Cross-Spatiotemporal Graph Convolution Networks for Skeleton-Based Parkinsonian Gait MDS-UPDRS Score Estimation
IEEE TRANSACTIONS ON NEURAL SYSTEMS AND REHABILITATION ENGINEERING(2024)
摘要
Gait impairment in Parkinson's Disease (PD) is quantitatively assessed using the Movement Disorder Society Unified Parkinson's Disease Rating Scale (MDS-UPDRS), a well-established clinical tool. Objective and efficient PD gait assessment is crucial for developing interventions to slow or halt its advancement. Skeleton-based PD gait MDS-UPDRS score estimation has attracted increasing interest in improving diagnostic efficiency and objectivity. However, previous works ignore the important cross-spacetime dependencies between joints in PD gait. Moreover, existing PD gait skeleton datasets are very small, which is a big issue in deep learning-based gait studies. In this work, we collect a sizable PD gait skeleton dataset by multi-view Azure Kinect sensors. The collected dataset contains 102 PD patients and 30 healthy older adults. In addition, gait data from 16 young adults (aged 24-50 years) are collected to further examine the effect of age on PD gait assessment. For skeleton-based automatic PD gait analysis, we propose a novel cross-spatiotemporal graph convolution network (CST-GCN) to learn complex features of gait patterns. Specifically, a gait graph labeling strategy is designed to assemble and group cross-spacetime neighbors of the root node according to the spatiotemporal semantics of the gait skeleton. Based on this strategy, the CST-GCN module explicitly models the cross-spacetime dependencies among joints. Finally, a dual-path model is presented to realize the modeling and fusion of spatial, temporal, and cross-spacetime gait features. Extensive experiments validate the effectiveness of our method on the collected dataset.
更多查看译文
关键词
Parkinsonian gait,quantitative assessment,graph convolutional network,skeleton-based data
AI 理解论文
溯源树
样例
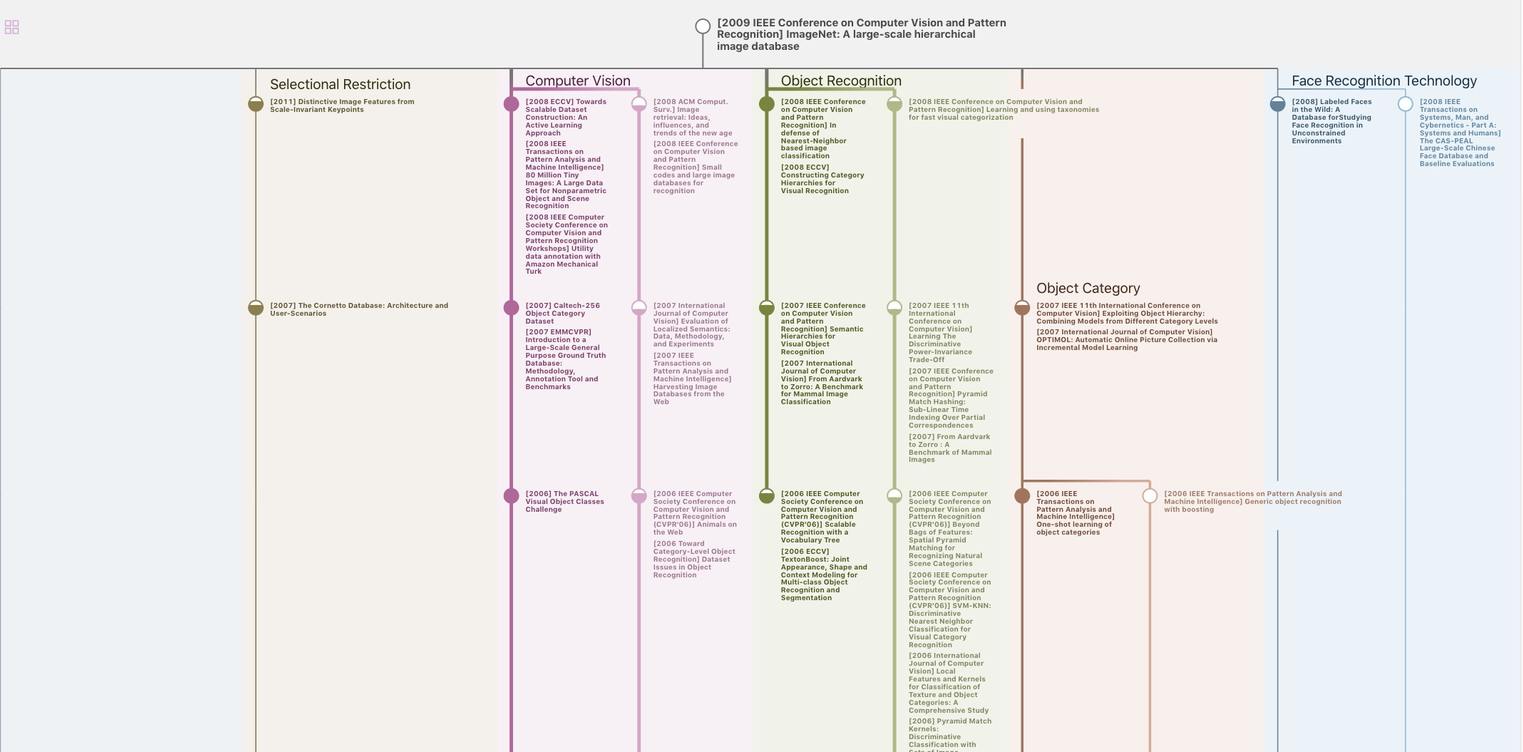
生成溯源树,研究论文发展脉络
Chat Paper
正在生成论文摘要