Towards prescriptive analytics of self-regulated learning strategies: A reinforcement learning approach
BRITISH JOURNAL OF EDUCATIONAL TECHNOLOGY(2024)
摘要
Self-regulated learning (SRL) is an essential skill to achieve one's learning goals. This is particularly true for online learning environments (OLEs) where the support system is often limited compared to a traditional classroom setting. Likewise, existing research has found that learners often struggle to adapt their behaviour to the self-regulatory demands of OLEs. Even so, existing SRL analysis tools have limited utility for real-time or individualised support of a learner's SRL strategy during a study session. Accordingly, we explore a reinforcement learning based approach to learning optimal SRL strategies for a specific learning task. Specifically, we utilise the sequences of SRL processes acted by 44 participants, and their assessment scores for a prescribed learning task, in a purpose-built OLE to develop a long short-term memory (LSTM) network based reward function. This is used to train a reinforcement learning agent to find the optimal sequence of SRL processes for the learning task. Our findings indicate that the developed agents were able to effectively select SRL processes so as to maximise a prescribed learning goal as measured by predicted assessment score and predicted knowledge gains. The contributions of this work will facilitate the development of a tool which can detect sub-optimal SRL strategy in real-time and enable individualised SRL focused scaffolding.
更多查看译文
关键词
learning analytics,learning strategies,reinforcement learning,self-regulated learning
AI 理解论文
溯源树
样例
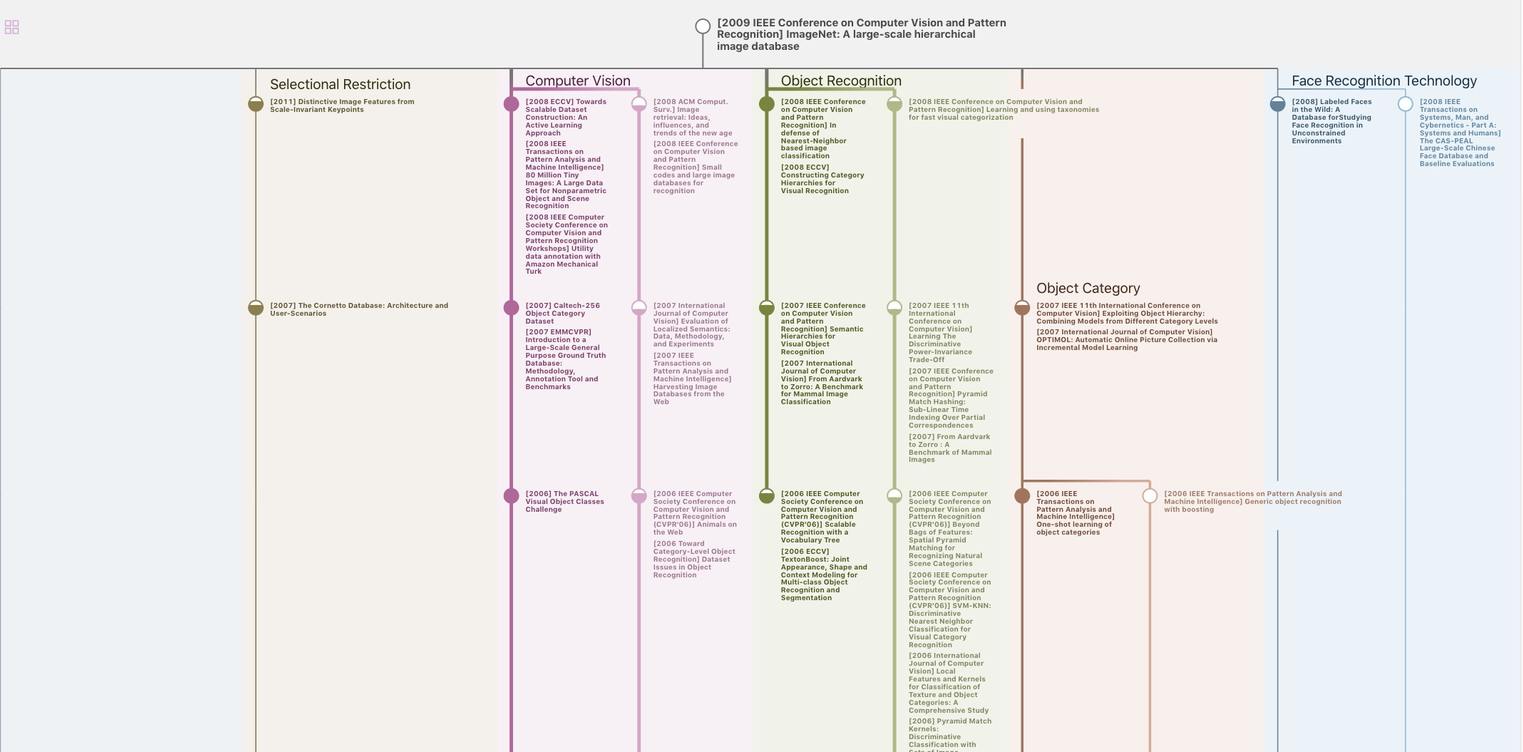
生成溯源树,研究论文发展脉络
Chat Paper
正在生成论文摘要