Ghost Sentence: A Tool for Everyday Users to Copyright Data from Large Language Models
arxiv(2024)
摘要
Web user data plays a central role in the ecosystem of pre-trained large
language models (LLMs) and their fine-tuned variants. Billions of data are
crawled from the web and fed to LLMs. How can everyday web
users confirm if LLMs misuse their data without permission? In this work, we
suggest that users repeatedly insert personal passphrases into their documents,
enabling LLMs to memorize them. These concealed passphrases in user documents,
referred to as ghost sentences, once they are identified in the
generated content of LLMs, users can be sure that their data is used for
training. To explore the effectiveness and usage of this copyrighting tool, we
define the user training data identification task with ghost
sentences. Multiple datasets from various sources at different scales are
created and tested with LLMs of different sizes. For evaluation, we introduce a
last k words verification manner along with two metrics: document and user
identification accuracy. In the specific case of instruction tuning of a 3B
LLaMA model, 11 out of 16 users with ghost sentences identify their data within
the generation content. These 16 users contribute 383 examples to ∼1.8M
training documents. For continuing pre-training of a 1.1B TinyLlama model, 61
out of 64 users with ghost sentences identify their data within the LLM output.
These 64 users contribute 1156 examples to ∼10M training documents.
更多查看译文
AI 理解论文
溯源树
样例
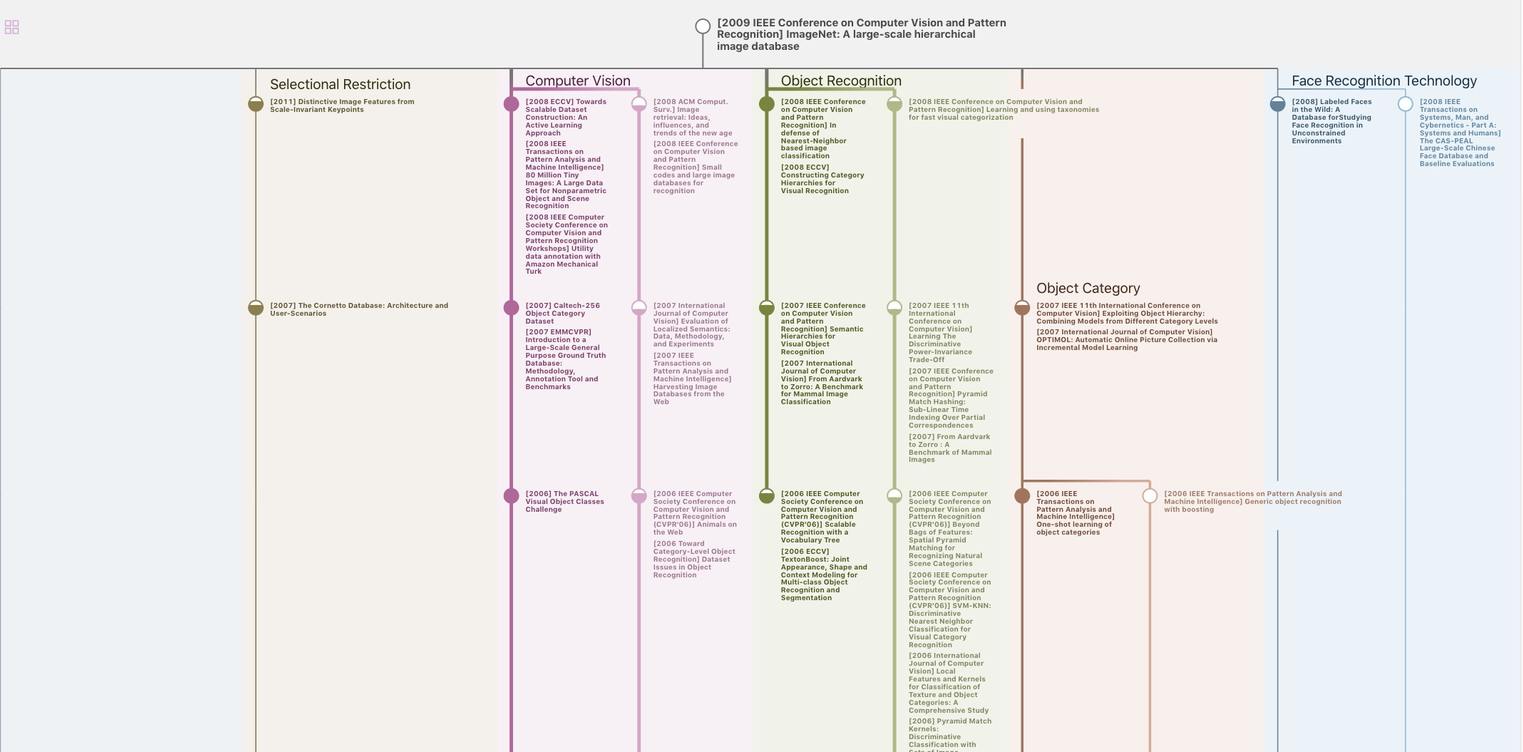
生成溯源树,研究论文发展脉络
Chat Paper
正在生成论文摘要