Effective Multimodal Reinforcement Learning with Modality Alignment and Importance Enhancement
arXiv (Cornell University)(2023)
摘要
Many real-world applications require an agent to make robust and deliberate decisions with multimodal information (e.g., robots with multi-sensory inputs). However, it is very challenging to train the agent via reinforcement learning (RL) due to the heterogeneity and dynamic importance of different modalities. Specifically, we observe that these issues make conventional RL methods difficult to learn a useful state representation in the end-to-end training with multimodal information. To address this, we propose a novel multimodal RL approach that can do multimodal alignment and importance enhancement according to their similarity and importance in terms of RL tasks respectively. By doing so, we are able to learn an effective state representation and consequentially improve the RL training process. We test our approach on several multimodal RL domains, showing that it outperforms state-of-the-art methods in terms of learning speed and policy quality.
更多查看译文
关键词
effective multimodal reinforcement learning,modality alignment,importance
AI 理解论文
溯源树
样例
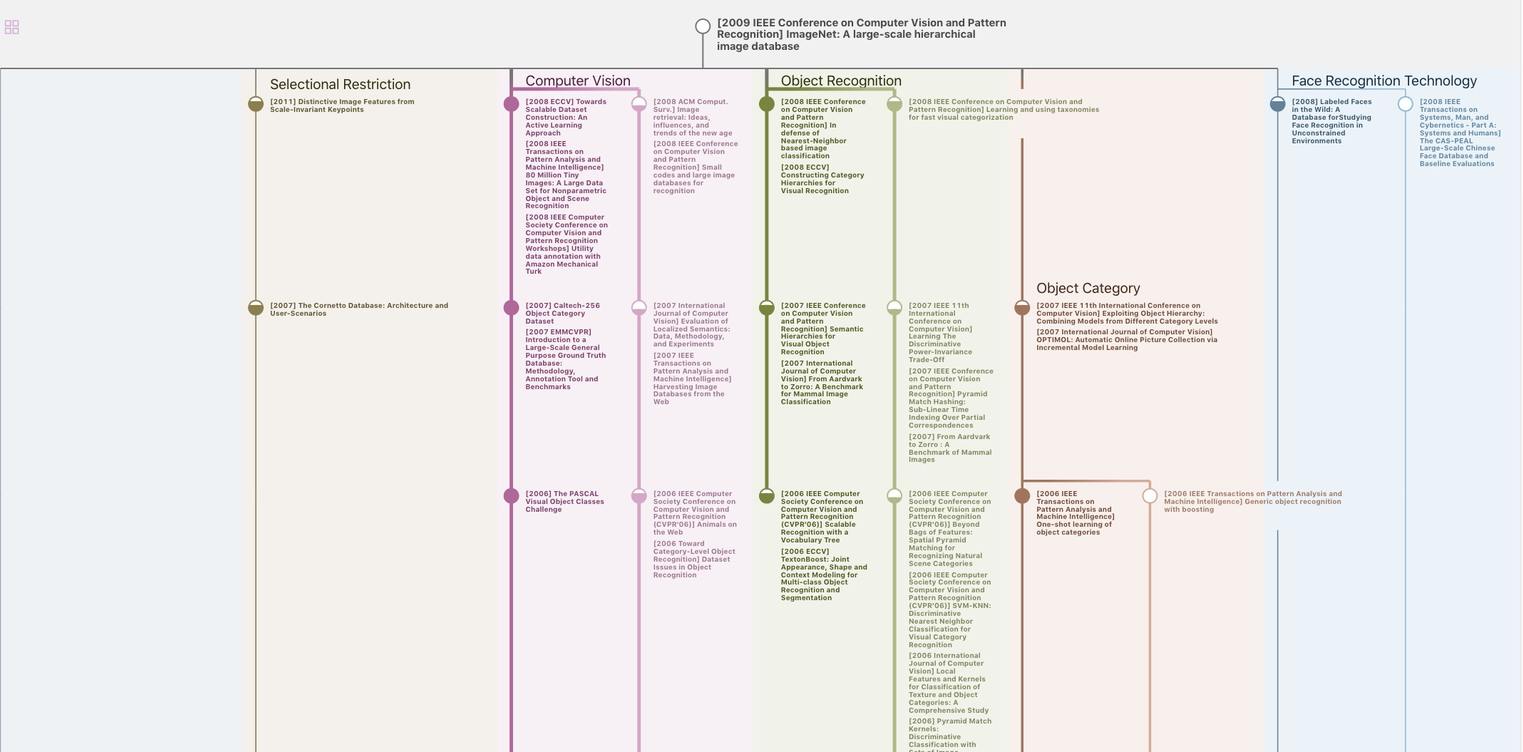
生成溯源树,研究论文发展脉络
Chat Paper
正在生成论文摘要