal-aware location recommendation, it suffers from three critical challenges. First, it doesn't distinguish the confidence of negative preference for time-dependent unvisited locations from that for fully unvisited ones. Second, discontinuity arises from time discretization, and thus an infinitely large margin may exist between different bins of time. Third, geographical constraints of neighbor locations are not taken into account. To address these challenges, we propose a regularized content-aware tensor factorization (RCTF) algorithm, which exploits three strategies to address the corresponding challenges. First, it introduces a novel interaction regularization; second, it represents each bin of time by a derived feature vector from eigen decomposition of a time- bin similarity matrix, to capture the proximity of neighbor bins of time; third, it encodes geographical information of locations by discrete spatial distributions, so that spatial proximity constraints can be satisfied by simply feeding them into location content. The proposed algorithm is then evaluated for time- aware location recommendation on two large scale location-based social network datasets. The experimental results show the superiority of the proposed algorithm to several competing time- aware recommendation baselines, and verify the significant benefit of three strategies in the proposed algorithm.
更多查看译文
关键词
regularized content-aware tensor factorization,temporal-aware location recommendation,weighted tensor factorization,interaction regularization,feature vector,eigen decomposition,time-bin similarity matrix,spatial proximity constraints,time-aware location recommendation,large scale location-based social network datasets,RCTF
AI 理解论文
溯源树
样例
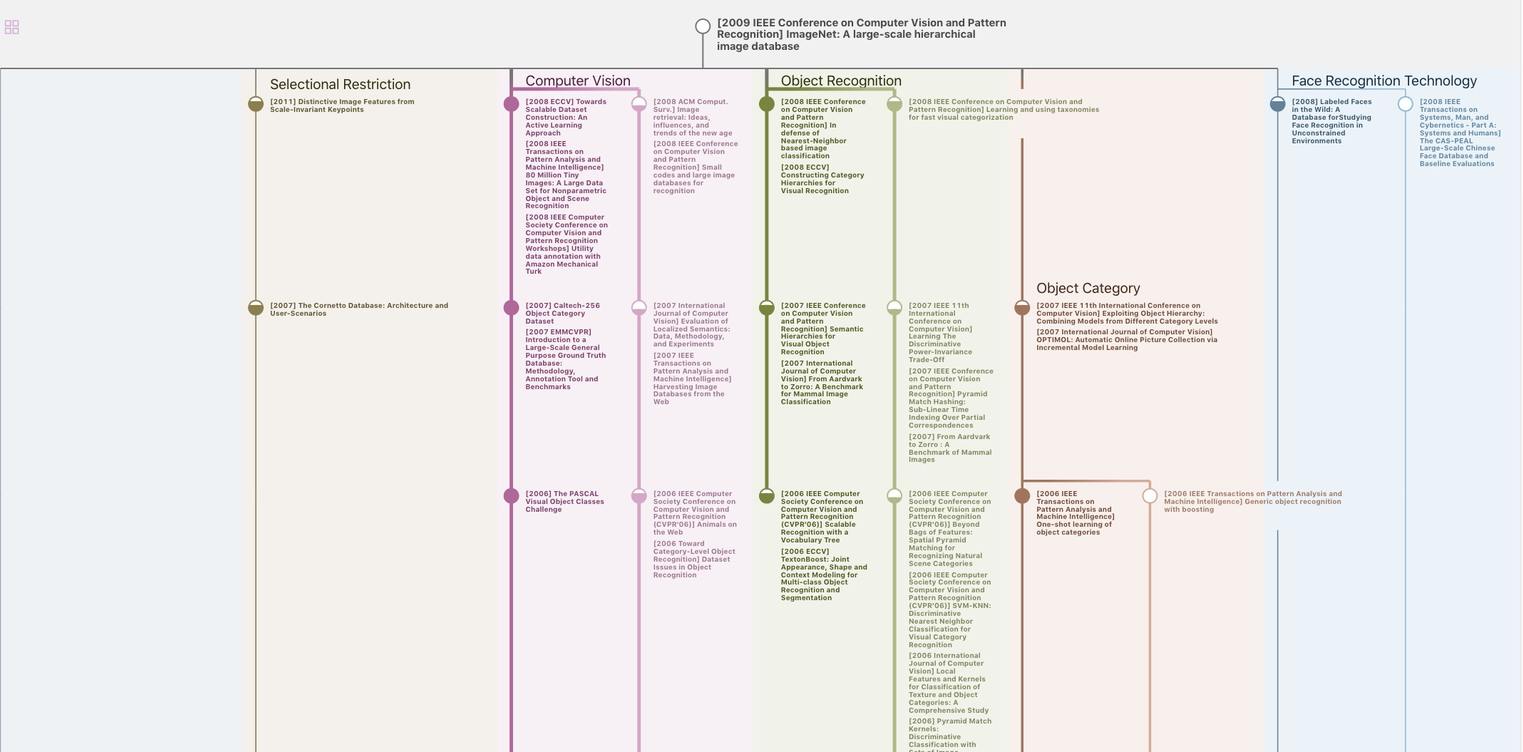
生成溯源树,研究论文发展脉络
Chat Paper
正在生成论文摘要