Graph Reasoning With Supervised Contrastive Learning for Legal Judgment Prediction
IEEE TRANSACTIONS ON NEURAL NETWORKS AND LEARNING SYSTEMS(2024)
摘要
Given the fact descriptions of legal cases, the legal judgment prediction (LJP) problem aims to determine three judgment tasks of law articles, charges, and the term of penalty. Most existing studies have considered task dependencies while neglecting the prior dependencies of labels among different tasks. Therefore, how to make better use of the information on the relation dependencies among tasks and labels becomes a crucial issue. To this end, we transform the text classification problem into a node classification framework based on graph reasoning and supervised contrastive learning (SCL) techniques, named GraSCL. Specifically, we first design a graph reasoning network to model the potential dependency structures and facilitate relational learning under various graph topologies. Then, we introduce the SCL method for the LJP task to further leverage the label relation on the graph. To accommodate the node classification settings, we extend the traditional SCL method to novel variants for SCL at the node level, which allows the GraSCL framework to be trained efficiently even with small batches. Furthermore, to recognize the importance of hard negative samples in contrastive learning, we introduce a simple yet effective technique called online hard negative mining (OHNM) to enhance our SCL approach. This technique complements our SCL method and enables us to control the number and complexity of negative samples, leading to further improvements in the model's performance. Finally, extensive experiments are conducted on two well-known benchmarks, demonstrating the effectiveness and rationality of our proposed SCL approach as compared to the state-of-the-art competitors.
更多查看译文
关键词
Task analysis,Law,Cognition,Topology,Learning systems,Network topology,Transformers,Graph reasoning,legal judgment prediction (LJP),supervised contrastive learning (SCL)
AI 理解论文
溯源树
样例
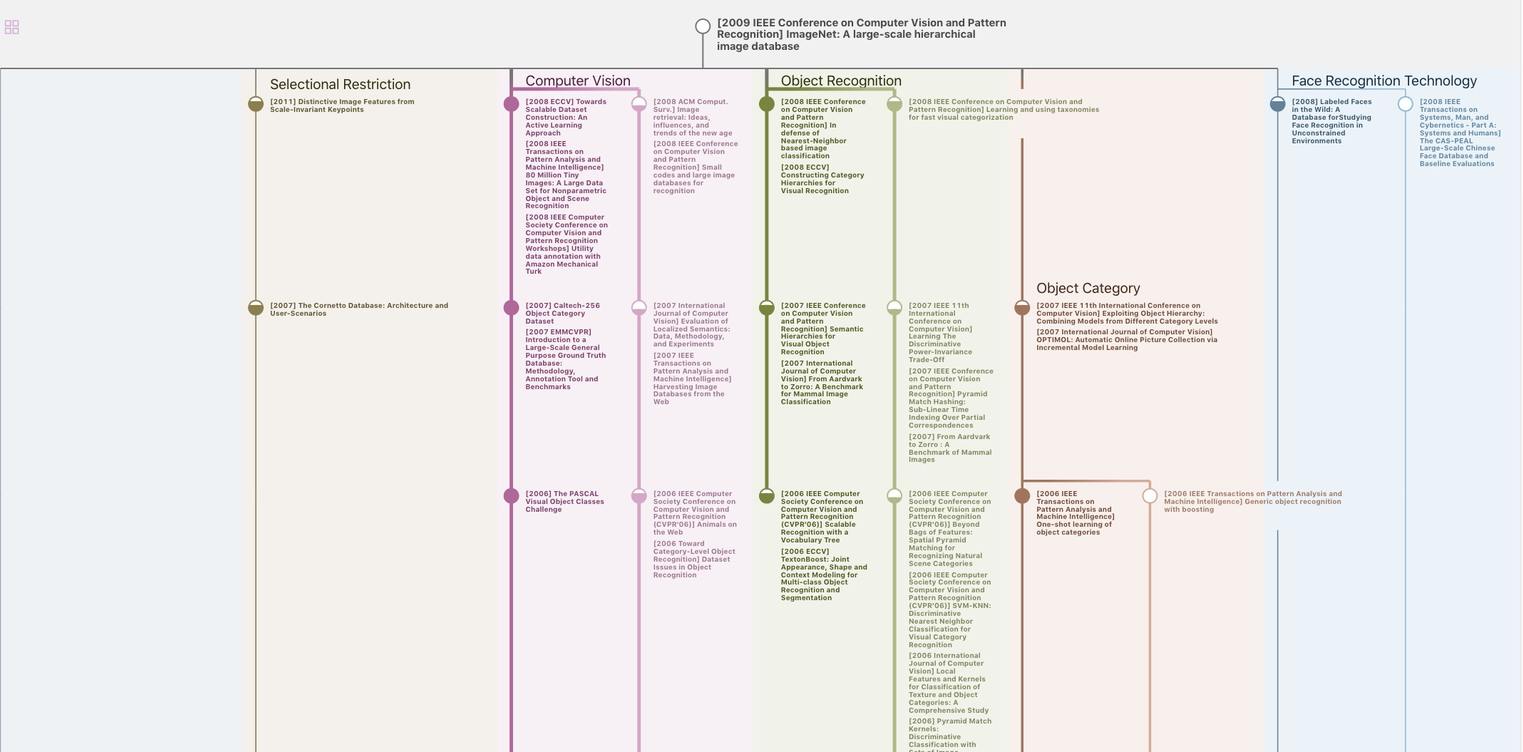
生成溯源树,研究论文发展脉络
Chat Paper
正在生成论文摘要