Modeling Cycle-to-Cycle Variation in Memristors for In-Situ Unsupervised Trace-STDP Learning.
IEEE Trans. Circuits Syst. II Express Briefs(2024)
摘要
Evaluating the computational accuracy of Spiking Neural Network (SNN) implemented as in-situ learning on largescale memristor crossbars remains a challenge due to the lack of a versatile model for the variations in non-ideal memristors. This paper proposes a novel behavioral variation model along with a four-stage pipeline for physical memristors. The proposed variation model combines both absolute and relative variations. Therefore, it can better characterize different memristor cycle-tocycle (C2C) variations in practice. The proposed variation model has been used to simulate the behavior of two physical memristors. Adopting the non-ideal memristor model, the trace-based spiking-timing dependent plasticity (STDP) unsupervised inmemristor learning system is simulated. Although the synapticlevel weight simulation shows a performance degradation of 7.99% and 4.07% increase in the relative root mean square error (RRMSE), the network-level simulation results show no accuracy loss on the MNIST benchmark. Furthermore, the impacts of absolute and relative C2C variations on network performance are simulated and analyzed through two sets of univariate experiments.
更多查看译文
关键词
Memristor,non-ideality,variation model,tracebased STDP,in-situ unsupervised learning
AI 理解论文
溯源树
样例
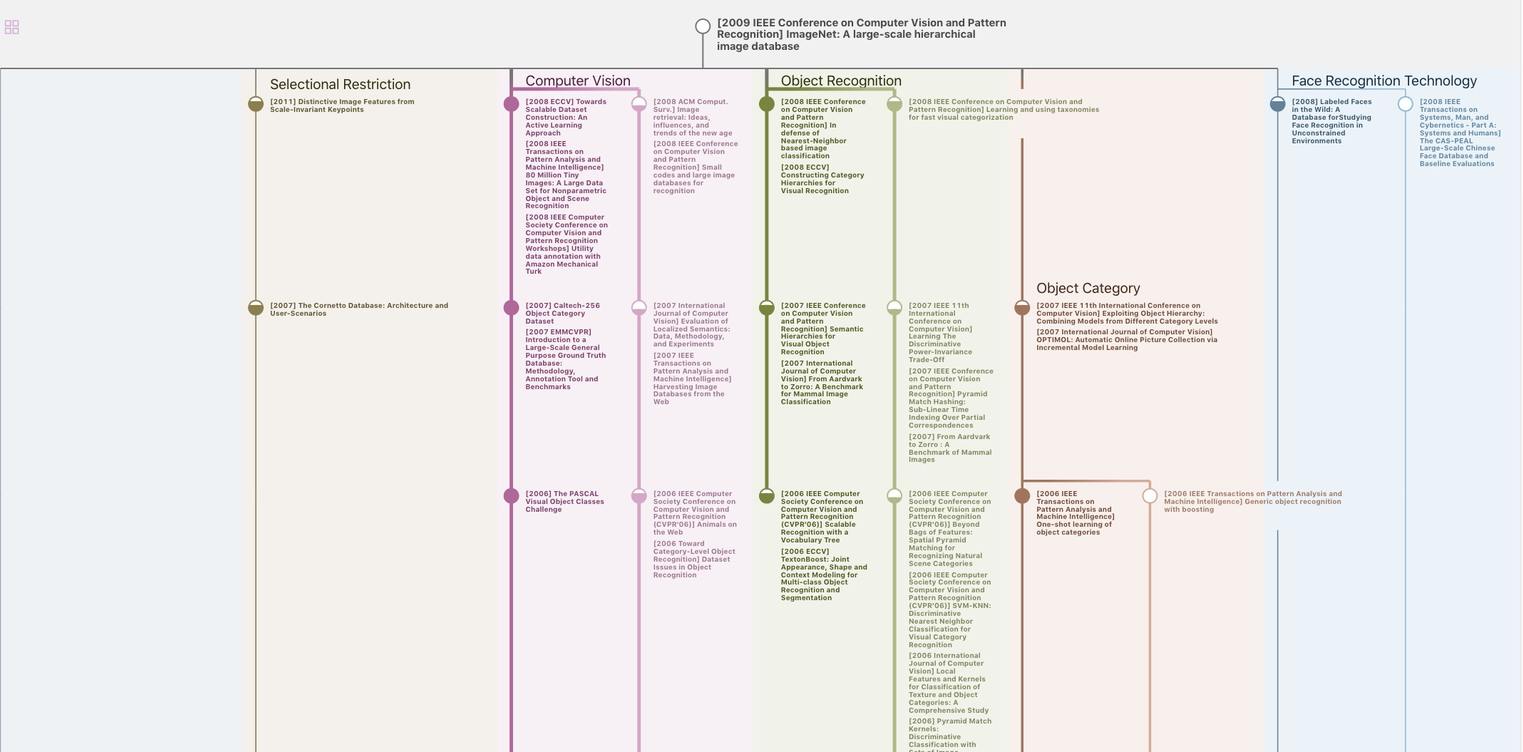
生成溯源树,研究论文发展脉络
Chat Paper
正在生成论文摘要