Uncertainty-Aware Ensemble Combination Method for Quality Monitoring Fault Diagnosis in Safety-Related Products
IEEE TRANSACTIONS ON INDUSTRIAL INFORMATICS(2023)
摘要
With the advent of Industry 4.0 (I4.0) leading to the proliferation of industrial process data, deep learning (DL) techniques have become instrumental in developing intelligent fault diagnosis (FD) applications. However, despite their potentially superior process monitoring capabilities, DL-based FD models are poorly calibrated and generate point estimate predictions without the associated uncertainty estimates. For DL-based FD models, accurate predictive uncertainty estimates from well-calibrated models are essential in ensuring industrial process safety and reliability. This paper proposes Ensemble-to-Distribution (E2D), an uncertainty-aware combination method for quality monitoring FD based on an ensemble of deep neural networks (DNNs). First, E2D addresses safety by providing accurate uncertainty estimates on model predictions, enabling informed decision-making to minimize operational risks. Second, E2D improves model performance on out-of-distribution (OOD) detection tasks to facilitate deployments in the real world. Third, E2D is a post hoc application, implementable at inference time, and compatible with diverse pre-trained models. Finally, to demonstrate the effectiveness of E2D, we explore the problem of monitoring the stability of industrial processes and product quality using case studies on the steel plates faults and APS failure at Scania trucks datasets.
更多查看译文
关键词
Ensemble methods,deep learning,uncertainty estimation,calibration,fault diagnosis,process monitoring,safety-critical
AI 理解论文
溯源树
样例
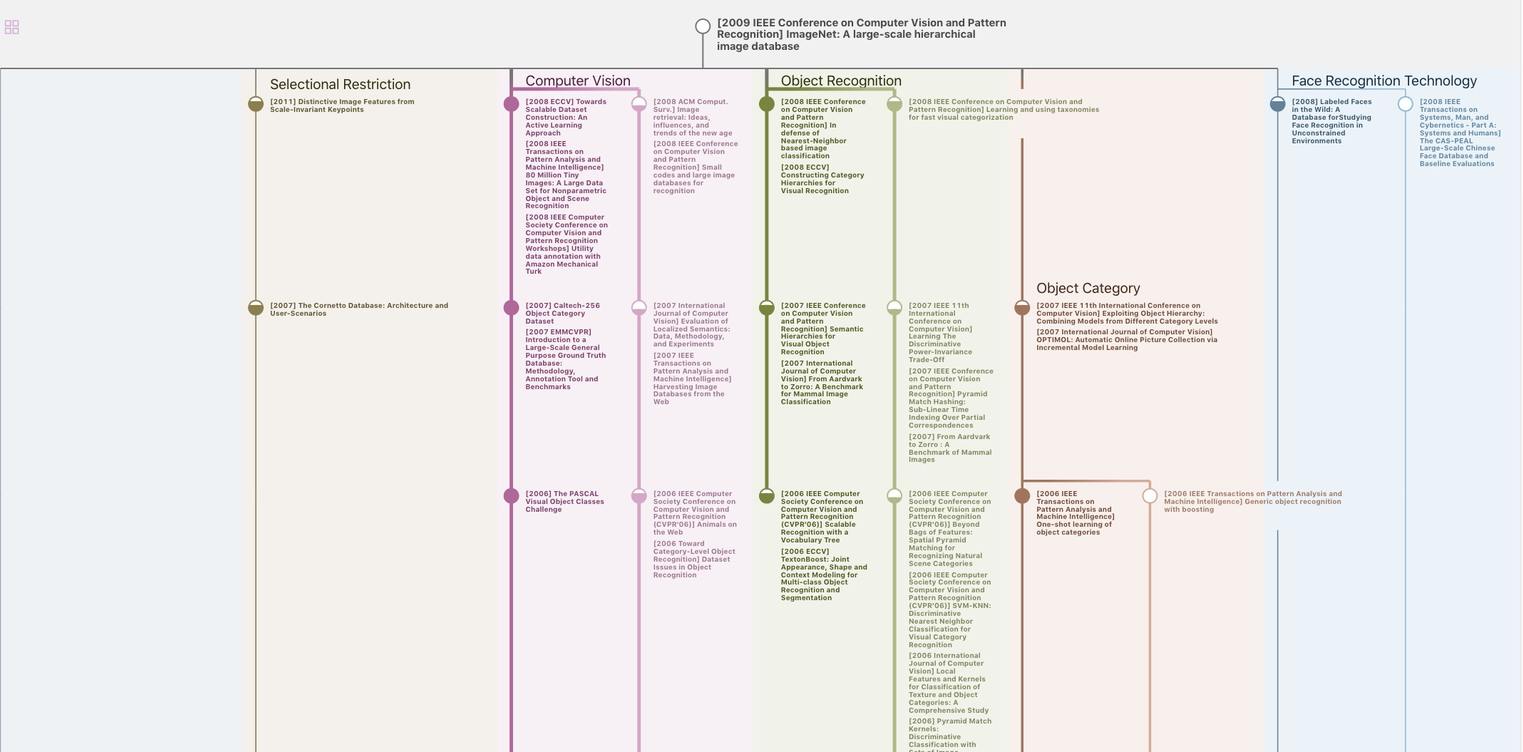
生成溯源树,研究论文发展脉络
Chat Paper
正在生成论文摘要