Bidirectional feature learning network for RGB-D salient object detection
PATTERN RECOGNITION(2024)
摘要
RGB-D salient object detection aims to perform the pixel-wise localization of salient objects from both RGB and depth images, whose challenge mainly comes from how to learn complementary features from each modality. Existing works often use increasingly large models for performance enhancement, which need large memory and time consumption in practice. In this paper, we propose a simple yet effective Bidirectional Feature Learning Network (BFLNet) for RGB-D salient object detection under limited memory and time conditions. To achieve accurate performance with lightweight backbone networks, an effective Bidirectional Feature Fusion (BFF) module is designed to merge features from both RGB and depth streams, in which the crossmodal fusions and cross-scale fusions are jointly conducted to fuse the immediate features in multiple scales and multiple modals. What is more, a simple Dual Consistency Loss (DCL) function is designed to prompt cross -modal fusion by keeping the consistency between cross -modal target predictions. Extensive experiments on four benchmark datasets demonstrate that our method has achieved the state-of-the-art performance with high efficiency in RGB-D salient object detection. Code will be available at https://github.com/nightskynostar/BFLNet.
更多查看译文
关键词
RGB-D salient object detection,Bidirectional feature fusion,Dual consistency loss
AI 理解论文
溯源树
样例
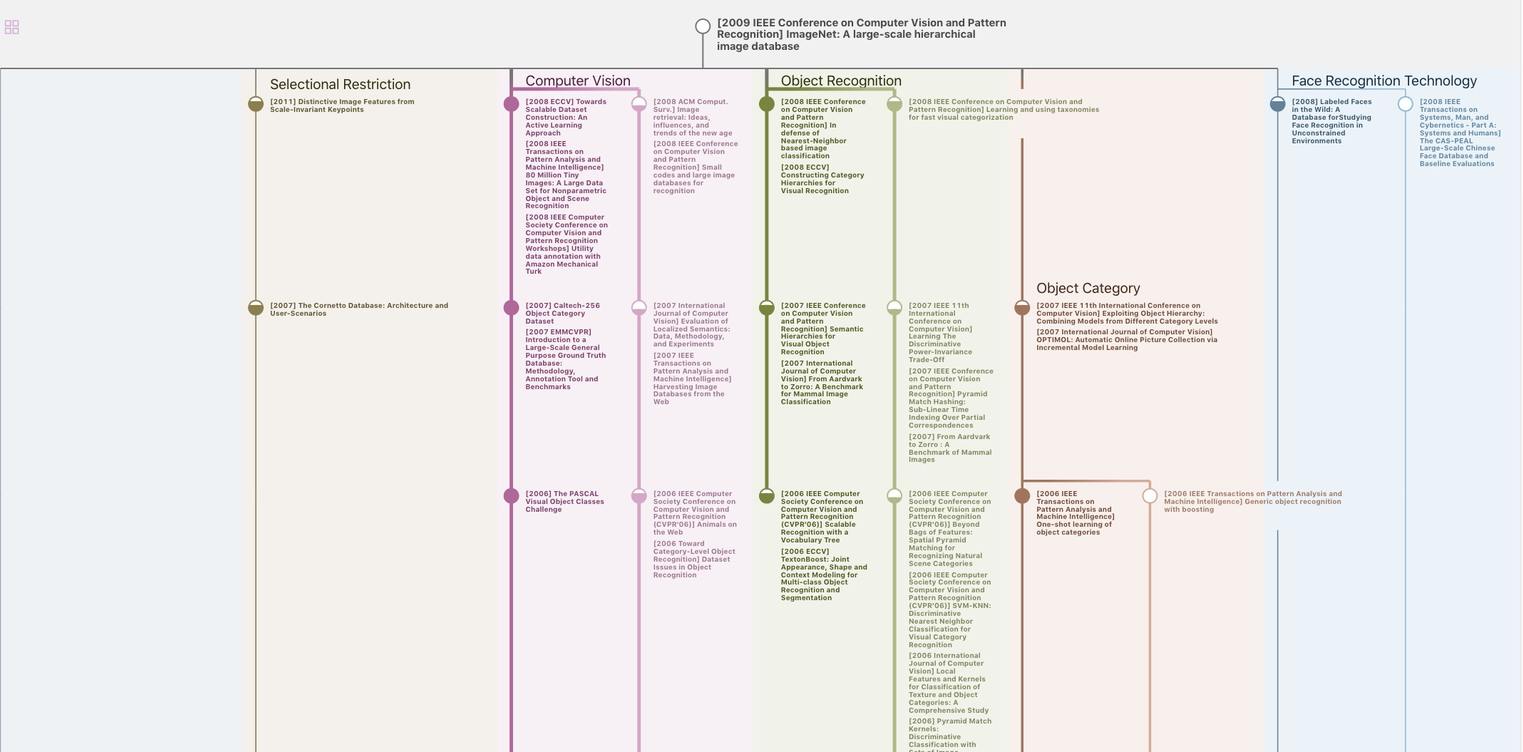
生成溯源树,研究论文发展脉络
Chat Paper
正在生成论文摘要