Motion planning framework based on dual-agent DDPG method for dual-arm robots guided by human joint angle constraints
FRONTIERS IN NEUROROBOTICS(2024)
摘要
Introduction Reinforcement learning has been widely used in robot motion planning. However, for multi-step complex tasks of dual-arm robots, the trajectory planning method based on reinforcement learning still has some problems, such as ample exploration space, long training time, and uncontrollable training process. Based on the dual-agent depth deterministic strategy gradient (DADDPG) algorithm, this study proposes a motion planning framework constrained by the human joint angle, simultaneously realizing the humanization of learning content and learning style. It quickly plans the coordinated trajectory of dual-arm for complex multi-step tasks.Methods The proposed framework mainly includes two parts: one is the modeling of human joint angle constraints. The joint angle is calculated from the human arm motion data measured by the inertial measurement unit (IMU) by establishing a human-robot dual-arm kinematic mapping model. Then, the joint angle range constraints are extracted from multiple groups of demonstration data and expressed as inequalities. Second, the segmented reward function is designed. The human joint angle constraint guides the exploratory learning process of the reinforcement learning method in the form of step reward. Therefore, the exploration space is reduced, the training speed is accelerated, and the learning process is controllable to a certain extent.Results and discussion The effectiveness of the framework was verified in the gym simulation environment of the Baxter robot's reach-grasp-align task. The results show that in this framework, human experience knowledge has a significant impact on the guidance of learning, and this method can more quickly plan the coordinated trajectory of dual-arm for multi-step tasks.
更多查看译文
关键词
trajectory planning,reinforcement learning,dual-agent depth deterministic strategy gradient,human experience constrains guidance,motion parameter mapping
AI 理解论文
溯源树
样例
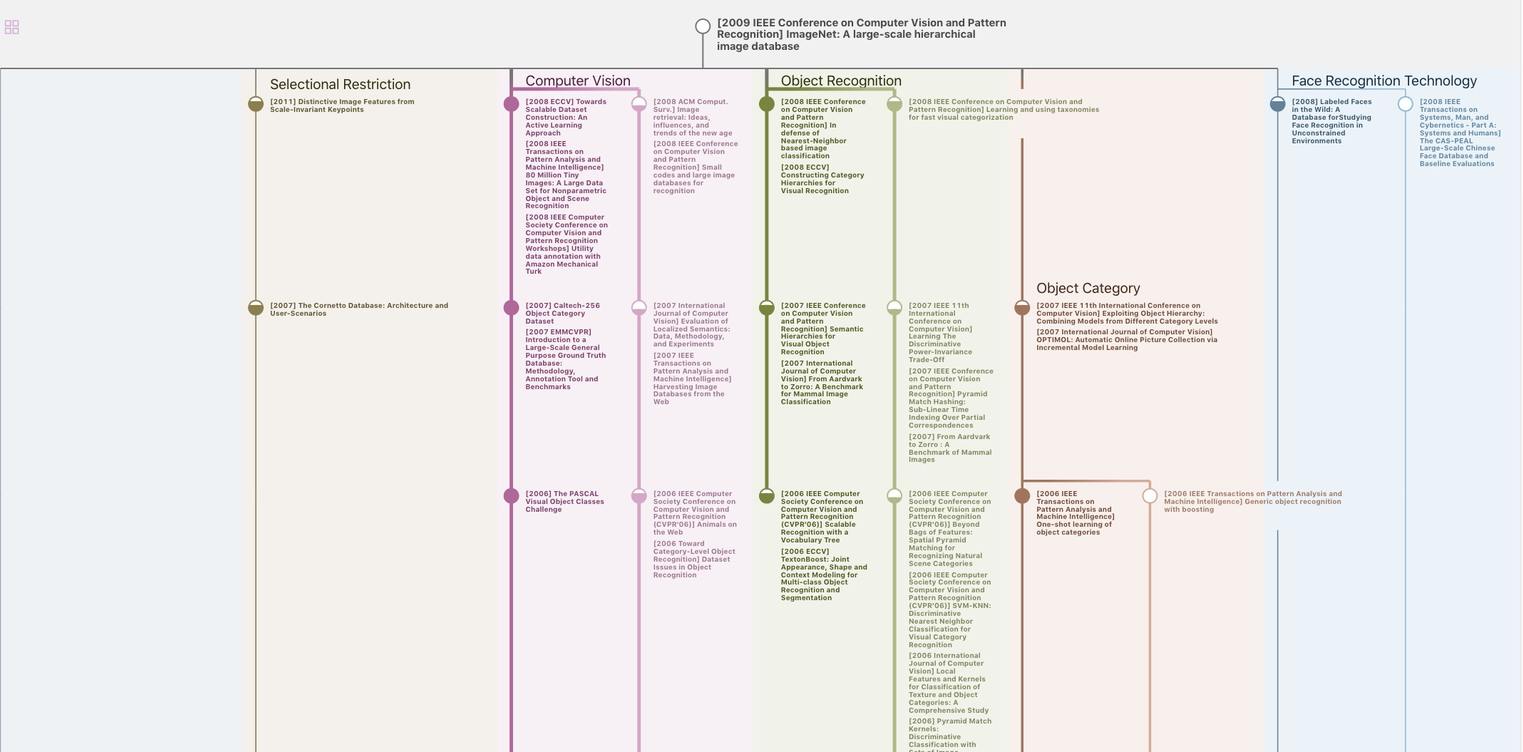
生成溯源树,研究论文发展脉络
Chat Paper
正在生成论文摘要