Electricity demand error corrections with attention bi-directional neural networks
ENERGY(2024)
摘要
Reliable forecast of electricity demand is crucial to stability, supply, and management of electricity grids. Shortterm hourly and sub -hourly demand forecasts are difficult due to the stochastic nature of electricity demand. To improve the electricity demand forecasts, artificial neural networks are combined with attention -based bidirectional neural network as well as Error Correction methods, and Bayesian hyperparameter optimizations. As part of the hybrid VMD-CABLSTM-ANN-EC model, the intrinsic mode functions, representing distinct predictive features, are separated from electricity demand data using variational mode decomposition algorithm. For predicting each intrinsic mode function and respective error time series, convolutional neural networks -bidirectional long short-term memory algorithms are applied. In a second stage, error time series are split, followed by a model to predict the error series' intrinsic mode functions, and a final integration stage to predict the error. The results of the proposed VMD-CABLSTM-ANN-EC model are benchmarked against decompositionbased and standalone models at substations in Queensland, Australia. The proposed VMD-CABLSTM-ANN-EC model outperformed all benchmark models. By splitting the predictor and target variables, it will be shown that the variational model decomposition method improves error corrections by revealing key features of historical demand data. Using error correction method in short-term electricity demand modelling can provide greater confidence in the prediction of electricity demand. The models can be used by energy providers for market analysis and insight research, to manage power failure risk, and make financial decisions.
更多查看译文
关键词
Electricity demand prediction,Sustainable energy,Artificial intelligence,Deep learning,Error correction,Variational mode decomposition
AI 理解论文
溯源树
样例
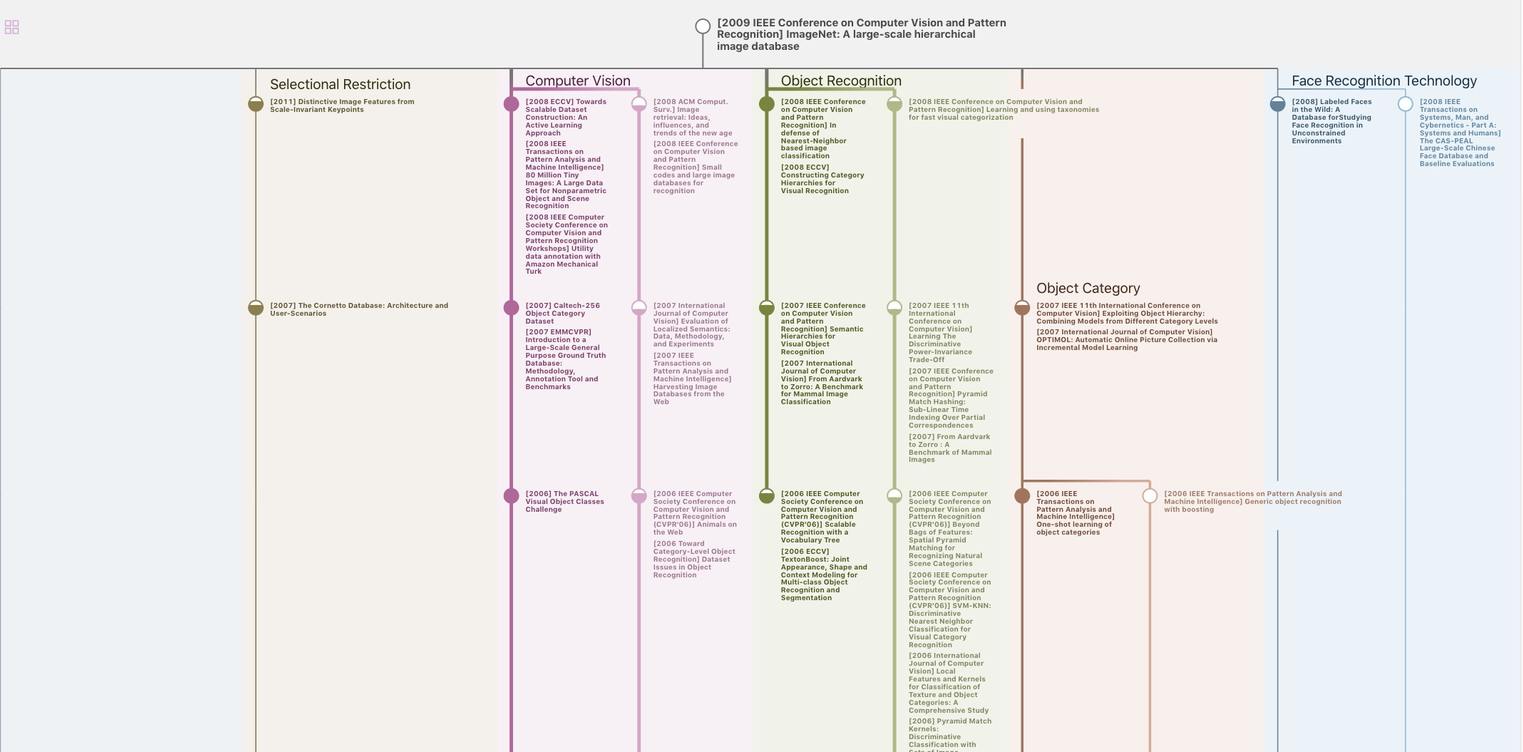
生成溯源树,研究论文发展脉络
Chat Paper
正在生成论文摘要