Unveiling the Unseen: Identifiable Clusters in Trained Depthwise Convolutional Kernels
CoRR(2024)
摘要
Recent advances in depthwise-separable convolutional neural networks
(DS-CNNs) have led to novel architectures, that surpass the performance of
classical CNNs, by a considerable scalability and accuracy margin. This paper
reveals another striking property of DS-CNN architectures: discernible and
explainable patterns emerge in their trained depthwise convolutional kernels in
all layers. Through an extensive analysis of millions of trained filters, with
different sizes and from various models, we employed unsupervised clustering
with autoencoders, to categorize these filters. Astonishingly, the patterns
converged into a few main clusters, each resembling the difference of Gaussian
(DoG) functions, and their first and second-order derivatives. Notably, we were
able to classify over 95% and 90% of the filters from state-of-the-art
ConvNextV2 and ConvNeXt models, respectively. This finding is not merely a
technological curiosity; it echoes the foundational models neuroscientists have
long proposed for the vision systems of mammals. Our results thus deepen our
understanding of the emergent properties of trained DS-CNNs and provide a
bridge between artificial and biological visual processing systems. More
broadly, they pave the way for more interpretable and biologically-inspired
neural network designs in the future.
更多查看译文
关键词
Depthwise Convolutions,Explainability,Neuroscience,Computer Vision,ConvNext
AI 理解论文
溯源树
样例
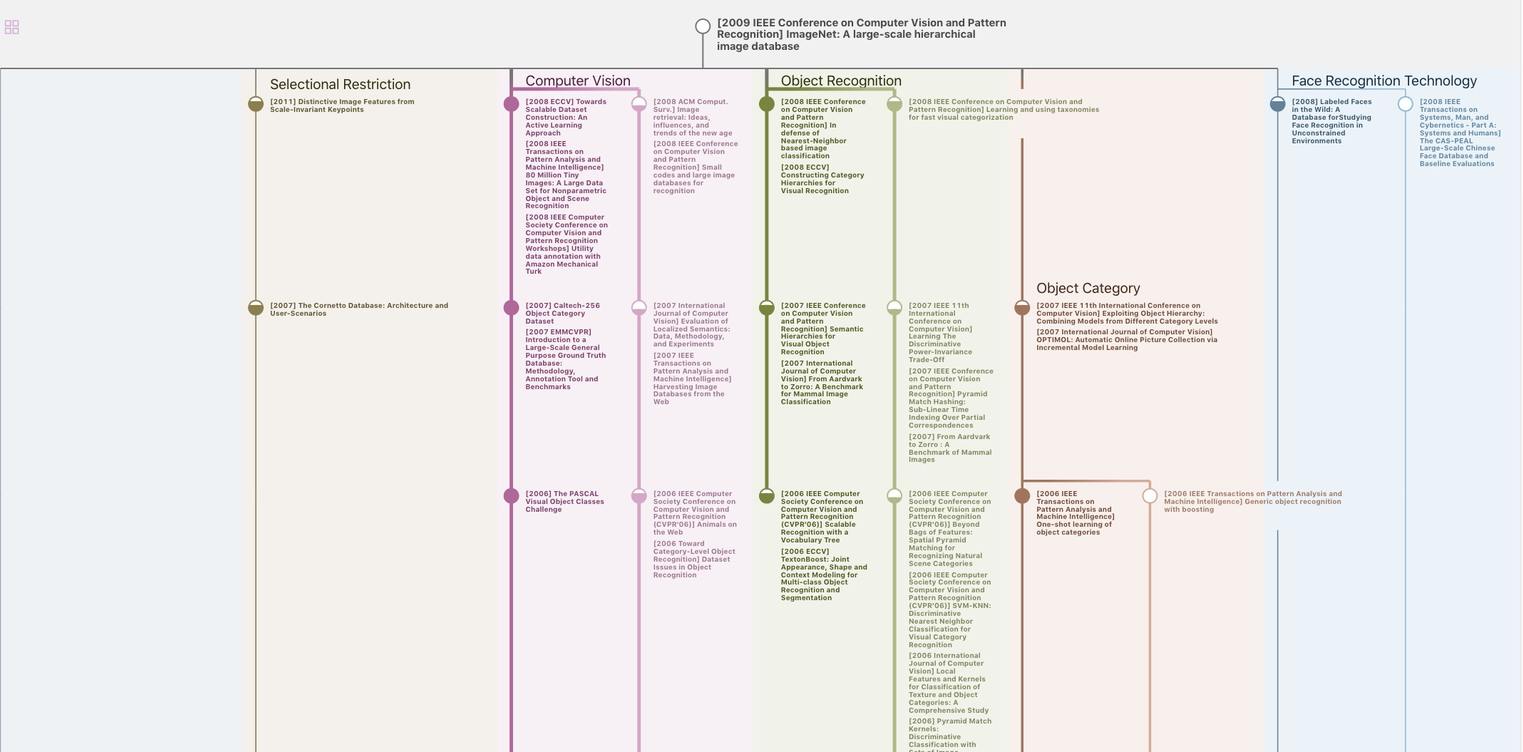
生成溯源树,研究论文发展脉络
Chat Paper
正在生成论文摘要