DeepKP: A Robust and Accurate Framework for Weld Seam Keypoint Extraction in Welding Robots
IEEE TRANSACTIONS ON INSTRUMENTATION AND MEASUREMENT(2024)
摘要
To meet the demand for seam tracking of welding robots, a deep learning-based framework called DeepKP is proposed in this article, which aims to precisely extract weld seam keypoints (WSKPs) under multiple arc light interference. DeepKP comprises a keypoint extraction model named WeldExt and a denoising model named WeldDenoise. WeldExt is proposed to identify weld seam types, obtain prior box region (PBR), and then extract keypoints in the region. WeldExt addresses the problem that most recent extraction models cannot directly obtain the keypoints. WeldDenoise is proposed for denoising weld seam images affected by multiple arc light interference, which overcomes the limitation that most recent denoising models must use paired datasets for training. Experiment results show that the average locating error of WSKP in WeldExt is 1.75 pixels and the average seam tracking error of DeepKp is 0.336 mm. Therefore, DeepKP performs excellently in extracting WSKPs under challenging arc light conditions and improves the quality of seam tracking.
更多查看译文
关键词
Linear laser vision sensor,robot welding,seam tracking,weld seam denoising,weld seam keypoint (WSKP) extraction
AI 理解论文
溯源树
样例
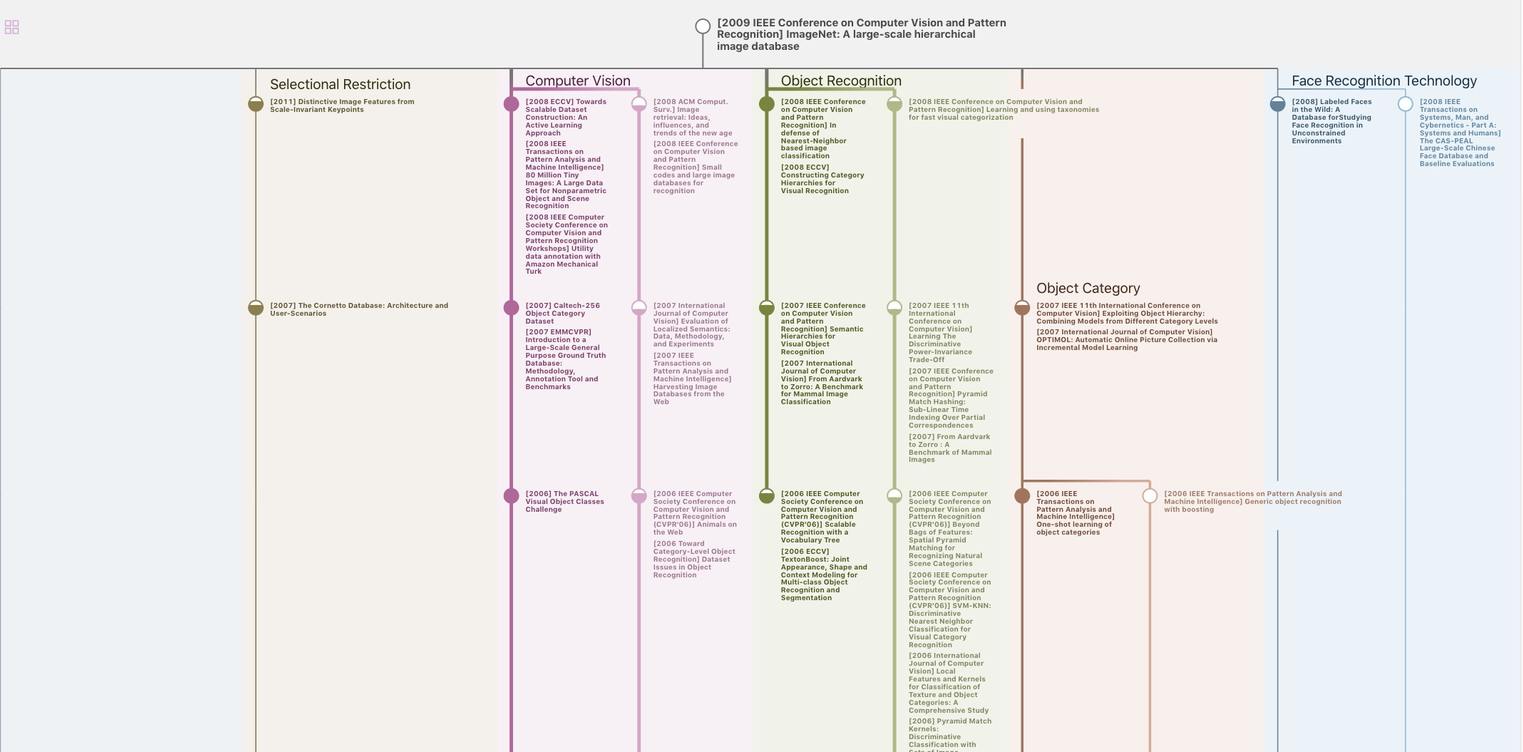
生成溯源树,研究论文发展脉络
Chat Paper
正在生成论文摘要