Boosting Dynamic Decentralized Federated Learning by Diversifying Model Sources
IEEE Transactions on Services Computing(2024)
摘要
Recently, federated learning (FL) has received intensive research because of its ability in preserving data privacy for scattered clients to collaboratively train machine learning models. Decentralized federated learning (DFL) is upgraded from FL which allows clients to aggregate model parameters with their neighbours directly. DFL is particularly feasible for dynamic systems, in which the neighbour set of each client is dynamic. However, due to the restrictions of client trajectories and communication distances, it is hard for individual clients to sufficiently exchange models with others, resulting in poor model accuracy. To address this challenge, we propose the DFL-DMS (DFL with Diversified Model Sources) algorithm to diversify sources for model aggregation, and improve model utility. Specifically, models exchanged between DFL-DMS clients are jointly determined by their staleness scores and the bandwidth constraint. An asynchronous learning mode is adopted so that DFL-DMS clients can temporarily store and relay fresh models collected from different client sources to accelerate the propagation of rare models. A state vector is maintained to track the contribution weight of each source to its model aggregation, and an entropy based metric (EBM) is optimized by clients in a distributed manner. Finally, the superiority of DFL-DMS is evaluated by extensive experiments (with MNIST and CIFAR-10 datasets) which demonstrate that DFL-DMS can accelerate the convergence of DFL and improve the model accuracy significantly compared with the state-of-the-art baselines.
更多查看译文
关键词
Decentralized Federated Learning,Privacy Protection,Vehicular Networks,KL Divergence
AI 理解论文
溯源树
样例
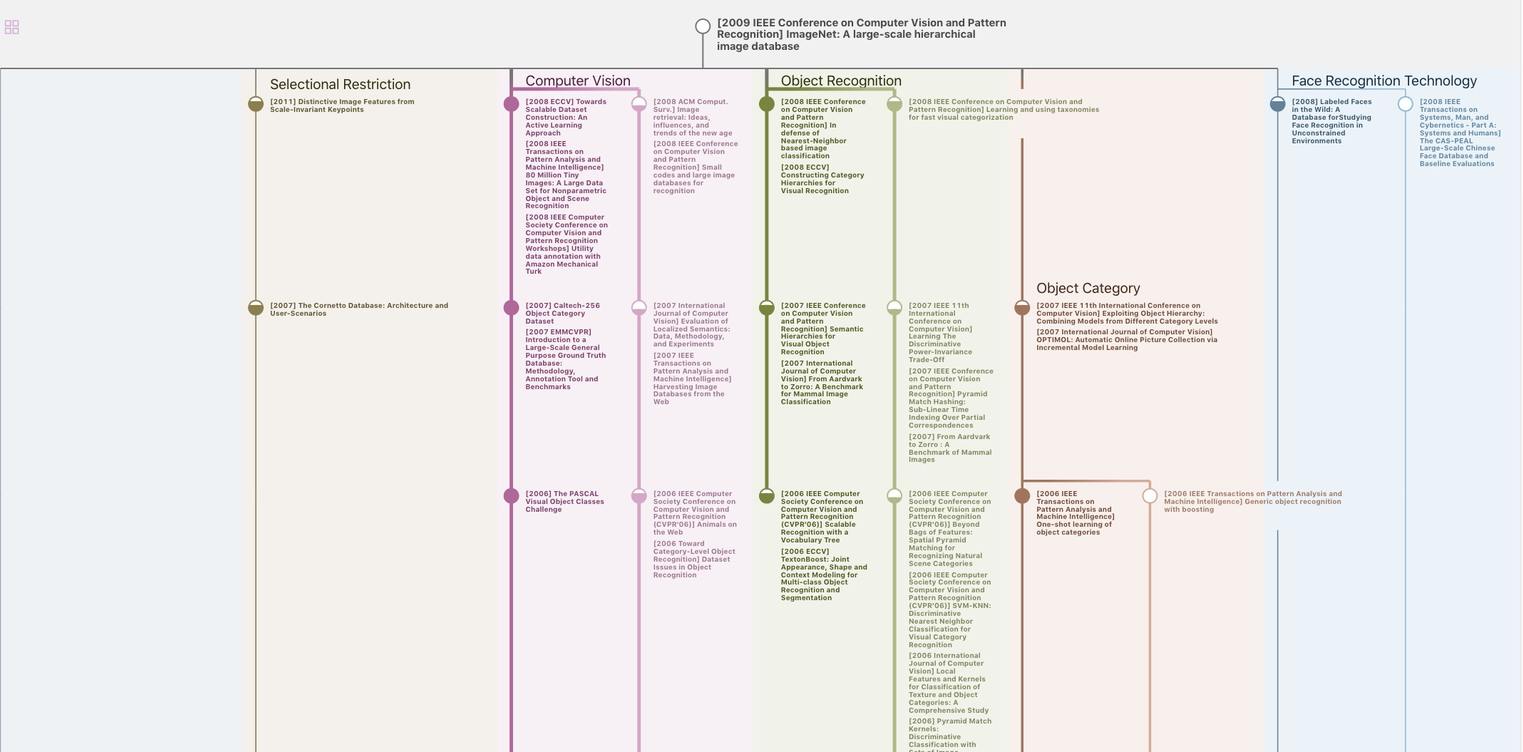
生成溯源树,研究论文发展脉络
Chat Paper
正在生成论文摘要