基本信息
浏览量:504
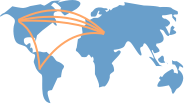
个人简介
His recent research focuses on the interplay between optimization theory and various areas of algorithm design, such as computational geometry, machine learning, signal processing, and algorithmic game theory.
研究兴趣
论文共 212 篇作者统计合作学者相似作者
按年份排序按引用量排序主题筛选期刊级别筛选合作者筛选合作机构筛选
时间
引用量
主题
期刊级别
合作者
合作机构
arxiv(2024)
引用0浏览0引用
0
0
COMPUTATIONAL GEOMETRY-THEORY AND APPLICATIONS (2024): 102077
ICASSP 2024 - 2024 IEEE International Conference on Acoustics, Speech and Signal Processing (ICASSP)pp.7195-7199, (2024)
Mathematical Programmingpp.1-24, (2024)
arxiv(2024)
引用0浏览0引用
0
0
IEEE Transactions on Signal Processingno. 99 (2024): 1-14
arxiv(2024)
引用0浏览0引用
0
0
加载更多
作者统计
合作学者
合作机构
D-Core
- 合作者
- 学生
- 导师
数据免责声明
页面数据均来自互联网公开来源、合作出版商和通过AI技术自动分析结果,我们不对页面数据的有效性、准确性、正确性、可靠性、完整性和及时性做出任何承诺和保证。若有疑问,可以通过电子邮件方式联系我们:report@aminer.cn