TCRSCANet: Harnessing Temporal Convolutions and Recurrent Skip Component for Enhanced RUL Estimation in Mechanical Systems
Human-Centric Intelligent Systems(2024)
摘要
Estimating the remaining useful life (RUL) of critical industrial assets is of crucial importance for optimizing maintenance strategies, enabling proactive planning of repair tasks, enhanced reliability, and reduced downtime in prognostic health management (PHM). Deep learning-based data-driven approaches have made RUL prediction a lot better, but traditional methods often do not look at the similarities and differences in the data, which lowers the accuracy of the estimates. Previous attempts to use Long Short-Term Memory (LSTM) networks for RUL prediction have failed because they depend on learned features for regression at the very end of the time step. The single objective function for estimation also constrains the learned representations, which has an impact on RUL estimation. The goal of this study is to find out how to predict the RUL of mechanical systems using complex sensor data. To do this, we present a data-driven framework called temporal convolution, along with a recurrent skip component and an attention mechanism network called TCRSCANet. It uses a combination of temporal convolution, recurrent skip parts, and an attention mechanism to make RUL estimation more accurate. The recurrent skip component finds long-term patterns in time series data, while temporal convolution pulls out high-level features from longer sequences. Finding hidden representations and degradation-development interactions between features at each window position in the input matrix is what the attention layer does to focus on the most important information for RUL estimation. The proposed methodology is tested and validated against the well-established C-MAPSS dataset, which focuses on aircraft degradation. The TCRSCANet model is better at predicting RUL as compared to other state-of-the-art methods because it uses the root mean square error (RMSE) and a scoring function to measure performance. The results of this study demonstrate the importance of the recurrent skip component and attention mechanisms for determining how long an industrial asset will be valuable.
更多查看译文
关键词
Industry 4.0,Smart manufacturing,Recurrent skip component (RSC),Attention layer (AL),Remaining useful life (RUL),Temporal convolution with recurrent-skip-component and attention mechanism network (TCRSCANet)
AI 理解论文
溯源树
样例
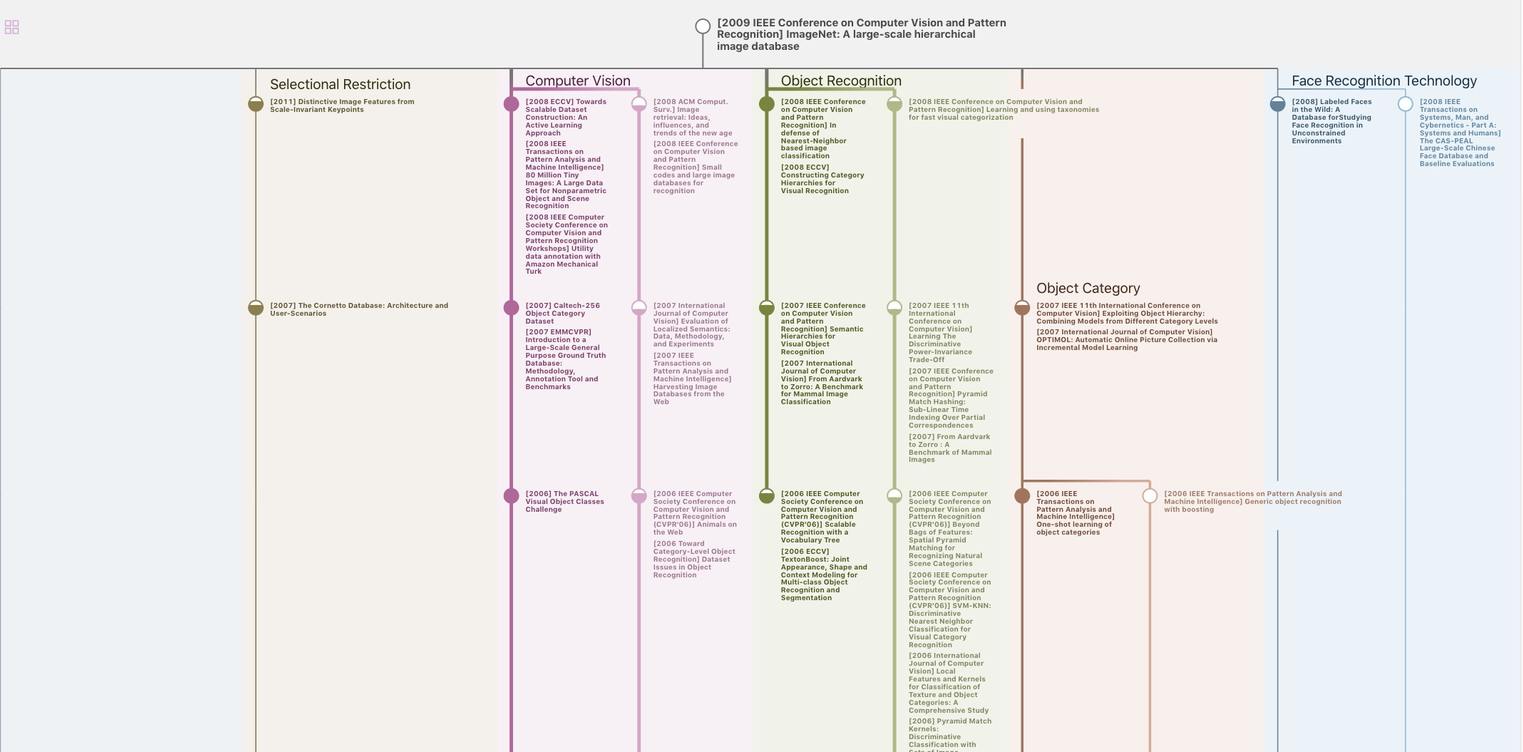
生成溯源树,研究论文发展脉络
Chat Paper
正在生成论文摘要