Graph spatiotemporal process for multivariate time series anomaly detection with missing values
INFORMATION FUSION(2024)
摘要
The detection of anomalies in multivariate time series data is crucial for various practical applications, including smart power grids, traffic flow forecasting, and industrial process control. However, real -world time series data is usually not well -structured, posting significant challenges to existing approaches: (1) The existence of missing values in multivariate time series data along variable and time dimensions hinders the effective modeling of interwoven spatial and temporal dependencies, resulting in important patterns being overlooked during model training; (2) Anomaly scoring with irregularly -sampled observations is less explored, making it difficult to use existing detectors for multivariate series without fully -observed values. In this work, we introduce a novel framework called GST-Pro, which utilizes a graph spatiotemporal process and anomaly scorer to tackle the aforementioned challenges in detecting anomalies on irregularly -sampled multivariate time series. Our approach comprises two main components. First, we propose a graph spatiotemporal process based on neural controlled differential equations. This process enables effective modeling of multivariate time series from both spatial and temporal perspectives, even when the data contains missing values. Second, we present a novel distribution -based anomaly scoring mechanism that alleviates the reliance on complete uniform observations. By analyzing the predictions of the graph spatiotemporal process, our approach allows anomalies to be easily detected. Our experimental results show that the GST-Pro method can effectively detect anomalies in time series data and outperforms state-of-the-art methods, regardless of whether there are missing values present in the data. Our code is available: https://github.com/huankoh/GST-Pro.
更多查看译文
关键词
Time series,Anomaly forecasting,Graph neural networks
AI 理解论文
溯源树
样例
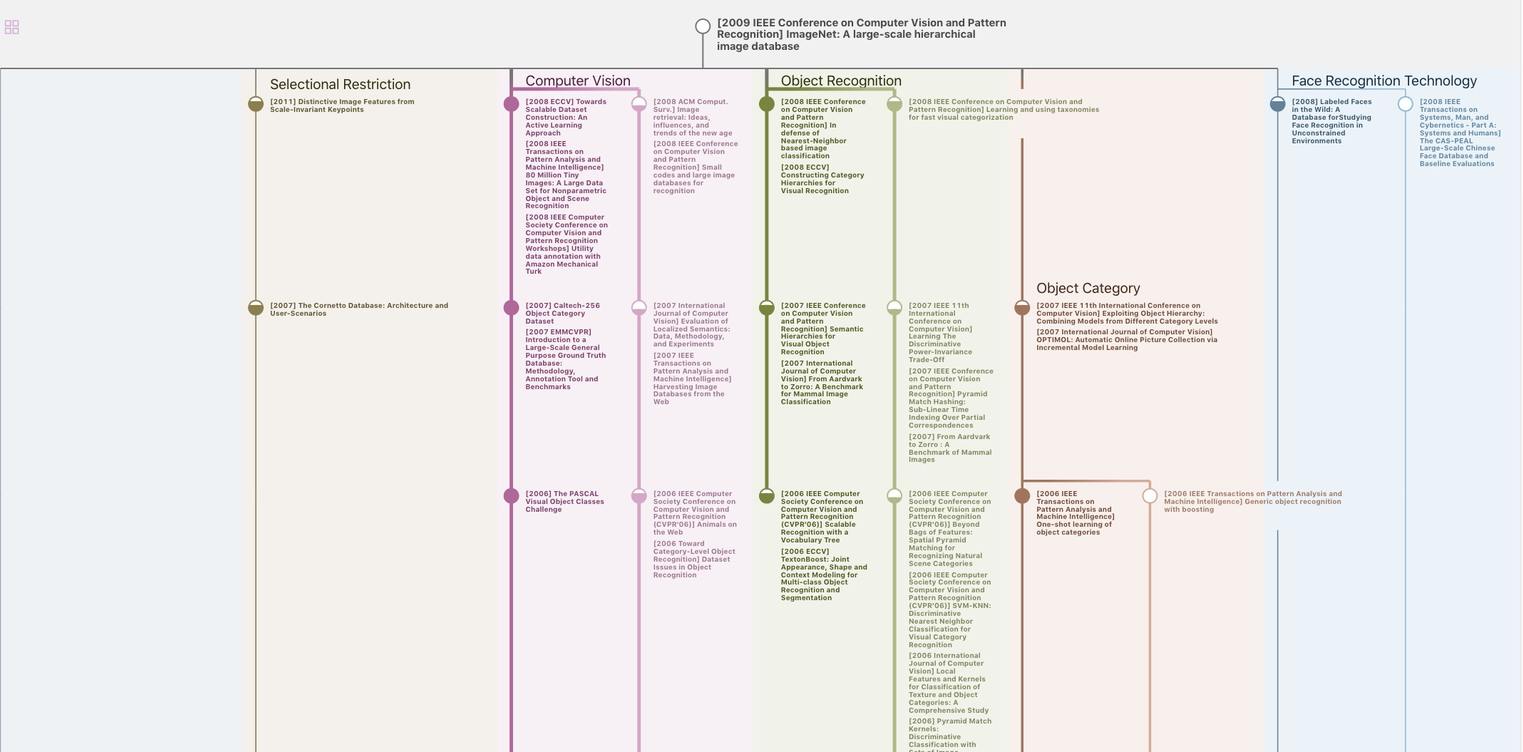
生成溯源树,研究论文发展脉络
Chat Paper
正在生成论文摘要