Large-Scale Continuous-Time Crude Oil Scheduling: A Variable-Length Evolutionary Optimization Approach
IEEE TRANSACTIONS ON AUTOMATION SCIENCE AND ENGINEERING(2024)
摘要
Evolutionary algorithms (EAs) have significantly contributed to addressing large-scale crude oil scheduling problems (COSPs) that exceed the capabilities of mathematical programming. However, current research using EAs to resolve COSPs is limited to discrete-time models that assume uniform operation durations. While this simplification transforms COSPs into fixed-length optimization problems suitable for conventional EAs, it fails to adequately tackle the challenge posed by variable operation durations in COSPs. This limitation results in a compromised solution quality for EAs. To bridge the gap between large-scale COSPs and real-world refineries, this paper introduces the formulation of a continuous-time model for a large-scale COSP, accounting for varying operating durations and practical requirements. In an effort to enhance the effectiveness of EAs in optimizing COSPs represented in continuous time, we propose a variable-length constrained evolutionary algorithm (VLCEA). The variable-length encoding in VLCEA, tailored to the specific problem, facilitates the generation of high-quality solutions with variable lengths. This feature addresses the challenge of determining solution length in advance based on a priori knowledge using conventional methods. Additionally, VLCEA incorporates a sorting strategy and a niching-based selection to expedite the search for promising regions, aiming to achieve better solutions in less time. Experimental results on practical cases not only verify that the continuous-time model produces higher quality solutions compared to the discrete-time model but also demonstrate that VLCEA significantly outperforms five state-of-the-art large-scale EAs with fixed length when applied to the continuous-time model. Note to Practitioners-Crude oil scheduling is one of the most challenging problems in the refinery scheduling process. With the increasing size of scheduling tasks, traditional methods have become inefficient and inaccurate. To address this, evolutionary algorithms (EAs) have emerged as powerful tools for solving large-scale crude oil scheduling problems (COSPs). However, existing EA-based approaches often rely on a fixed number of decisions, resulting in suboptimal solutions when confronted with insufficient prior knowledge. To overcome these obstacles, we have developed a comprehensive continuous-time model specifically designed to address COSPs in real-world refineries. Additionally, we propose a variable-length constrained evolutionary algorithm (VLCEA) as an effective approach for resolving these complex scheduling problems. Computational results demonstrate that the VLCEA achieves superior performance compared to three state-of-the-art EAs in practical large-scale cases. Consequently, the proposed VLCEA exhibits significant potential for solving real-world refinery scheduling problems and can be extended to tackle other scheduling problems with an unfixed number of decisions.
更多查看译文
关键词
Job shop scheduling,Oils,Optimization,Encoding,Task analysis,Dynamic scheduling,Evolutionary computation,Large-scale crude oil scheduling,continuous-time model,variable-length representation,evolutionary algorithm
AI 理解论文
溯源树
样例
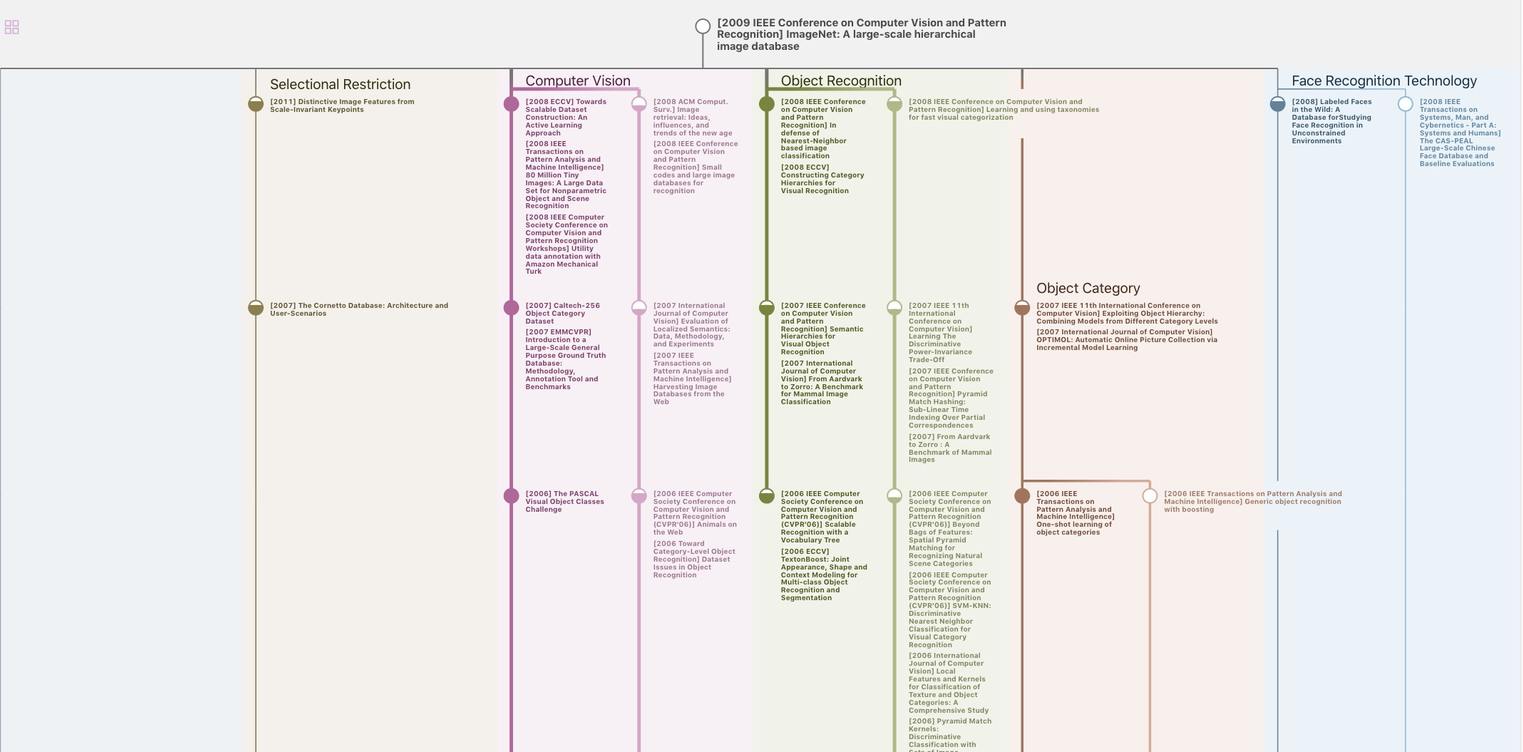
生成溯源树,研究论文发展脉络
Chat Paper
正在生成论文摘要