Tackling Challenges of Low-texture and Illumination Variations for Endoscopy Self-supervised Monocular Depth Estimation.
2023 IEEE International Conference on Bioinformatics and Biomedicine (BIBM)(2023)
摘要
Extracting 3D environmental insights from endoscopy images holds immense value for minimally invasive surgical procedures. The Self-supervised Monocular Depth Estimation (SMDE) framework is promising for achieving this objective. However, existing methods struggle with low-texture and drastic illumination fluctuations in endoscopic images. To tackle this, we incorporate Photometric Aliagnment method based on pixel-wise Color Offset, and propose a carefully designed Color Offset penalty based on Reconstruction Confidence. We furthur apply an Auto-Mask mechanism and a cutting-edge backbone to enhance the performance. Our experiments employ a dataset of airway intubation images captured with a low-resolution and near-field electronic bronchoscope. The experimental results unequivocally highlight the exceptional performance of our approach, achieving root mean square error of 1.64 ± 0.22 mm after point cloud registration, which decreases by 16% than the current SoTA. Furthermore, we introduce an innovative evaluation metric rooted in Photometric Alignment Symmetry, and conduct ablation experiments on this metric. The ablation experiments furthur validate the effectiveness of proposed modules. This study underscores the efficacy of the Reconstruction Confidence-based Color Offset penalty and symmetric alignment evaluations in extracting 3D information from low-resolution and near-field endoscopy images.
更多查看译文
关键词
self-supervised monocular endoscopy depth estimation,low-texture,photometric alignment,reconstruction confidence
AI 理解论文
溯源树
样例
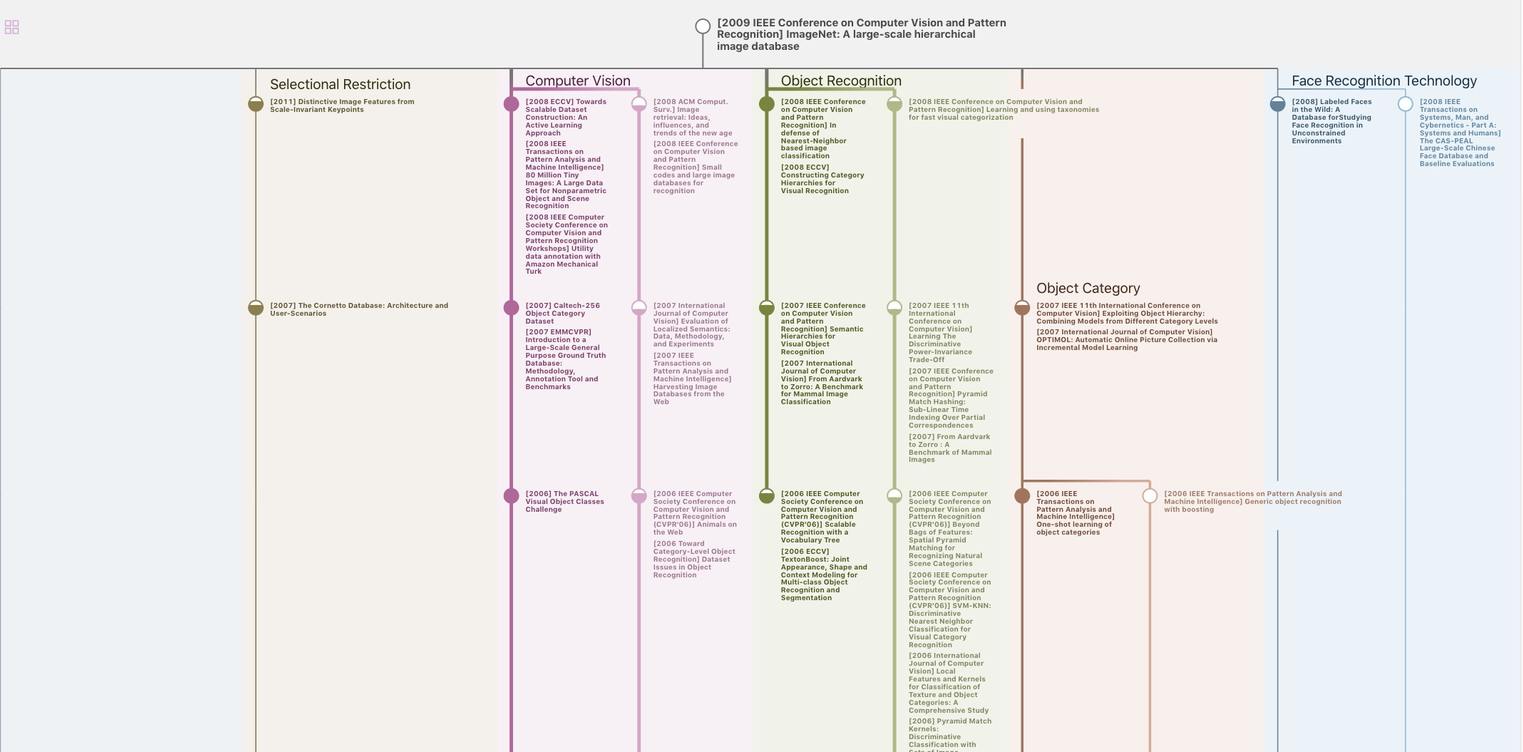
生成溯源树,研究论文发展脉络
Chat Paper
正在生成论文摘要