YOLO SRv2: An evolved version of YOLO SR
Engineering Applications of Artificial Intelligence(2024)
摘要
Recently, object detection based on deep neural network has become the mainstream research direction of sweeping robots since different obstacles may produce completely contradictory sweeping decisions. However, due to the limited computational ability of the sweeping robot, the size of the object detection network is constrained, which leads to relatively low recognition accuracy and makes it difficult to be applied practically. To address this problem, we present a novel lightweight object detection network, namely YOLO_SRv2, which is an evolved version of our previously proposed You Only Look Once network for Sweeping Robots (YOLO_SR). First, an improved max-pooling structure is presented in the backbone network to avoid losing the suboptimal features. Second, a Convolutional Block Attention Module (CBAM) is added behind the backbone network to enhance the extraction of important features. Finally, a decoupled head module is included to replace the original couple head so as to solve the conflict between classification and regression tasks. In addition, we optimize the classification loss methods to improve the detection performance of hard examples. In the experimental studies, we evaluated the proposed YOLO_SRv2 with other 7 state-of-the-art methods on the ODSR-HIS dataset and PASCAL VOC dataset. The experimental results show that YOLO_SRv2 achieves 86.1% mAP on the ODSR-HIS dataset and 78.2% mAP on PASCAL VOC dataset with 180 FPS on RTX 2080Ti, which verifies the efficiency and the effectiveness of the proposed method as a lightweight object detection network. Source code is at https://github.com/lvyongshjd/YOLO_SRv2.
更多查看译文
关键词
Object detection,Sweeping robots,Lightweight network,Decoupled head
AI 理解论文
溯源树
样例
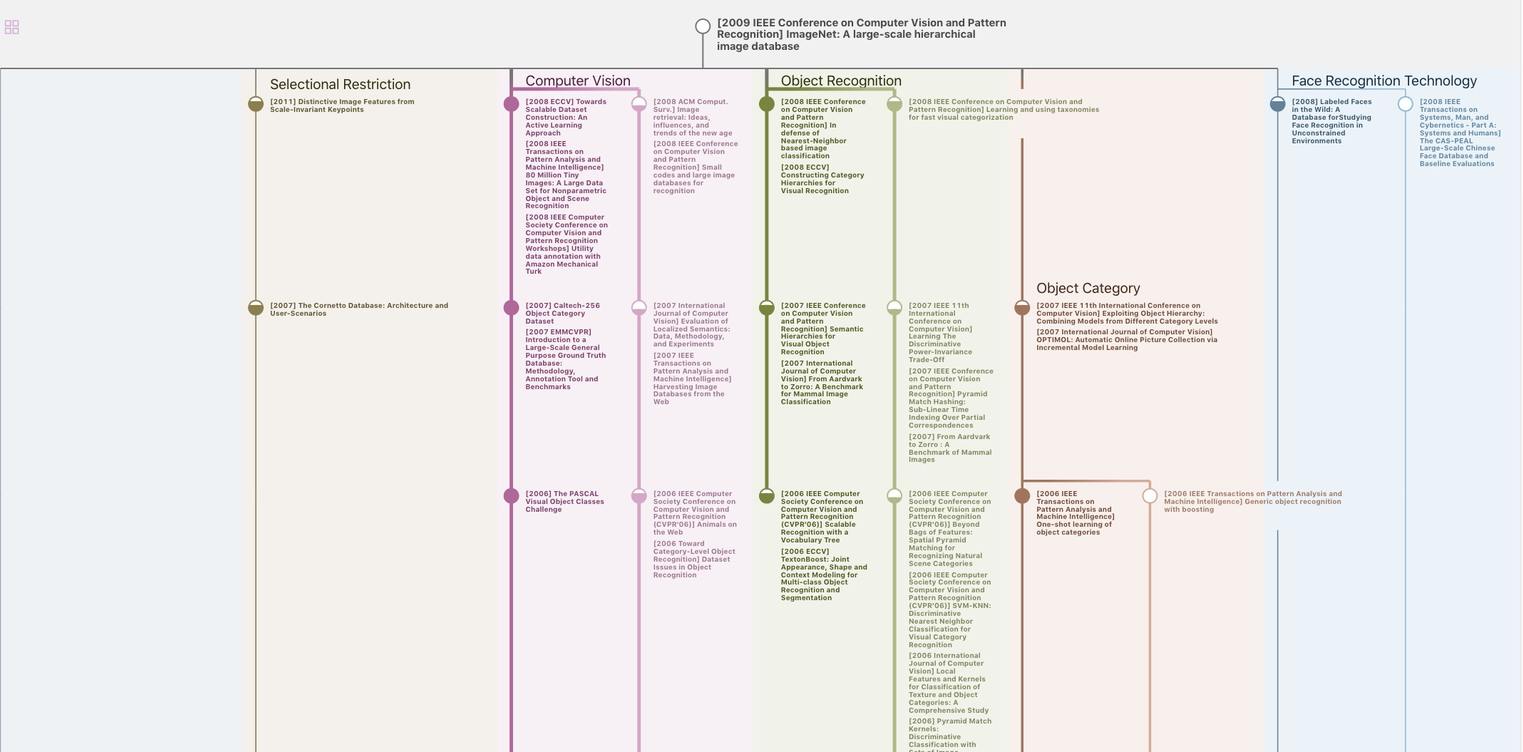
生成溯源树,研究论文发展脉络
Chat Paper
正在生成论文摘要