An attentive Copula-based spatio-temporal graph model for multivariate time-series
APPLIED SOFT COMPUTING(2024)
摘要
Time -series forecasting is widely applied to electricity consumption. However, accurate prediction for tasks is challenging due to intricate spatial dependencies and non-linear temporal dynamics. Existing models have limited capability for considering correlation factors, leading to reduced accuracy. Incorporating geographical information can enhance predictions in multivariate models. Graph neural networks effectively capture variable interdependencies, and including location information between nodes complements these dependencies. Therefore, we propose an attentive spatio-temporal graph neural network framework for accurate time -series forecasting. Our approach incorporates time -series and geographical factors to enhance prediction accuracy. We create a geometrical graph using node locations and a probabilistic graph structure learned from node embedding to capture non-linear temporal dynamics. The attention mechanism facilitates feature crossover, improving spatial -related features. We model representation and correlation information based on joint distributions in the nodes, separating them into edge densities and Copula densities. We link the graph structure and the covariance matrix in the Copula densities. Extensive evaluations of the public electrical consumption dataset demonstrate that our approach outperforms state-of-the-art models, significantly improving accuracy in multi -factor time -series forecasting tasks such as electricity consumption.
更多查看译文
关键词
Electricity consumption,Copula method,Attention mechanism,Spatio-temporal graph,Time-series prediction
AI 理解论文
溯源树
样例
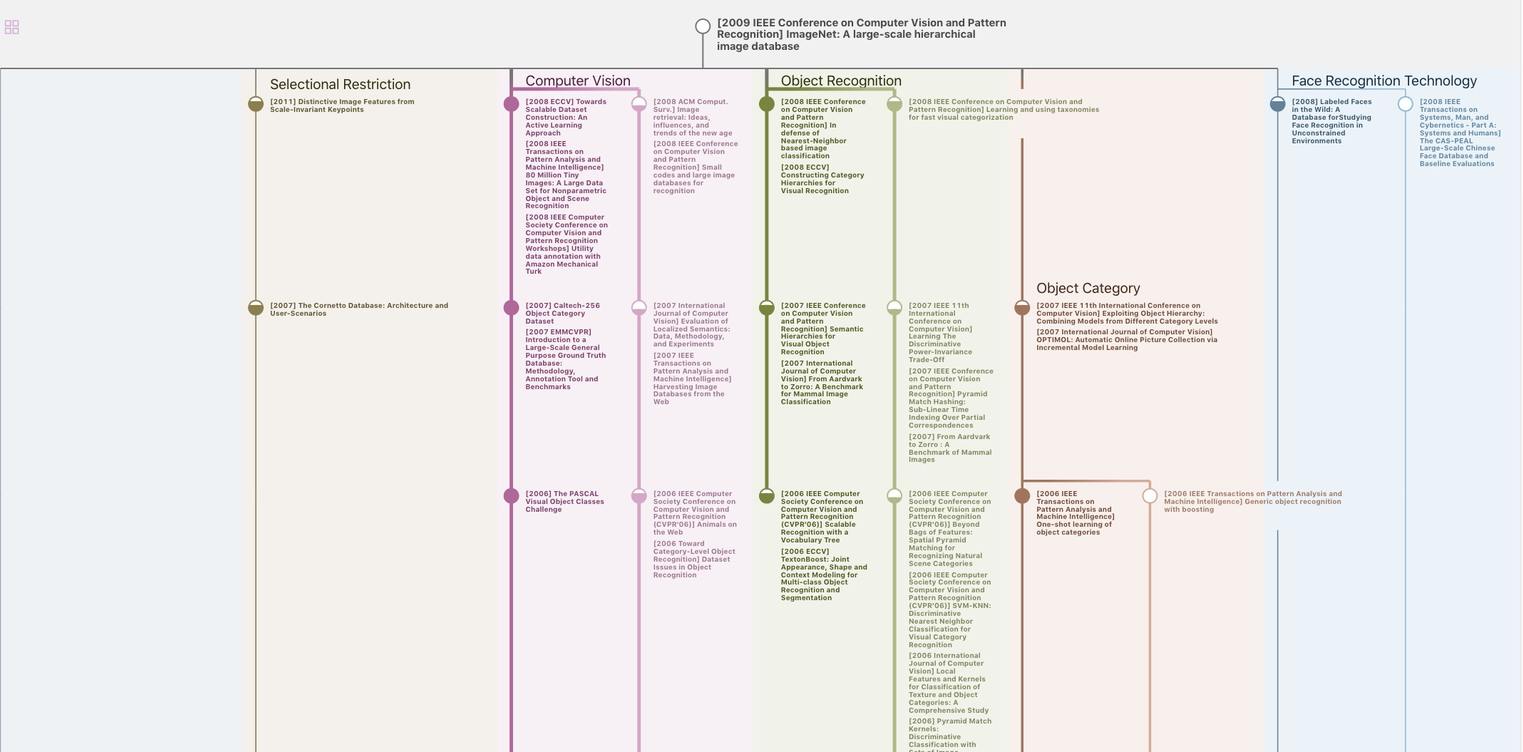
生成溯源树,研究论文发展脉络
Chat Paper
正在生成论文摘要