An Efficient Alternating Riemannian/Projected Gradient Descent Ascent Algorithm for Fair Principal Component Analysis
ICASSP 2024 - 2024 IEEE International Conference on Acoustics, Speech and Signal Processing (ICASSP)(2024)
摘要
Fair principal component analysis (FPCA), a ubiquitous dimensionality reduction technique in signal processing and machine learning, aims to find a low-dimensional representation for a high-dimensional dataset in view of fairness. The FPCA problem involves optimizing a non-convex and non-smooth function over the Stiefel manifold. The state-of-the-art methods for solving the problem are subgradient methods and semidefinite relaxation-based methods. However, these two types of methods have their obvious limitations and thus are only suitable for efficiently solving the FPCA problem in special scenarios. This paper aims at developing efficient algorithms for solving the FPCA problem in general, especially large-scale, settings. In this paper, we first transform FPCA into a smooth non-convex linear minimax optimization problem over the Stiefel manifold. To solve the above general problem, we propose an efficient alternating Riemannian/projected gradient descent ascent (ARPGDA) algorithm, which performs a Riemannian gradient descent step and an ordinary projected gradient ascent step at each iteration. We prove that ARPGDA can find an ε-stationary point of the above problem within ${\mathcal{O}}\left( {{\varepsilon ^{ - 3}}} \right)$ iterations. Simulation results show that, compared with the state-of-the-art methods, our proposed ARPGDA algorithm can achieve a better performance in terms of solution quality and speed for solving the FPCA problems.
更多查看译文
关键词
FPCA,iteration complexity,minimax problem,Riemannian optimization
AI 理解论文
溯源树
样例
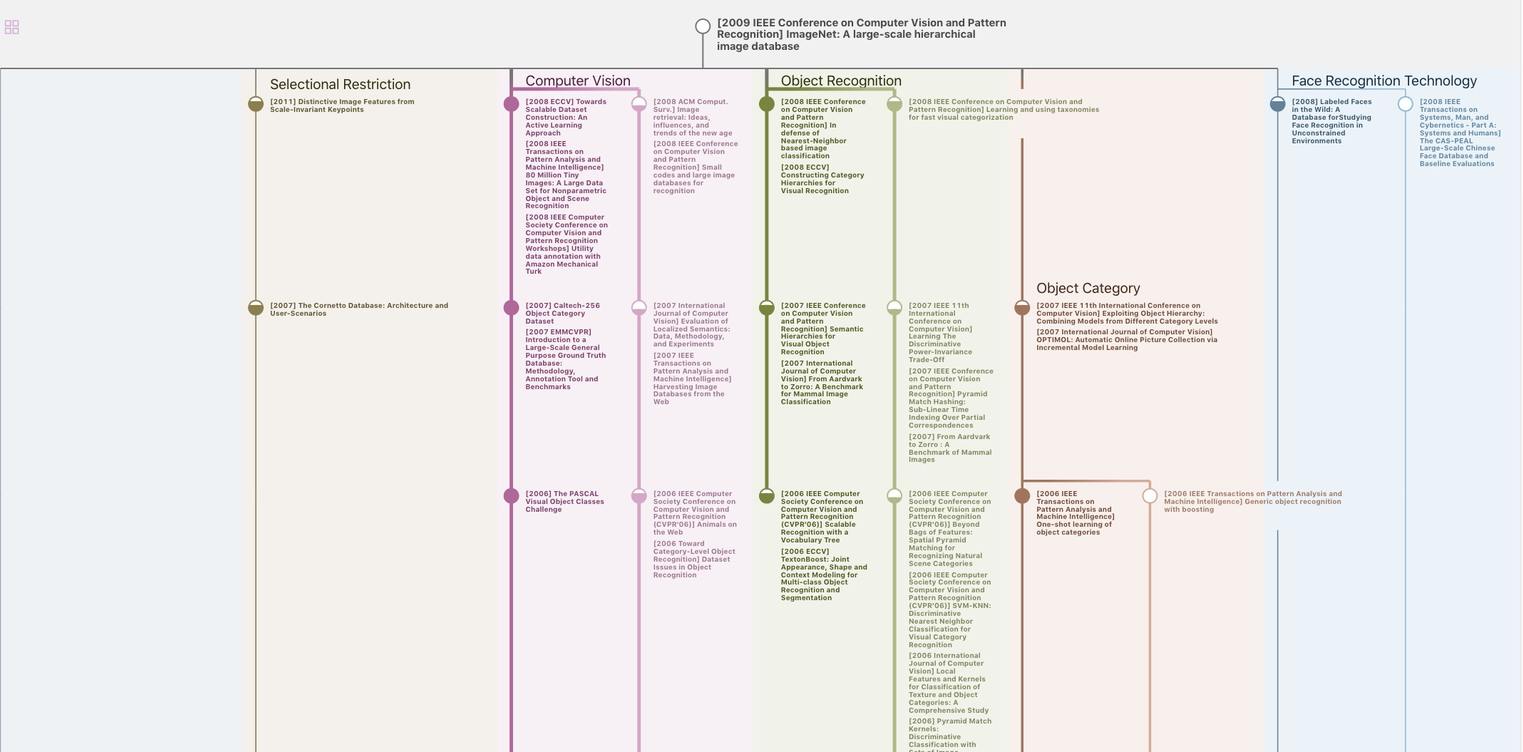
生成溯源树,研究论文发展脉络
Chat Paper
正在生成论文摘要