MCU-Enabled Epileptic Seizure Detection System With Compressed Learning.
IEEE Internet Things J.(2024)
摘要
Epilepsy is one of the most common neurological disorder diseases all over the world, which gives patients a huge burden in seizure-related disabilities. For epileptic seizure detection, encephalography (EEG) is a commonly used clinical approach. Recently, several Internet of Things (IoT) based wearable monitoring systems using machine learning (ML) approaches have been proposed to assist real-time detection of epileptic seizure attack scenarios. Among these approaches, convolutional neural networks (CNNs) provide superior accuracy, at the expense of high computational complexity that is not friendly to resource-constrained wearable devices. In this work, we propose a compressed learning (CL)-based epileptic seizure detection system which enables implementing CNN on microcontroller units (MCUs). The proposed CL approach combines the measurement matrix of compressed sensing (CS) and a 1-D CNN. As a result, the input data size and parameter number of CNN can be significantly reduced while eliminating the complex reconstruction process of the traditional CS approach. Evaluated on the Bonn dataset, our proposed system ensures a 96.44% accuracy under a 0.1 compressed ratio (CR) corresponding to a 33.5× multiply-accumulate operations (MACs) reduction and a 21.9× decrease in model size compared with the baseline. Mapping such a model on an MSP432 MCU, the memory requirement is 50.13 kB and the power consumption is 13.4727 mW at 40 MHz frequency, corresponding to an energy consumption of 269.4 μJ/Classification, with a classification latency of 21.07 ms.
更多查看译文
关键词
Epilepsy,EEG,wearable system,neural network,compressed learning,MCU,end-to-end
AI 理解论文
溯源树
样例
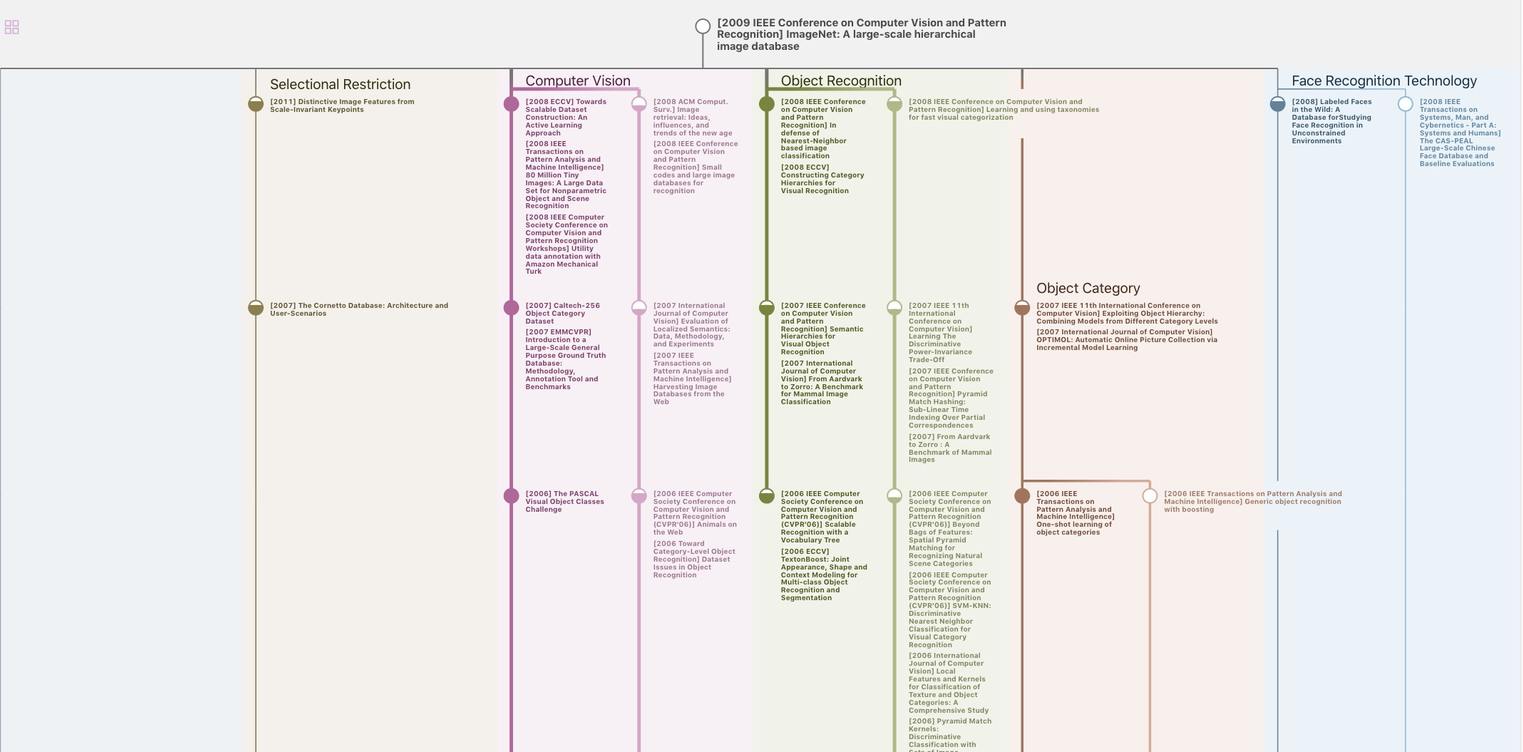
生成溯源树,研究论文发展脉络
Chat Paper
正在生成论文摘要