Anomaly detection via gating highway connection for retinal fundus images
PATTERN RECOGNITION(2024)
摘要
Since the labels for medical images are challenging to collect in real scenarios, especially for rare diseases, fully supervised methods cannot achieve robust performance for clinical anomaly detection. Recent research tried to tackle this problem by training the anomaly detection framework using only normal data. Reconstruction-based methods, e.g., auto-encoder, achieved impressive performances in the anomaly detection task. However, most existing methods adopted the straightforward backbone architecture (i.e., encoder-and-decoder) for image reconstruction. The design of a skip connection, which can directly transfer information between the encoder and decoder, is rarely used. Since the existing U-Net has demonstrated the effectiveness of skip connections for image reconstruction tasks, in this paper, we first use the dynamic gating strategy to achieve the usage of skip connections in existing reconstruction-based anomaly detection methods and then propose a novel gating highway connection module to adaptively integrate skip connections into the framework and boost its anomaly detection performance, namely GatingAno. Furthermore, we formulate an auxiliary task, namely histograms of oriented gradients (HOG) prediction, to encourage the framework to exploit contextual information from fundus images in a self-driven manner, which increases the robustness of feature representation extracted from the healthy samples. Last but not least, to improve the model generalization for anomalous data, we introduce an adversarial strategy for the training of our multi-task framework. Experimental results on the publicly available datasets, i.e., IDRiD and ADAM, validate the superiority of our method for detecting abnormalities in retinal fundus images. The source code is available at https://github.com/WentianZhang-ML/GatingAno.
更多查看译文
关键词
Anomaly detection,Feature prediction,Fundus image,Skip connection
AI 理解论文
溯源树
样例
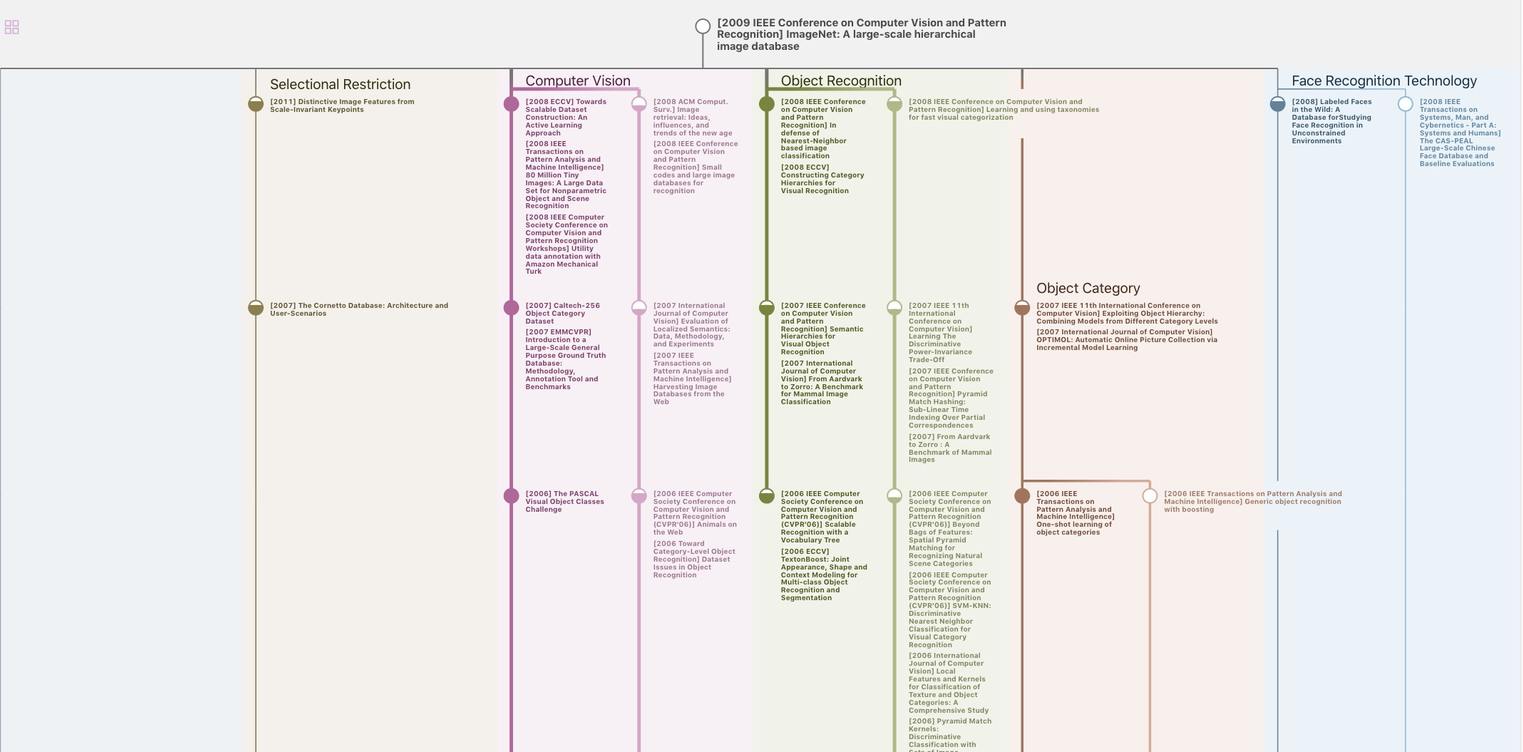
生成溯源树,研究论文发展脉络
Chat Paper
正在生成论文摘要