Unraveling Key Elements Underlying Molecular Property Prediction: A Systematic Study
arXiv (Cornell University)(2022)
摘要
Artificial intelligence (AI) has been widely applied in drug discovery with a major task as molecular property prediction. Despite booming techniques in molecular representation learning, key elements underlying molecular property prediction remain largely unexplored, which impedes further advancements in this field. Herein, we conduct an extensive evaluation of representative models using various representations on the MoleculeNet datasets, a suite of opioids-related datasets and two additional activity datasets from the literature. To investigate the predictive power in low-data and high-data space, a series of descriptors datasets of varying sizes are also assembled to evaluate the models. In total, we have trained 62,820 models, including 50,220 models on fixed representations, 4,200 models on SMILES sequences and 8,400 models on molecular graphs. Based on extensive experimentation and rigorous comparison, we show that representation learning models exhibit limited performance in molecular property prediction in most datasets. Besides, multiple key elements underlying molecular property prediction can affect the evaluation results. Furthermore, we show that activity cliffs can significantly impact model prediction. Finally, we explore into potential causes why representation learning models can fail and show that dataset size is essential for representation learning models to excel.
更多查看译文
关键词
prediction,systematic study
AI 理解论文
溯源树
样例
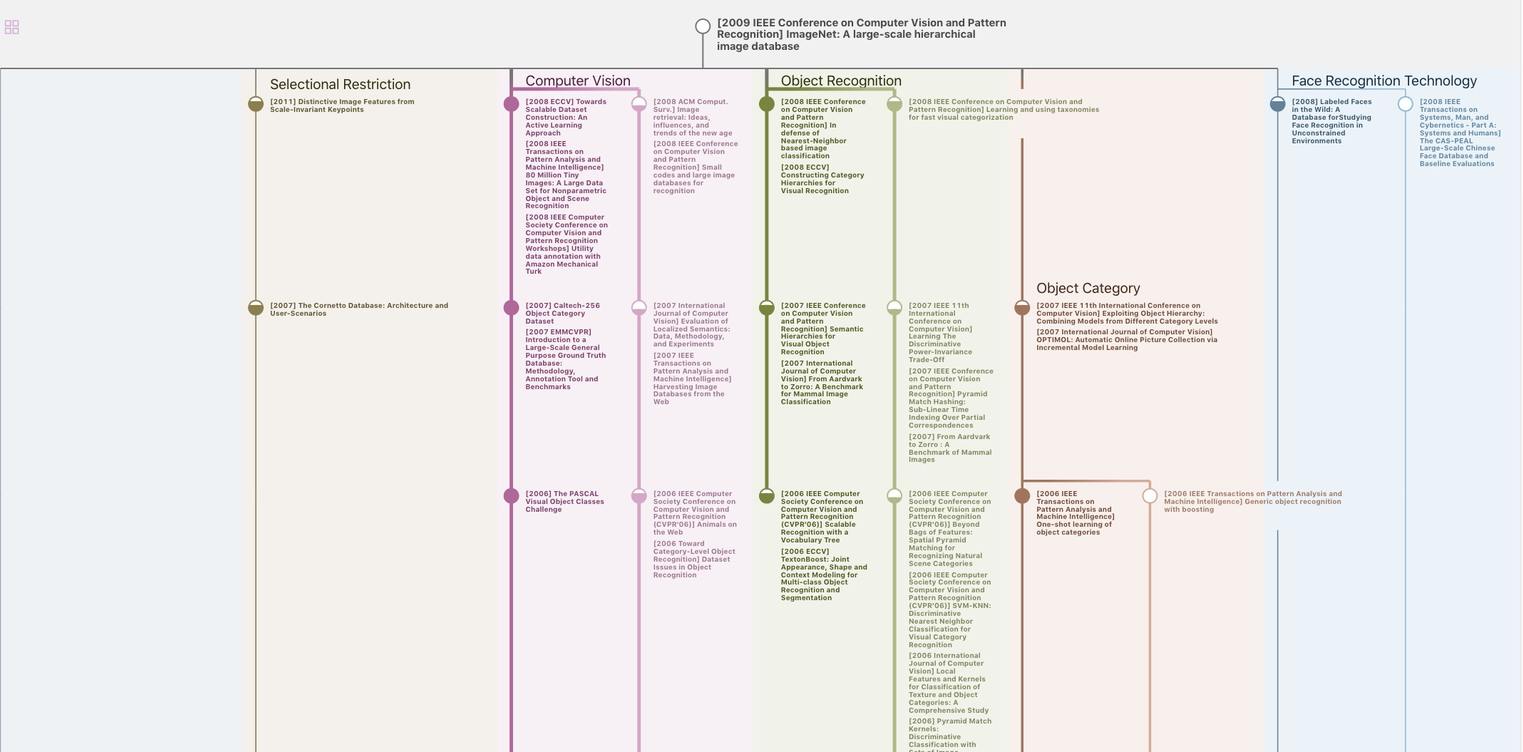
生成溯源树,研究论文发展脉络
Chat Paper
正在生成论文摘要