Pretraining Codomain Attention Neural Operators for Solving Multiphysics PDEs
arxiv(2024)
摘要
Existing neural operator architectures face challenges when solving
multiphysics problems with coupled partial differential equations (PDEs), due
to complex geometries, interactions between physical variables, and the lack of
large amounts of high-resolution training data. To address these issues, we
propose Codomain Attention Neural Operator (CoDA-NO), which tokenizes functions
along the codomain or channel space, enabling self-supervised learning or
pretraining of multiple PDE systems. Specifically, we extend positional
encoding, self-attention, and normalization layers to the function space.
CoDA-NO can learn representations of different PDE systems with a single model.
We evaluate CoDA-NO's potential as a backbone for learning multiphysics PDEs
over multiple systems by considering few-shot learning settings. On complex
downstream tasks with limited data, such as fluid flow simulations and
fluid-structure interactions, we found CoDA-NO to outperform existing methods
on the few-shot learning task by over 36%. The code is available at
https://github.com/ashiq24/CoDA-NO.
更多查看译文
AI 理解论文
溯源树
样例
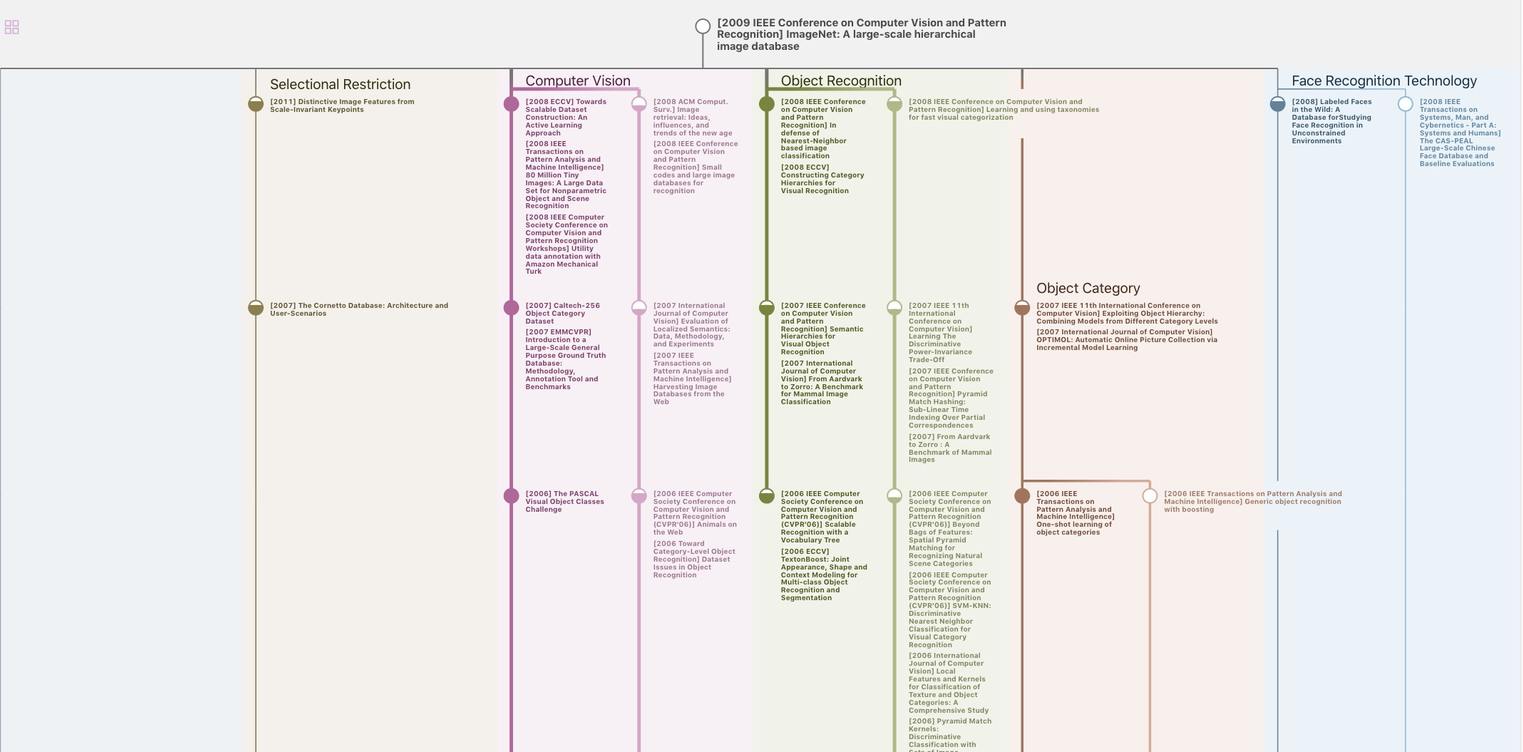
生成溯源树,研究论文发展脉络
Chat Paper
正在生成论文摘要