Reinforcement Learning-based Recommender Systems with Large Language Models for State Reward and Action Modeling
arxiv(2024)
摘要
Reinforcement Learning (RL)-based recommender systems have demonstrated
promising performance in meeting user expectations by learning to make accurate
next-item recommendations from historical user-item interactions. However,
existing offline RL-based sequential recommendation methods face the challenge
of obtaining effective user feedback from the environment. Effectively modeling
the user state and shaping an appropriate reward for recommendation remains a
challenge. In this paper, we leverage language understanding capabilities and
adapt large language models (LLMs) as an environment (LE) to enhance RL-based
recommenders. The LE is learned from a subset of user-item interaction data,
thus reducing the need for large training data, and can synthesise user
feedback for offline data by: (i) acting as a state model that produces high
quality states that enrich the user representation, and (ii) functioning as a
reward model to accurately capture nuanced user preferences on actions.
Moreover, the LE allows to generate positive actions that augment the limited
offline training data. We propose a LE Augmentation (LEA) method to further
improve recommendation performance by optimising jointly the supervised
component and the RL policy, using the augmented actions and historical user
signals. We use LEA, the state and reward models in conjunction with
state-of-the-art RL recommenders and report experimental results on two
publicly available datasets.
更多查看译文
AI 理解论文
溯源树
样例
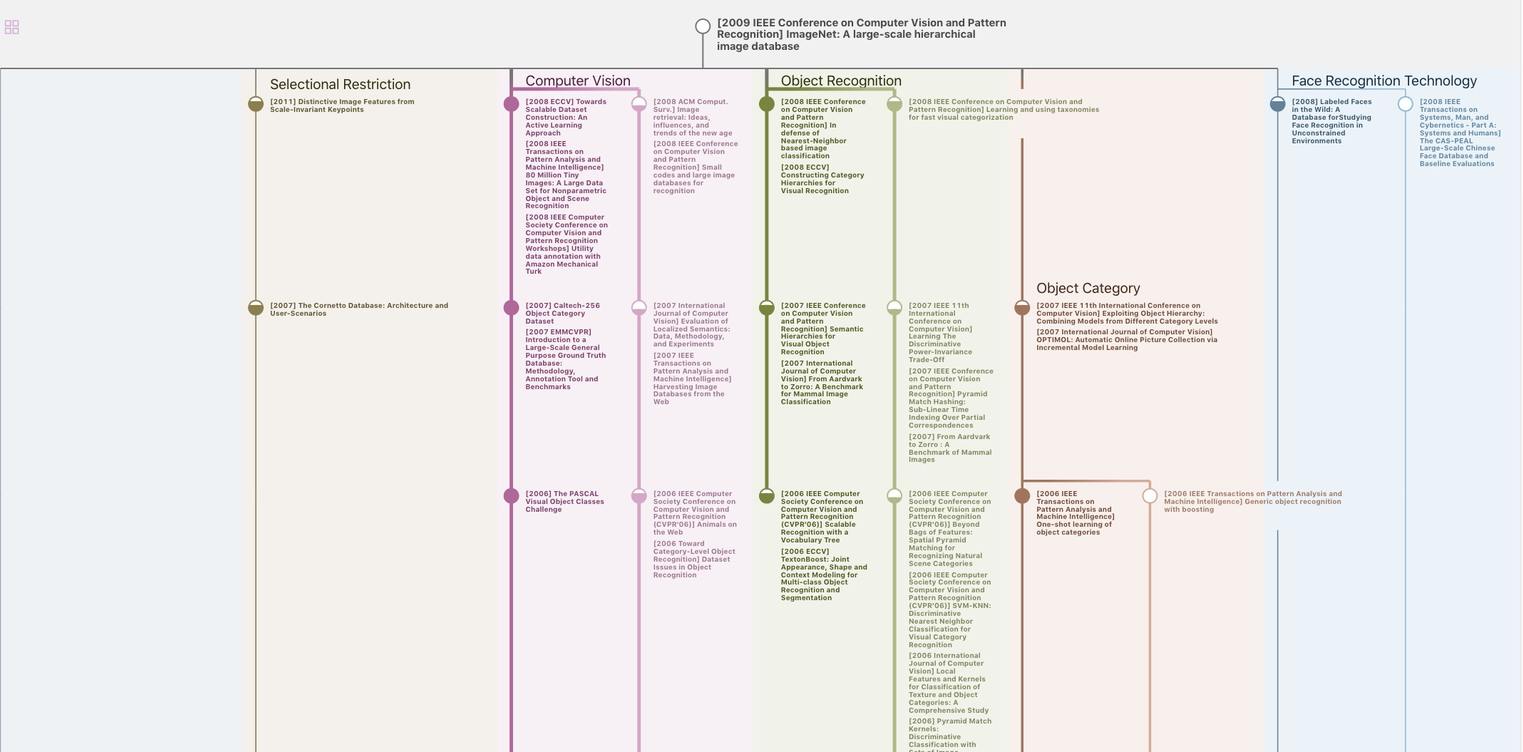
生成溯源树,研究论文发展脉络
Chat Paper
正在生成论文摘要