Abstract 1025: An AI based CT scan classifier for predicting extranodal extension and nodal metastasis of HPV-associated oropharyngeal carcinoma
Cancer Research(2024)
摘要
Abstract Background: Pathologic extranodal extension (ENE) and nodal metastasis (NM) are of prognostic significance in human papillomavirus (HPV)-associated oropharyngeal squamous cell carcinoma (OPSCC). The pretreatment identifications of these two factors through computed tomography (CT) imaging are of clinical importance since they could guide treatment de-escalation protocols and select candidates suitable for surgery. In an effort to enhance pretreatment decision making, we developed and validated an AI model (specifically a multi-layer perceptron (MLP)-based deep learning model) to predict radiologic NM and pathologic ENE in HPV-associated OPSCC patients. Methods: Radiographic CT scans for 290 OPSCC patients treated with definitive chemoradiation were acquired from The Cancer Imaging Archive (TCIA) and divided into training (D1) and validation (D2) cohorts for NM prediction. Radiologic NM for D1 and D2 were reviewed by two radiologists. Only nodes with concordant NM judgments from both radiologists were included for analysis. An additional 46 OPSCC patients treated with surgery at Emory Winship Cancer Institute were collected to form the test cohorts (D3) for ENE prediction. The largest metastatic or normal nodes were annotated for D1 and D2 while all visible nodes with pathology confirmation of ENE from D3 were annotated. We present a novel deep-learning model utilizing U-shaped multi-layer perceptron mixer (MLP-Mixer) to capture both global and local feature representations by spatially aggregating image patch information through a procedure called token-mixing. The model was trained for 20 epochs with learning rate of0.0001 and batch size of 64. The diagnostic performance of the AI model was evaluated on D2 and D3 using accuracy and area under the ROC curve (AUROC). Results: There were 121 NM negative and 130 NM positive nodes in D1, 14 NM negative and 25 NM positive nodes in D2 and 46 ENE negative and 20 ENE positive nodes in D3. On D2 for NM prediction, the AI model achieved overall accuracy of 0.77 and AUROC of 0.78. On D3 for ENE prediction, the AI model achieved overall accuracy of 0.71, AUROC of 0.69, positive predictive value of 0.52 and negative predictive value of 0.86. Conclusions: The new AI–based deep learning model was able to identify HPV-associated OPSCC NM and ENE with satisfactory diagnostic performance and shows promise to assist clinicians in treatment decision-making. Additional, multi-site prospective validation of the approach is warranted. Citation Format: Bolin Song, Kailin Yang, Shaoyan Pan, Liping Li, Qiyun Liu, Jonathan Lee, Sarah Stock, Nabil F. Saba, Mehmet Asim Bilen, Mihir R. Patel, Anant Madabhushi. An AI based CT scan classifier for predicting extranodal extension and nodal metastasis of HPV-associated oropharyngeal carcinoma [abstract]. In: Proceedings of the American Association for Cancer Research Annual Meeting 2024; Part 1 (Regular Abstracts); 2024 Apr 5-10; San Diego, CA. Philadelphia (PA): AACR; Cancer Res 2024;84(6_Suppl):Abstract nr 1025.
更多查看译文
AI 理解论文
溯源树
样例
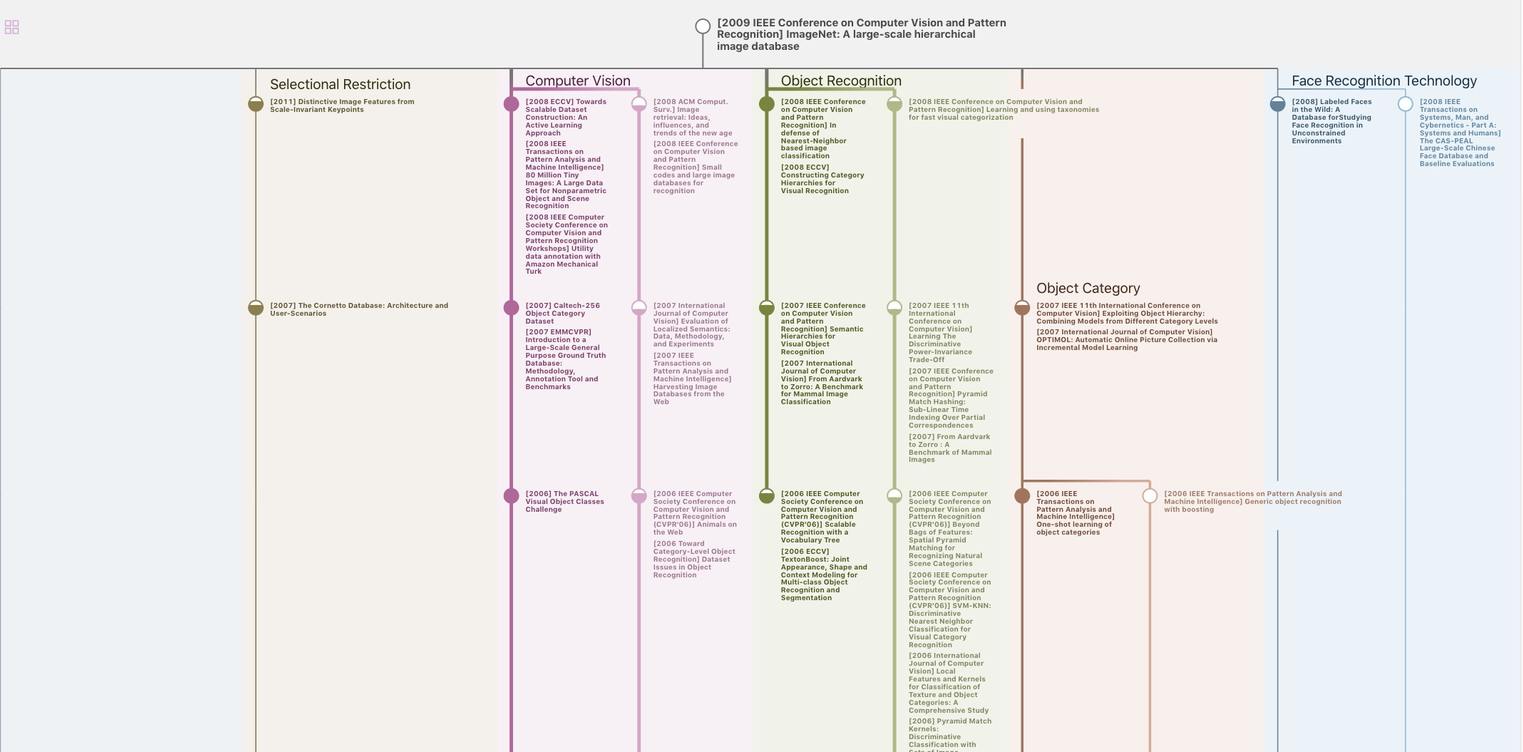
生成溯源树,研究论文发展脉络
Chat Paper
正在生成论文摘要