Escaping the Sample Trap: Fast and Accurate Epistemic Uncertainty Estimation with Pairwise-Distance Estimators
arXiv (Cornell University)(2023)
摘要
In machine learning, the ability to assess uncertainty in model predictions is crucial for decision-making, safety-critical applications, and model generalizability. This work introduces a novel approach for epistemic uncertainty estimation for ensemble models using pairwise-distance estimators (PaiDEs). These estimators utilize the pairwise-distance between model components to establish bounds on entropy, which are then used as estimates for information-based criterion. Unlike recent deep learning methods for epistemic uncertainty estimation, which rely on sample-based Monte Carlo estimators, PaiDEs are able to estimate epistemic uncertainty up to 100 times faster, over a larger input space (up to 100 times) and perform more accurately in higher dimensions. To validate our approach, we conducted a series of experiments commonly used to evaluate epistemic uncertainty estimation: 1D sinusoidal data, $\textit{Pendulum-v0}$, $\textit{Hopper-v2}$, $\textit{Ant-v2}$ and $\textit{Humanoid-v2}$. For each experimental setting, an Active Learning framework was applied to demonstrate the advantages of PaiDEs for epistemic uncertainty estimation.
更多查看译文
关键词
accurate epistemic uncertainty
AI 理解论文
溯源树
样例
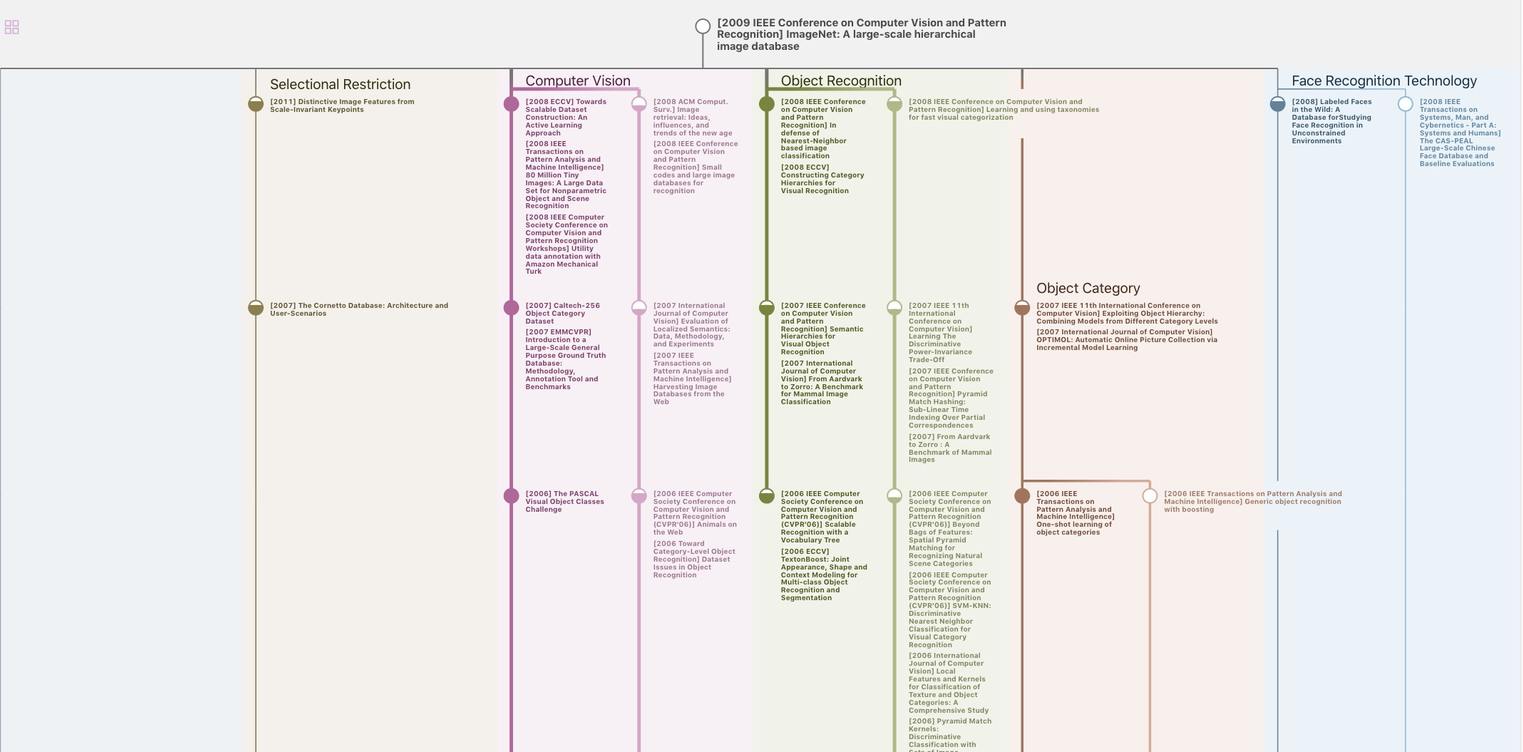
生成溯源树,研究论文发展脉络
Chat Paper
正在生成论文摘要