Deep learning modeling of ribosome profiling reveals regulatory underpinnings of translatome and interprets disease variants
biorxiv(2024)
摘要
Gene expression involves transcription and translation. Despite large datasets and increasingly powerful methods devoted to calculating genetic variants' effects on transcription, discrepancy between mRNA and protein levels hinders the systematic interpretation of the regulatory effects of disease-associated variants. Accurate models of the sequence determinants of translation are needed to close this gap and to interpret disease-associated variants that act on translation. Here, we present Translatomer, a multimodal transformer framework that predicts cell-type-specific translation from mRNA expression and gene sequence. We train Translatomer on 33 tissues and cell lines, and show that the inclusion of sequence substantially improves the prediction of ribosome profiling signal, indicating that Translatomer captures sequence-dependent translational regulatory information. Translatomer achieves accuracies of 0.72 to 0.80 for de novo prediction of cell-type-specific ribosome profiling. We develop an in silico mutagenesis tool to estimate mutational effects on translation and demonstrate that variants associated with translation regulation are evolutionarily constrained, both within the human population and across species. Notably, we identify cell-type-specific translational regulatory mechanisms independent of eQTLs for 3,041 non-coding and synonymous variants associated with complex diseases, including Alzheimer's disease, schizophrenia, and congenital heart disease. Translatomer accurately models the genetic underpinnings of translation, bridging the gap between mRNA and protein levels, and providing valuable mechanistic insights toward mapping "missing regulation" in disease genetics.
### Competing Interest Statement
The authors have declared no competing interest.
更多查看译文
AI 理解论文
溯源树
样例
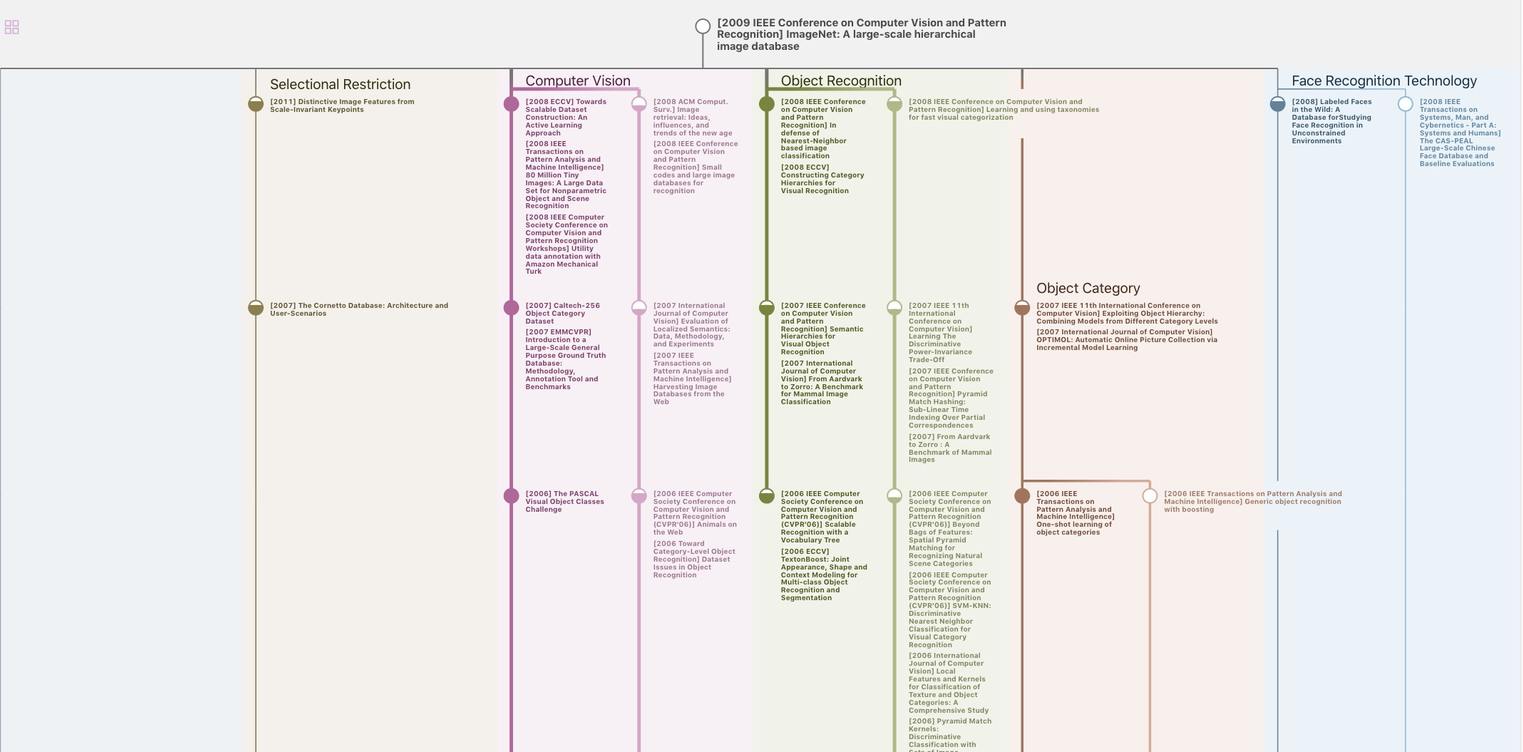
生成溯源树,研究论文发展脉络
Chat Paper
正在生成论文摘要