ViTree: Single-Path Neural Tree for Step-Wise Interpretable Fine-Grained Visual Categorization
AAAI 2024(2024)
摘要
As computer vision continues to advance and finds widespread applications across various domains, the need for interpretability in deep learning models becomes paramount. Existing methods often resort to post-hoc techniques or prototypes to explain the decision-making process, which can be indirect and lack intrinsic illustration. In this research, we introduce ViTree, a novel approach for fine-grained visual categorization that combines the popular vision transformer as a feature extraction backbone with neural decision trees. By traversing the tree paths, ViTree effectively selects patches from transformer-processed features to highlight informative local regions, thereby refining representations in a step-wise manner. Unlike previous tree-based models that rely on soft distributions or ensembles of paths, ViTree selects a single tree path, offering a clearer and simpler decision-making process. This patch and path selectivity enhances model interpretability of ViTree, enabling better insights into the model's inner workings. Remarkably, extensive experimentation validates that this streamlined approach surpasses various strong competitors and achieves state-of-the-art performance while maintaining exceptional interpretability which is proved by multi-perspective methods. Code can be found at https://github.com/SJTU-DeepVisionLab/ViTree.
更多查看译文
关键词
CV: Interpretability, Explainability, and Transparency
AI 理解论文
溯源树
样例
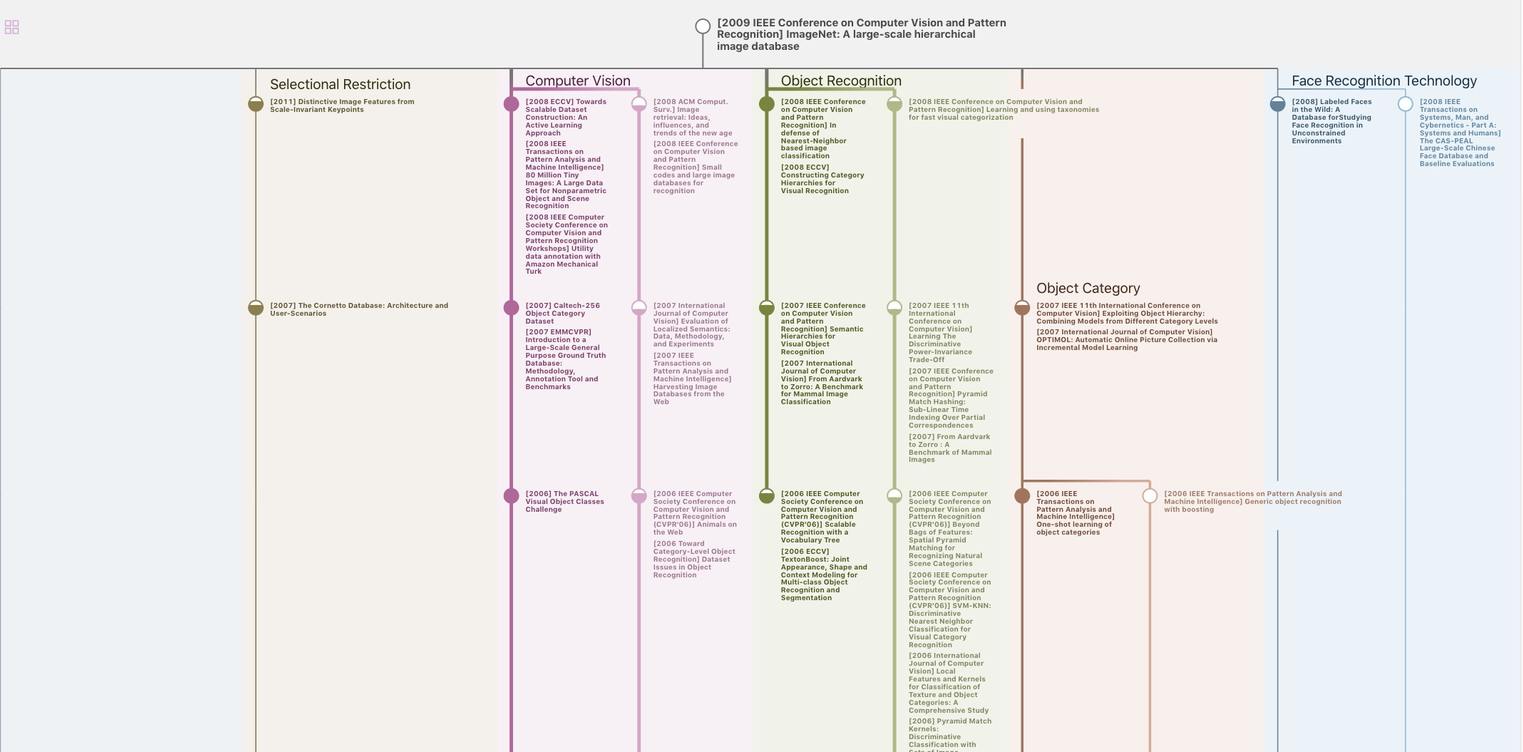
生成溯源树,研究论文发展脉络
Chat Paper
正在生成论文摘要