Transferability of Machine Learning Models for Soil Properties on Lucas Topsoil Spectral Libraries.
Workshop on Hyperspectral Image and Signal Processing(2023)
摘要
Soil spectroscopy has been widely used for soil characterization, due to its high potential for assessing different soil properties. Although machine learning models have been applied with great success, little is known about model performance when applied on different soil databases. This study investigates the prediction of key soil properties such as organic carbon, clay, sand, silt, and pH in H
2
O. Machine learning models are trained using the Partial Least Squares regression and Random Forest learning algorithms to predict the different topsoil physicochemical properties from the laboratory reflectance data in the LUCAS (Land Use/Land Cover Area Frame Survey) 2009 topsoil database. They are subsequently evaluated in the LUCAS 2015 topsoil dabase. Although a performance decline is observed, similar results can be obtained by using targeted additional data in the training sets, while being computationally less demanding.
更多查看译文
关键词
Artificial Intelligence,Soil Spectroscopy,SOC,LUCAS topsoil dataset
AI 理解论文
溯源树
样例
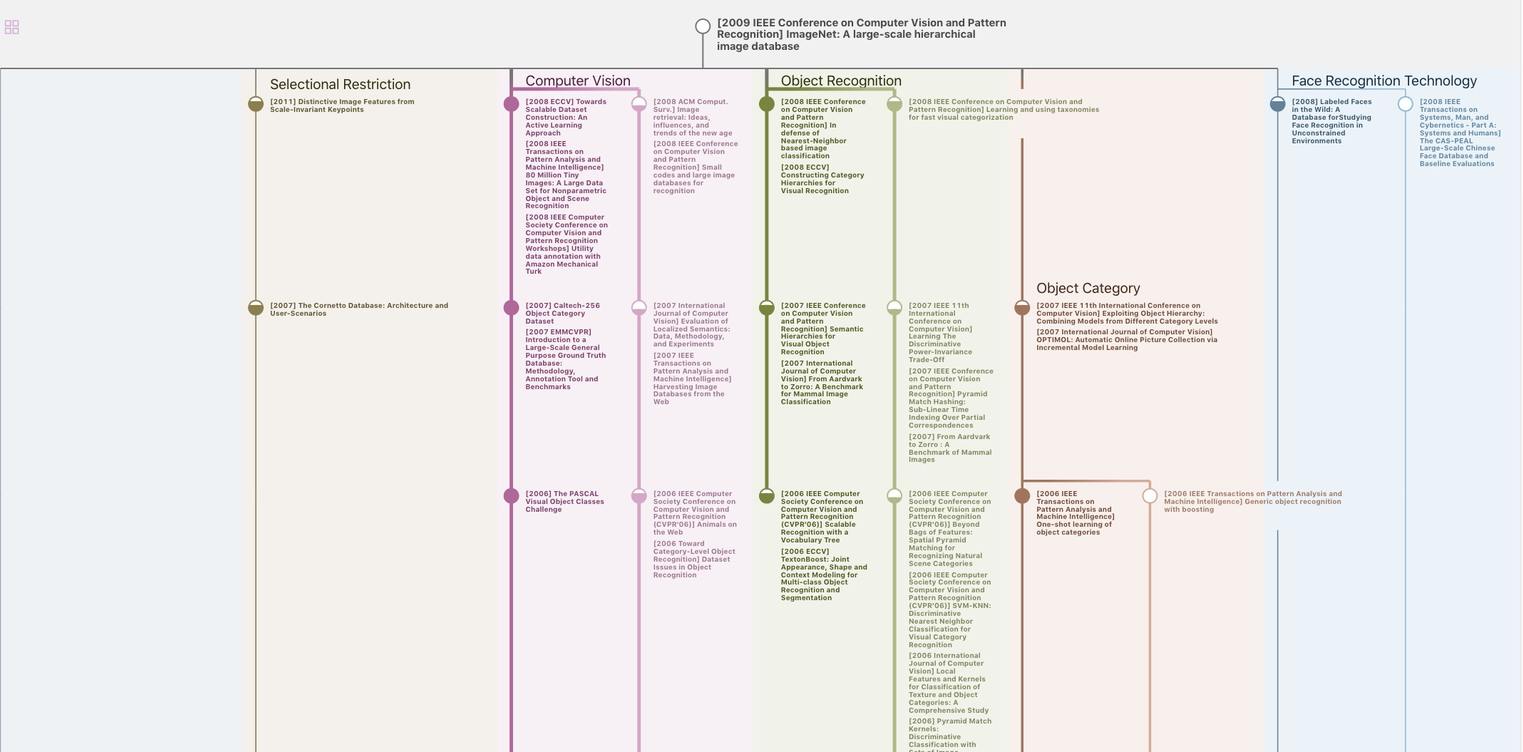
生成溯源树,研究论文发展脉络
Chat Paper
正在生成论文摘要