Data Quality Assessment: Challenges and Opportunities
arxiv(2024)
摘要
Data-oriented applications, their users, and even the law require data of
high quality. Research has broken down the rather vague notion of data quality
into various dimensions, such as accuracy, consistency, and reputation, to name
but a few. To achieve the goal of high data quality, many tools and techniques
exist to clean and otherwise improve data. Yet, systematic research on actually
assessing data quality in all of its dimensions is largely absent, and with it
the ability to gauge the success of any data cleaning effort. It is our vision
to establish a systematic and comprehensive framework for the (numeric)
assessment of data quality for a given dataset and its intended use. Such a
framework must cover the various facets that influence data quality, as well as
the many types of data quality dimensions. In particular, we identify five
facets that serve as a foundation of data quality assessment. For each facet,
we outline the challenges and opportunities that arise when trying to actually
assign quality scores to data and create a data quality profile for it, along
with a wide range of technologies needed for this purpose.
更多查看译文
AI 理解论文
溯源树
样例
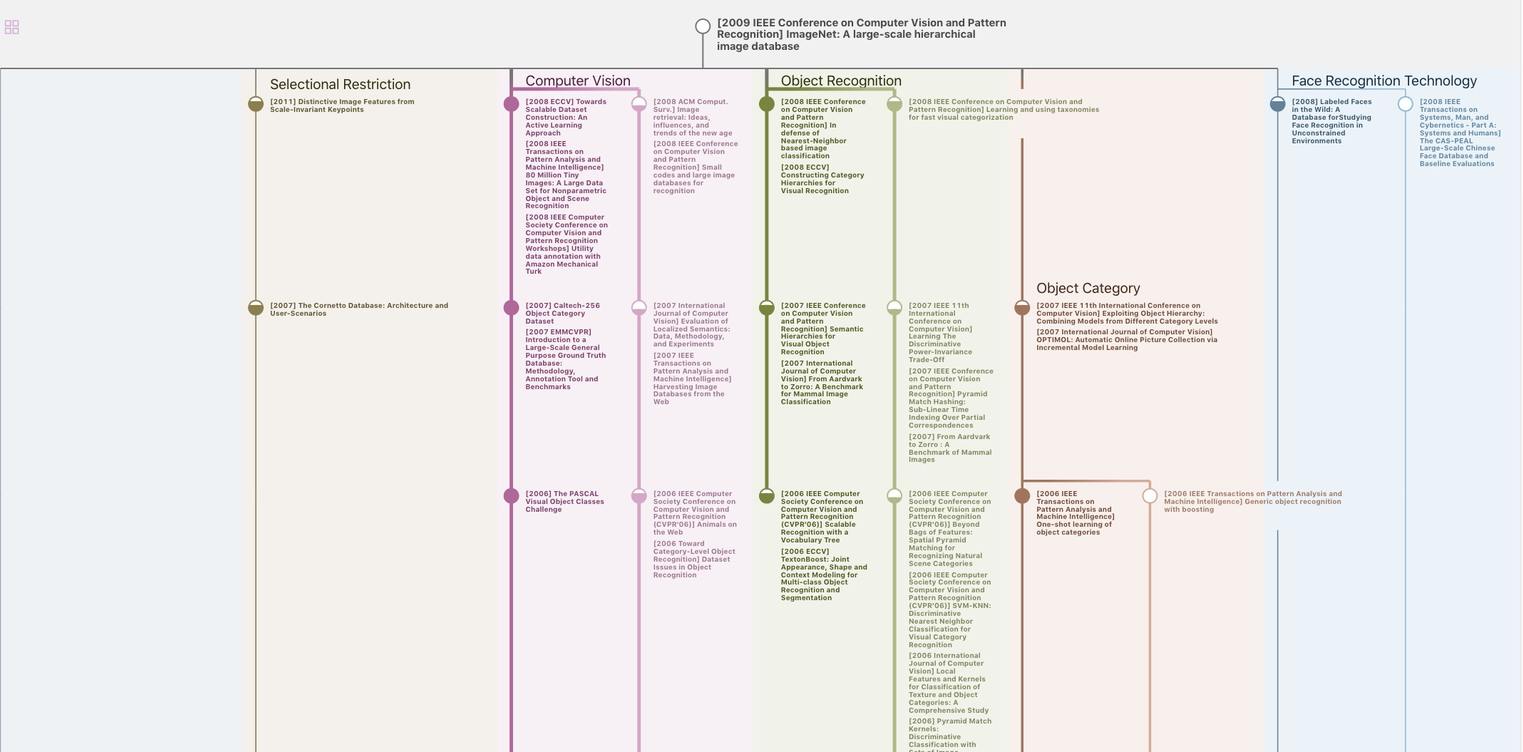
生成溯源树,研究论文发展脉络
Chat Paper
正在生成论文摘要