On Several New Dempster-Shafer-Inspired Uncertainty Measures Applicable for Active Learning
ROUGH SETS, IJCRS 2023(2023)
摘要
Uncertainty-based sampling is one of the most successful and commonly used techniques in active learning. The key element of this approach is an uncertainty function that measures the informativeness of cases from the data pool and is one of the main query selection criteria. In this paper, we investigate the mathematical properties of popular uncertainty functions. We also propose a new family of functions that are inspired by Dempster-Shafer's theory of evidence. Finally, we conduct a series of experiments in which we test the proposed functions against commonly used benchmarks. We argue that our approach is a safe choice for real-life active learning applications.
更多查看译文
关键词
active learning,uncertainty-based sampling,theory of evidence
AI 理解论文
溯源树
样例
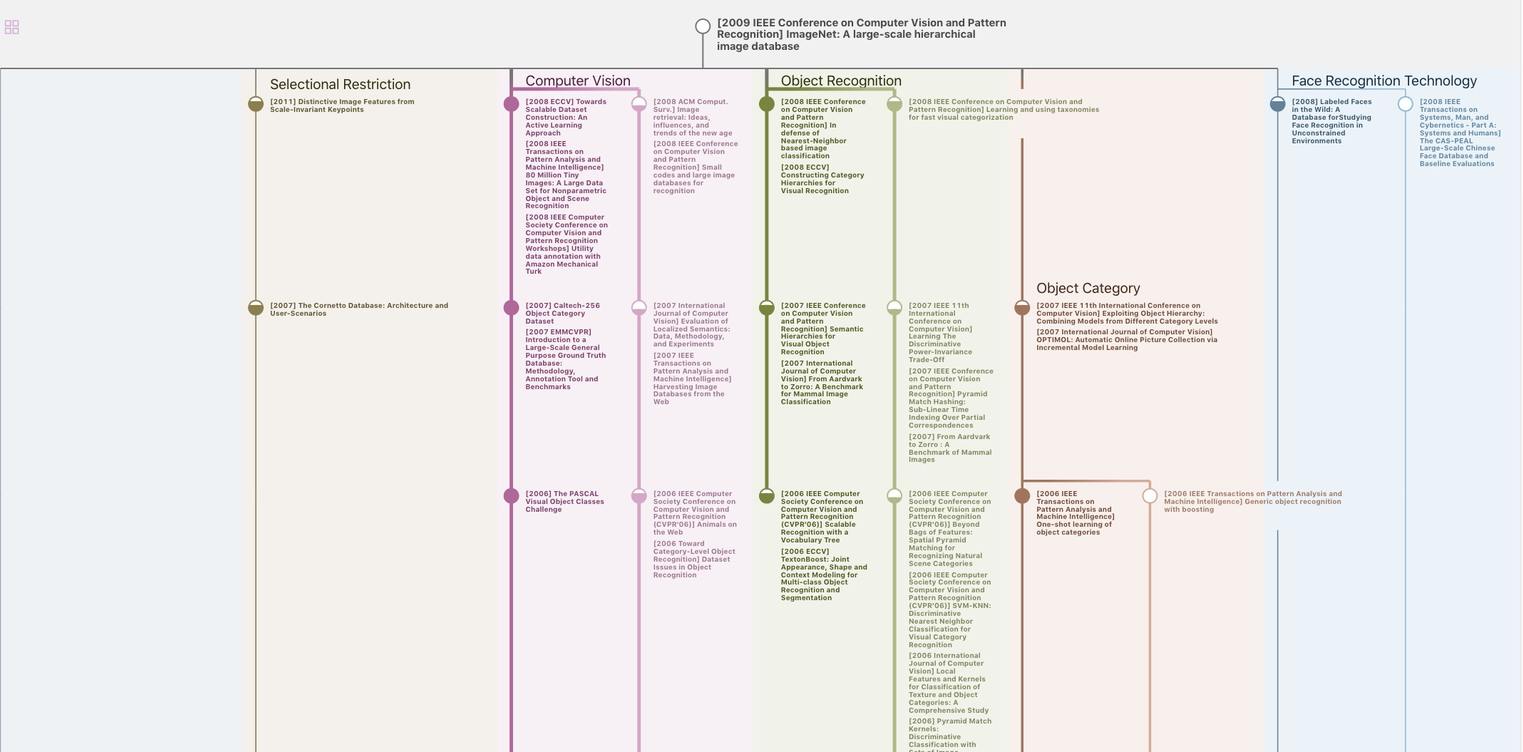
生成溯源树,研究论文发展脉络
Chat Paper
正在生成论文摘要