Untangling Multiple Deformable Linear Objects in Unknown Quantities With Complex Backgrounds
IEEE TRANSACTIONS ON AUTOMATION SCIENCE AND ENGINEERING(2024)
摘要
The manipulation of deformable objects, especially deformable linear objects (DLOs), represents an open challenge in robotics. The keys to solving the problem are accurately recognizing the topological model describing the entwined state of DLO(s) and a manipulation strategy based on it. The situation becomes more challenging in practical applications since the DLO(s) may be placed under complex and unencountered environments, making distinguishing between the targets and the background difficult. In addition, with the information obtained from a sensor system with a limited field of sense, a robot has to treat the encountered DLO(s) as multiple ones of an unknown quantity. This paper proposes a solution based on deep learning techniques for these complicated scenarios. The approach can derive a topological model describing the entangled structure of single or multiple DLOs. Based on the model, we proposed a strategy for untangling the DLO(s), considering both the possible self-tangling in one DLO and the tangling between multiple DLOs. The strategy ensures that the entangled DLO(s) can be arranged to be the neatest state given a limited field of view. The feasibility and effectiveness of the proposed solution were verified by untangling experiments utilizing a dual-arm robot system. Even if the exact quantity of the DLO(s) is unknown, the robots can still untangle the DLO(s). Moreover, the proposed approach performed robustness to unfamiliar background textures, which is preferable in practical applications. Dataset used in this paper can be found at https://github.com/lancexz/dlos-dataset.
更多查看译文
关键词
Automated unknotting,deep learning,deformable object manipulation,grasping planning
AI 理解论文
溯源树
样例
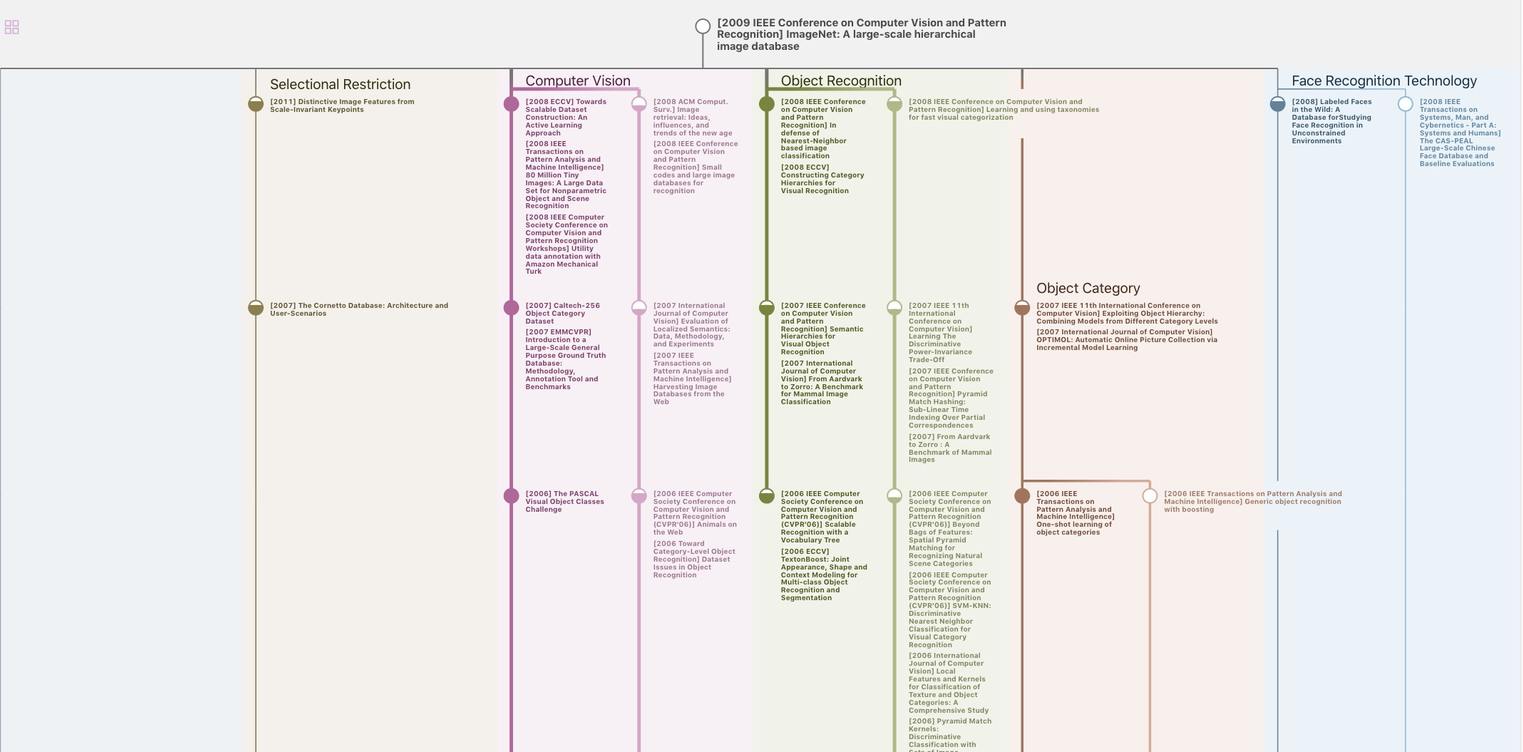
生成溯源树,研究论文发展脉络
Chat Paper
正在生成论文摘要