A General Framework for Evaluating Robustness of Combinatorial Optimization Solvers on Graphs
arXiv (Cornell University)(2021)
摘要
Solving combinatorial optimization (CO) on graphs is among the fundamental tasks for upper-stream applications in data mining, machine learning and operations research. Despite the inherent NP-hard challenge for CO, heuristics, branch-and-bound, learning-based solvers are developed to tackle CO problems as accurately as possible given limited time budgets. However, a practical metric for the sensitivity of CO solvers remains largely unexplored. Existing theoretical metrics require the optimal solution which is infeasible, and the gradient-based adversarial attack metric from deep learning is not compatible with non-learning solvers that are usually non-differentiable. In this paper, we develop the first practically feasible robustness metric for general combinatorial optimization solvers. We develop a no worse optimal cost guarantee thus do not require optimal solutions, and we tackle the non-differentiable challenge by resorting to black-box adversarial attack methods. Extensive experiments are conducted on 14 unique combinations of solvers and CO problems, and we demonstrate that the performance of state-of-the-art solvers like Gurobi can degenerate by over 20% under the given time limit bound on the hard instances discovered by our robustness metric, raising concerns about the robustness of combinatorial optimization solvers.
更多查看译文
关键词
optimization solvers,robustness,combinatorial
AI 理解论文
溯源树
样例
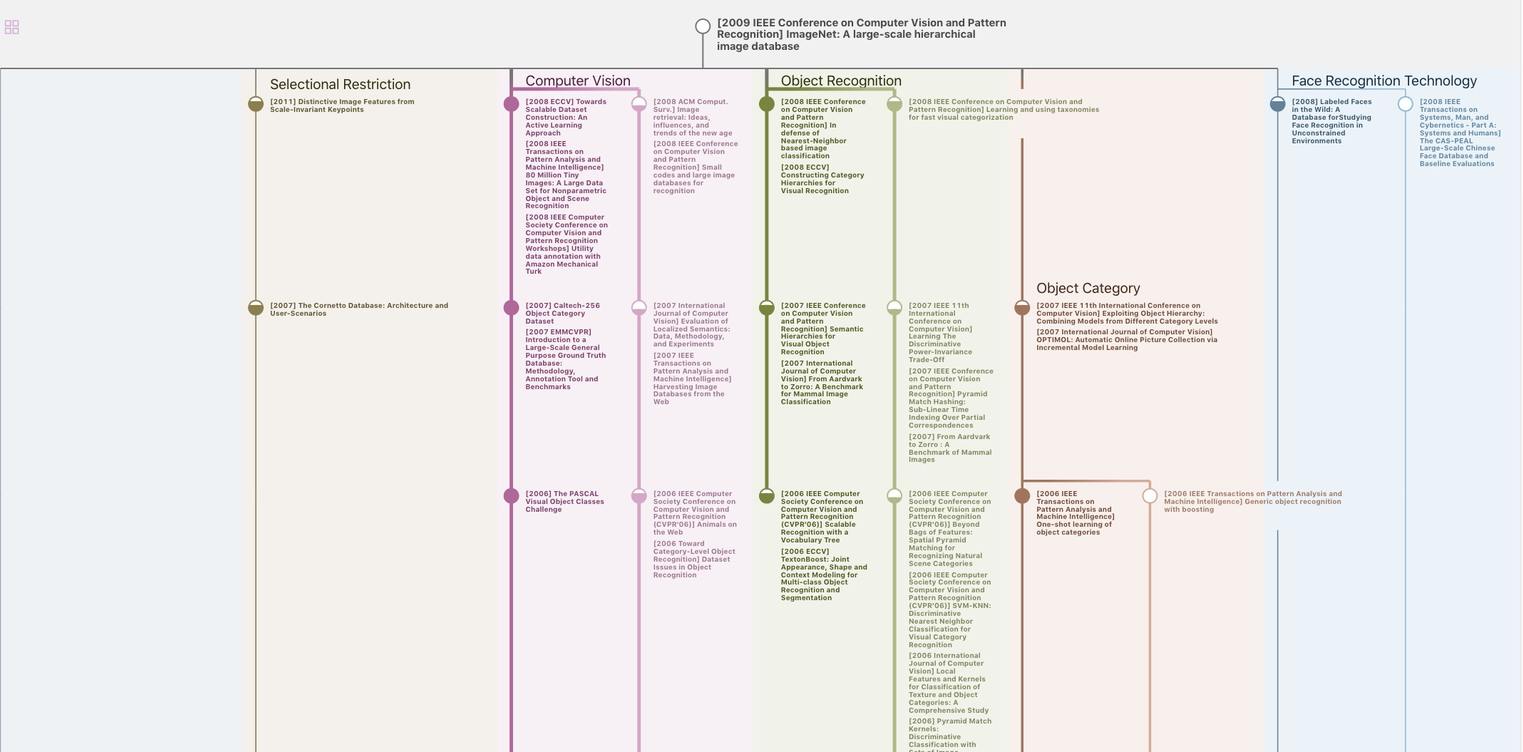
生成溯源树,研究论文发展脉络
Chat Paper
正在生成论文摘要