View Crafting For Instance-Level Representation from Scene Images
ICASSP 2024 - 2024 IEEE International Conference on Acoustics, Speech and Signal Processing (ICASSP)(2024)
摘要
Existing image-level self-supervised learning (SSL) methods pre-trained on natural scene data can have difficulty in adating to dense prediction tasks. However, scene images contain multiple varied instances. We devise two techniques to craft high-quality scene and instance views for instance-level SSL. Firstly, we leverage the prior from the image-level pre-trained model to discover the salient instances and the correspondence between cross-image instance pairs. Then, we augment multiple instances to compose the scene views, where both scene similarity and variance are promoted. Meanwhile, we encourage the scene and inner instances to align in feature space to model the scene-instance correlation. Experiments show its SOTA performance in dense prediction tasks.
更多查看译文
关键词
Self-supervised learning,semantic-aware proposal,scene-instance correlation
AI 理解论文
溯源树
样例
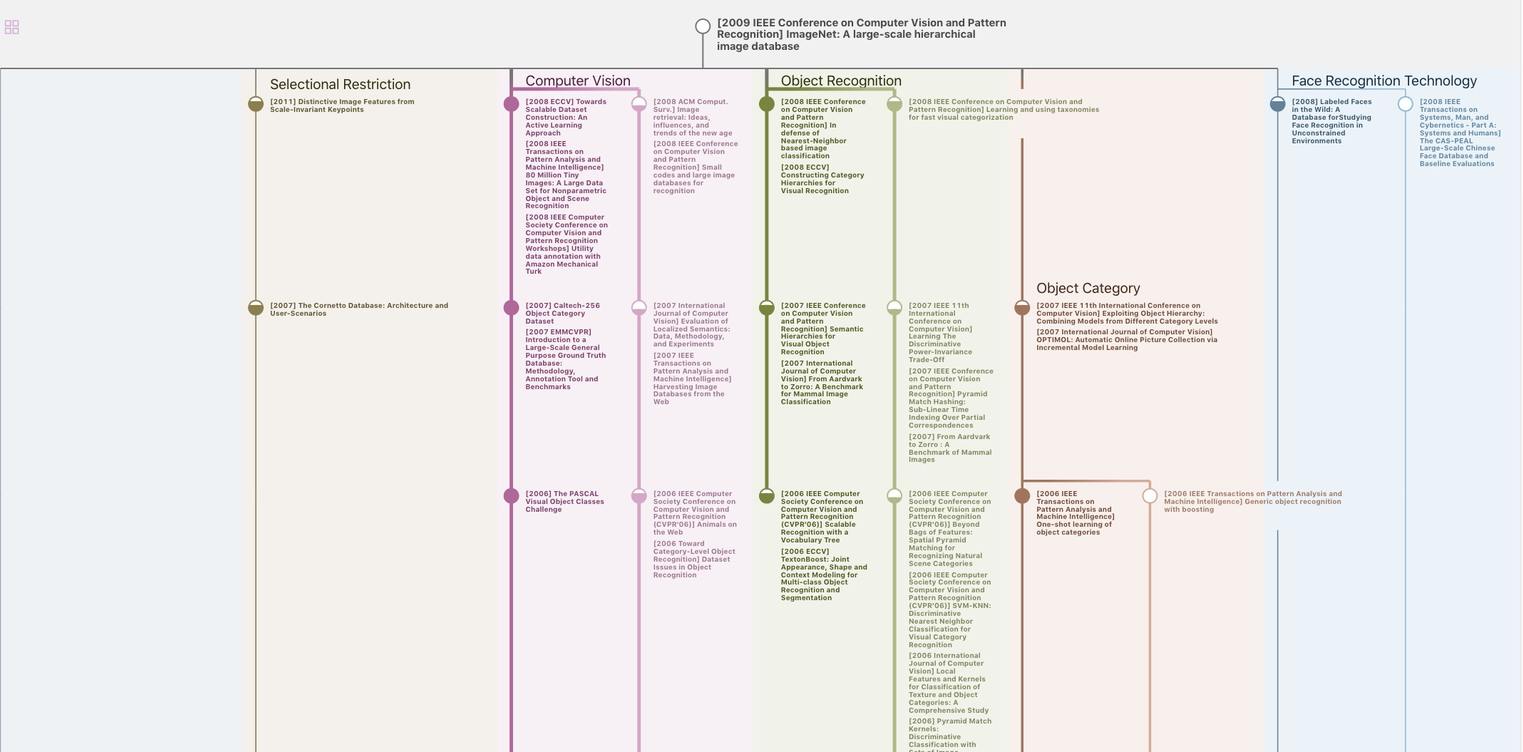
生成溯源树,研究论文发展脉络
Chat Paper
正在生成论文摘要