Network Convergence Indicator for Efficient Robot Grasping Pose Detection under Limited Computation Resource
IEEE Transactions on Instrumentation and Measurement(2024)
摘要
Industrial edge computing devices typically have limited computational performance. Current high-precision grasping pose detection models are often over-parameterized, resulting in computational inefficiency and making it difficult to be deployed on industrial robots. Existing efficient network design methods rely on constructing, training, and validating a large number of new networks to obtain the final target network, which also require significant computing resources. To address this issue, the Network Convergence Indicator (NCI) is proposed to realize the prediction of network accuracy before training. NCI can evaluate convergence based on the evasion of flat regions and saddle points theory. It is used to control the width and depth of the network model and optimize its innner layers. Based on these principles, NCIGrasp is proposed, which is a high-precision lightweight grasping network achieving outstanding performance on the Cornell and Jacquard datasets with only 10% of the parameters of previous advanced models. NCIGrasp has a small size of 0.69 MB and fast inference speed on server (~8ms), making it suitable for edge computing. Experimental results show that NCIGrasp has real-time grasping pose detection ability (~21ms) for unknown objects on an ordinary laptop with limited computation capability.
更多查看译文
关键词
Grasping Detection,Manipulation Relationship Detection,Object Detection
AI 理解论文
溯源树
样例
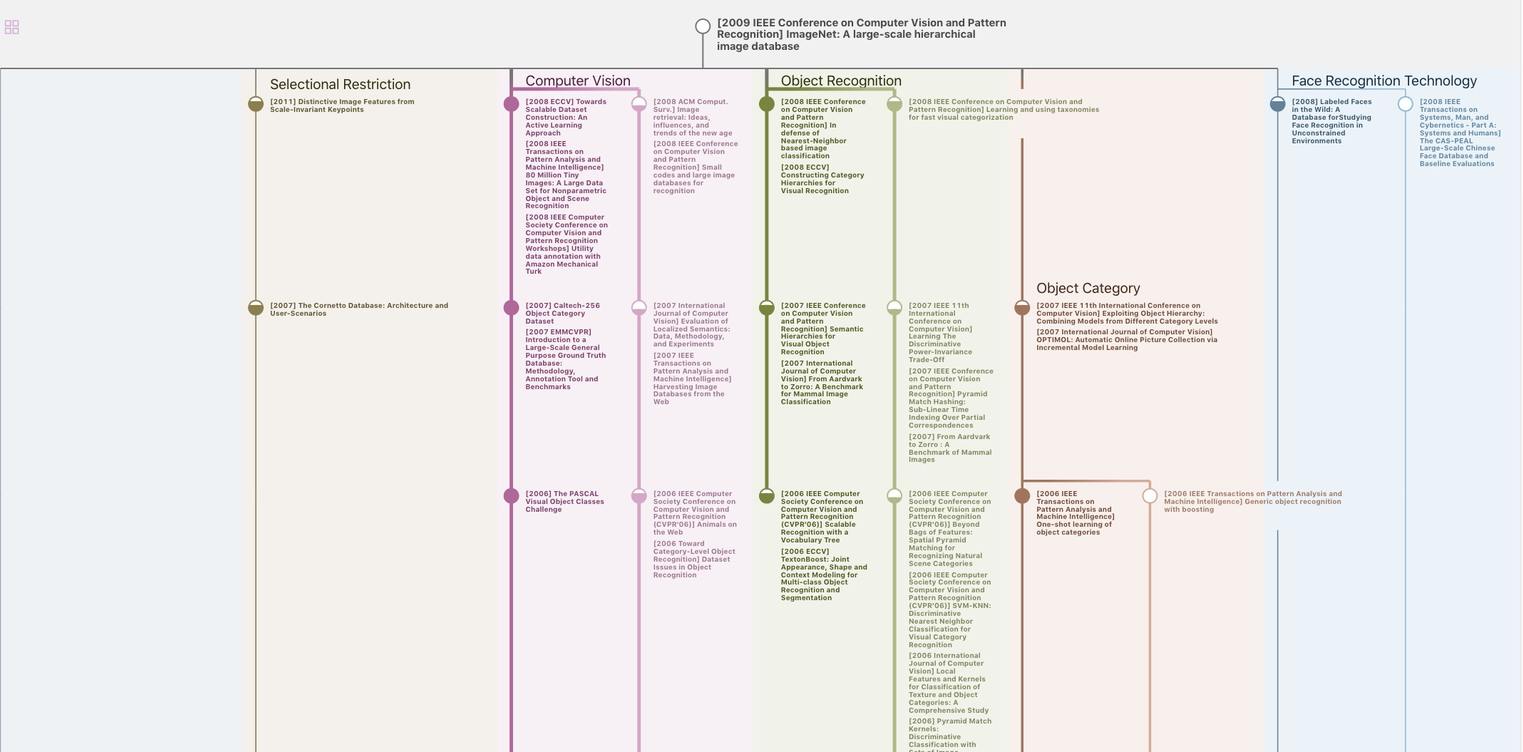
生成溯源树,研究论文发展脉络
Chat Paper
正在生成论文摘要