SLAH: A Siamese Network Vision Tracking Algorithm Using LSTM Adaptive Head
2023 13th International Conference on Information Science and Technology (ICIST)(2023)
摘要
In the field of single object tracking, conventional methods often rely on correlation filters or visual image processing. However, these approaches typically focus solely on extracting target object features and consider only the position information in the current frame. They lack integration of contextual and spatial-temporal information, which can limit tracking accuracy.Our method takes inspiration from single object detection and tracking techniques. It combines efficient model-based object detection with the spatial position of the final target in the image. This approach offers significant advantages in reducing tracking drift (deviation from the target object) and improving tracking accuracy (predicting bounding boxes that closely follow the target).To extract spatial information, we utilize a three-layer ResNet and Convolutional Block Attention Module (CBAM) in conjunction with a siamese network. This combination effectively captures the spatial characteristics of the target object. Additionally, we employ an adaptive processing head with an internal structure of Long Short Term Memory (LSTM) to capture temporal information. By integrating spatial and temporal cues, our method achieves more robust and accurate tracking.Through comprehensive comparison and ablation experiments across multiple datasets, we have demonstrated notable improvements with our method. It tightly connects the predicted bounding box with the tracking target and effectively combines spatial and temporal information for precise object tracking.
更多查看译文
关键词
Siamese network,VOT,Lstm,Attention
AI 理解论文
溯源树
样例
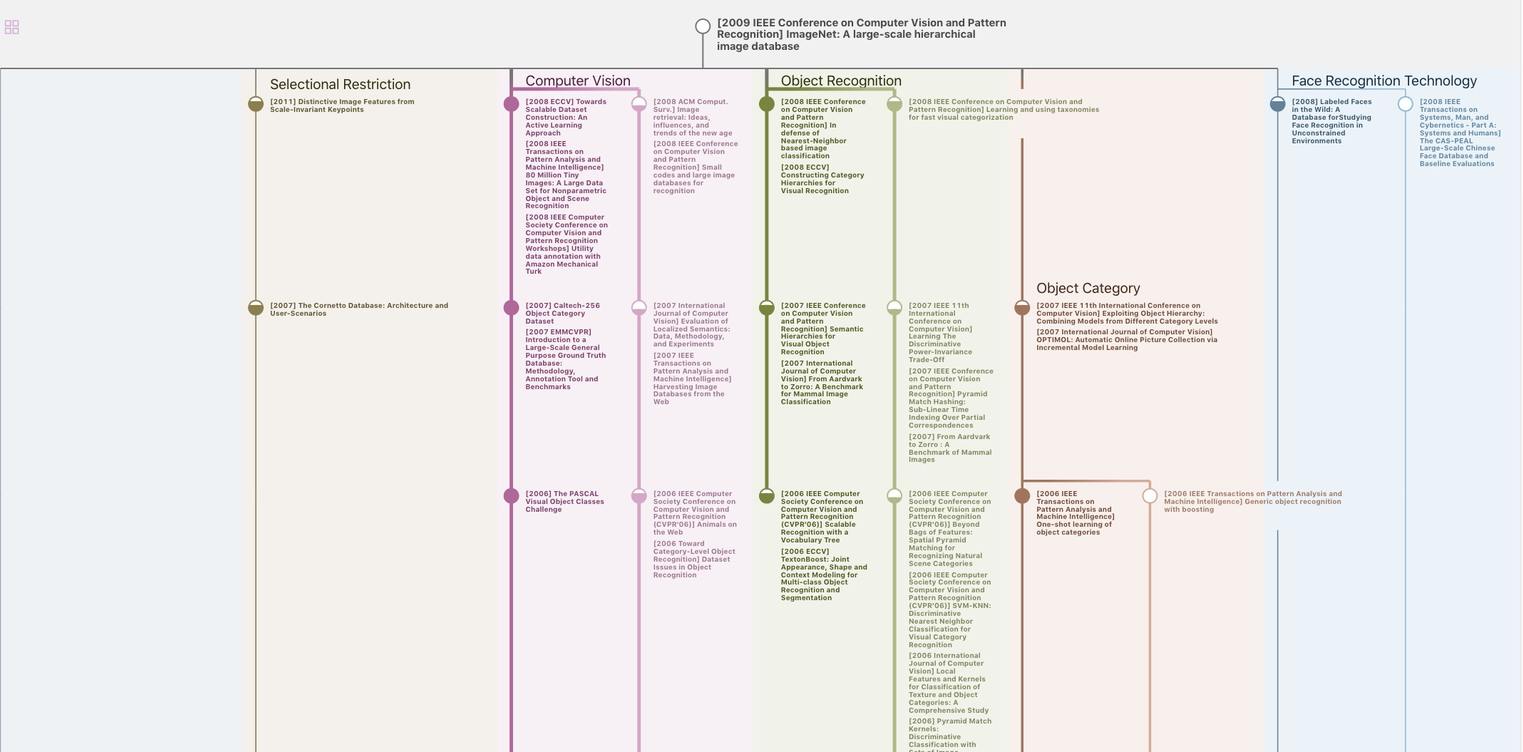
生成溯源树,研究论文发展脉络
Chat Paper
正在生成论文摘要