Towards lazy data association in SLAM
Springer Tracts in Advanced Robotics(2005)
摘要
We present a lazy data association algorithm for the simultaneous localization and mapping (SLAM) problem. Our approach uses a tree-structured Bayesian representation of map posteriors that makes it possible to revise data association decisions arbitrarily far into the past. We describe a criterion for detecting and repairing poor data association decisions. This technique makes it possible to acquire maps of large-scale environments with many loops, with a minimum of computational overhead for the management of multiple data association hypotheses. A empirical comparison with the popular FastSLAM algorithm shows the advantage of lazy over proactive data association.
更多查看译文
关键词
simultaneous localization and mapping,tree structure
AI 理解论文
溯源树
样例
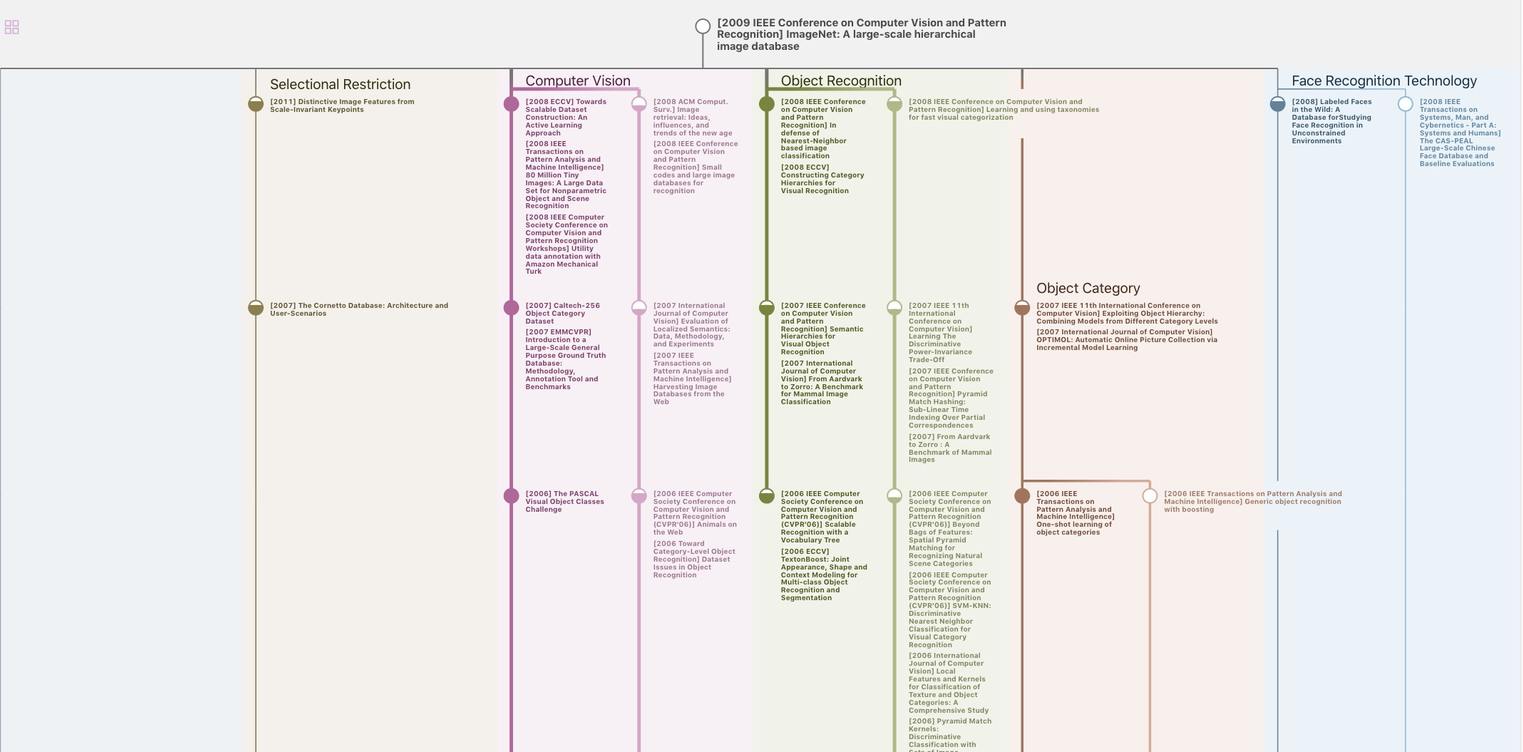
生成溯源树,研究论文发展脉络
Chat Paper
正在生成论文摘要