Multi-Constraint Safe RL with Objective Suppression for Safety-Critical Applications
arxiv(2024)
摘要
Safe reinforcement learning tasks with multiple constraints are a challenging
domain despite being very common in the real world. In safety-critical domains,
properly handling the constraints becomes even more important. To address this
challenge, we first describe the multi-constraint problem with a stronger
Uniformly Constrained MDP (UCMDP) model; we then propose Objective Suppression,
a novel method that adaptively suppresses the task reward maximizing objectives
according to a safety critic, as a solution to the Lagrangian dual of a UCMDP.
We benchmark Objective Suppression in two multi-constraint safety domains,
including an autonomous driving domain where any incorrect behavior can lead to
disastrous consequences. Empirically, we demonstrate that our proposed method,
when combined with existing safe RL algorithms, can match the task reward
achieved by our baselines with significantly fewer constraint violations.
更多查看译文
AI 理解论文
溯源树
样例
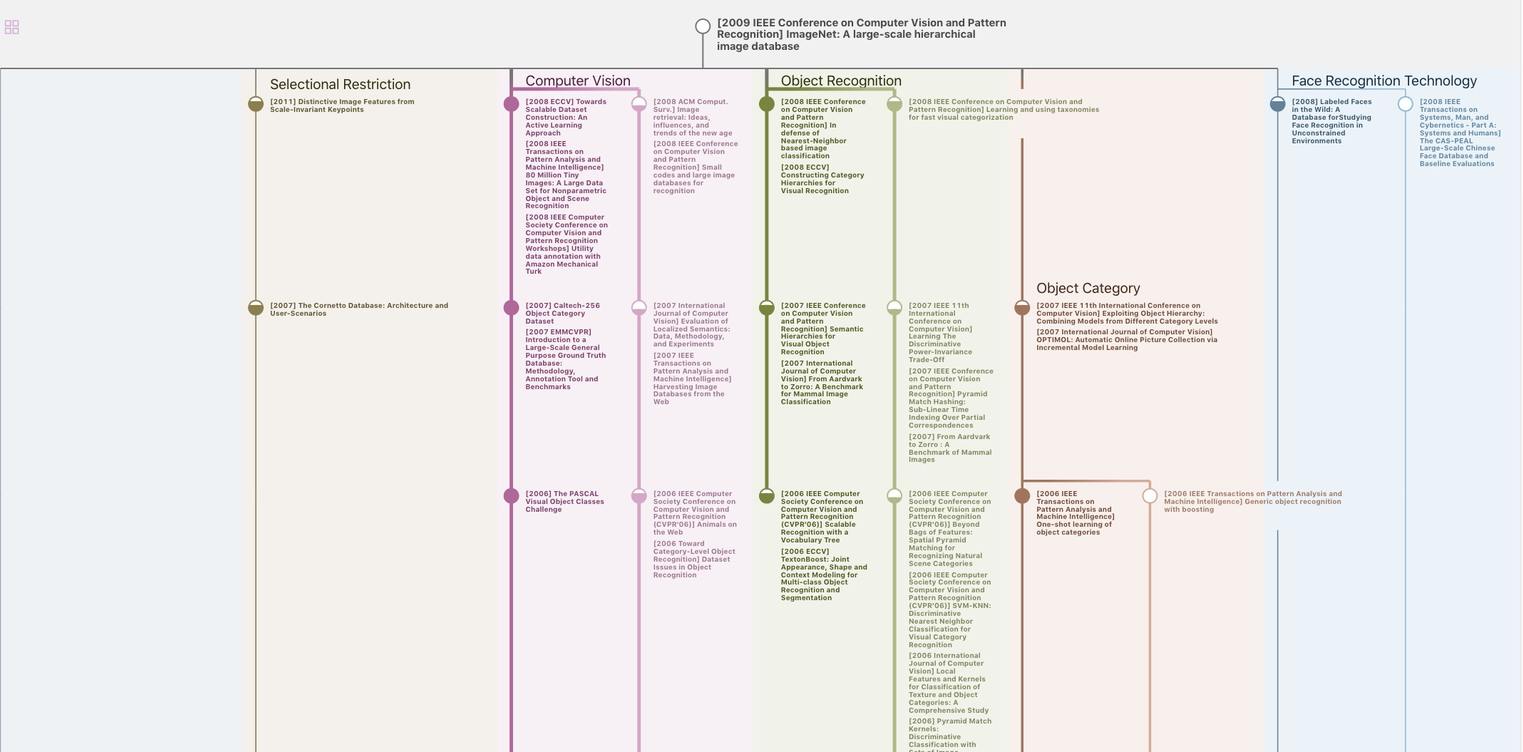
生成溯源树,研究论文发展脉络
Chat Paper
正在生成论文摘要