Improving condition severity classification with an efficient active learning based framework
Journal of Biomedical Informatics(2016)
摘要
(1) Inducing the initial classification model from a small initial training set of conditions randomly selected from the CAESAR dataset. (2) Evaluating the classification model using the test set (containing new unseen conditions) to measure its initial performance. (3) Introducing the pool of unlabeled conditions to the sampling methods. During each trial, a defined number of the most informative conditions are selected according to the AL method’s preferences (or randomly selected by the baseline Random method), and their labels are revealed by the single gold standard labeler used in the original CAESAR system (in a real system the selected conditions will be labeled by an expert, but in our dataset all of the conditions are already labeled). (4) Adding the acquired conditions to the training set and removing them from the pool. (5) Inducing an updated classification model using the updated training set. (6) This process (stages 2–6) iterates until the entire pool is acquired.
更多查看译文
关键词
Active learning,Electronic Health Records,Phenotyping,Condition,Severity
AI 理解论文
溯源树
样例
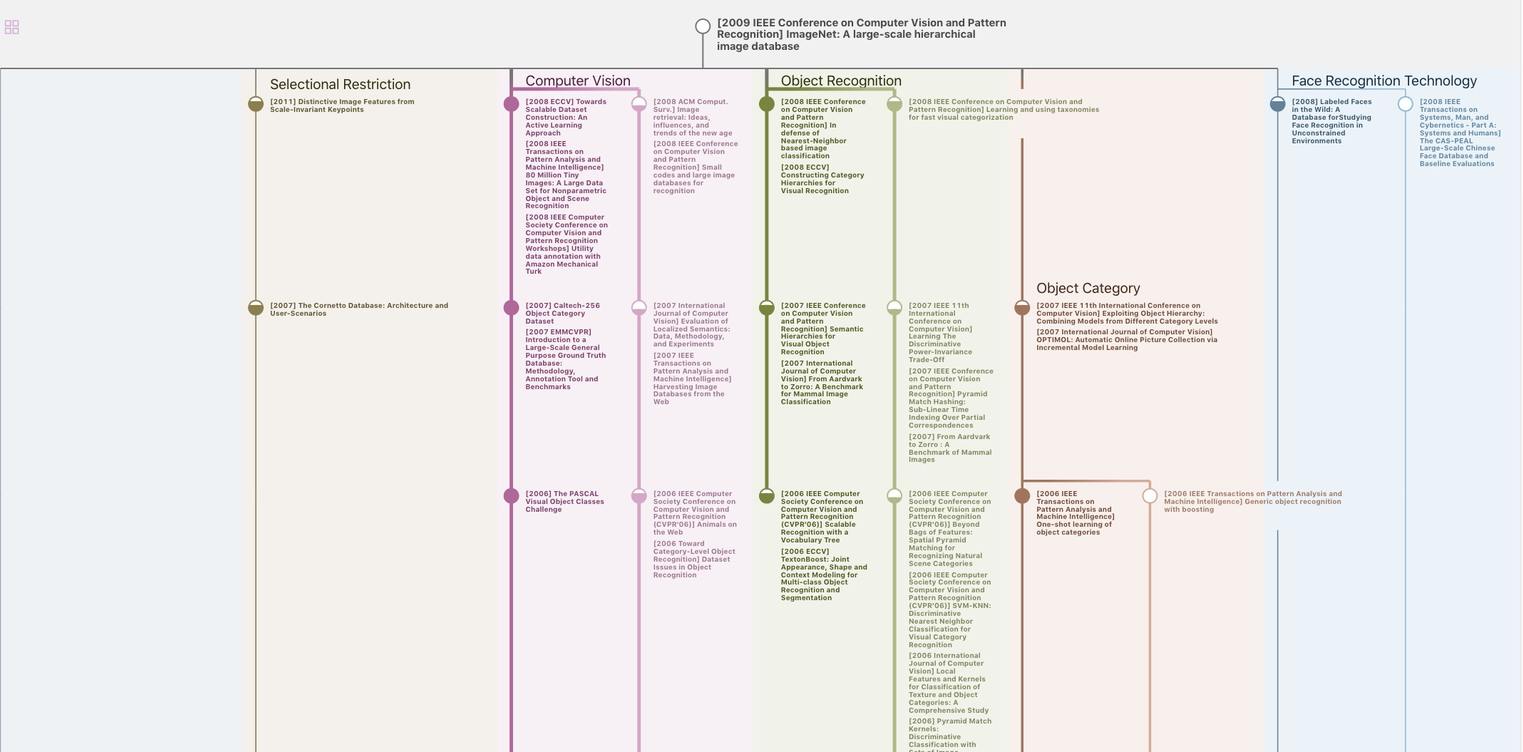
生成溯源树,研究论文发展脉络
Chat Paper
正在生成论文摘要