Concept Grounding with Modular Action-Capsules in Semantic Video Prediction
arXiv (Cornell University)(2020)
摘要
Recent works in video prediction have mainly focused on passive forecasting and low-level action-conditional prediction, which sidesteps the learning of interaction between agents and objects. We introduce the task of semantic action-conditional video prediction, which uses semantic action labels to describe those interactions and can be regarded as an inverse problem of action recognition. The challenge of this new task primarily lies in how to effectively inform the model of semantic action information. To bridge vision and language, we utilize the idea of capsule and propose a novel video prediction model, Modular Action Capsule Network (MAC). Our method is evaluated on two newly designed synthetic datasets, CLEVR-Building-Blocks and Sapien-Kitchen, and one real-world dataset called TowerCreation. Experiments show that given different action labels, MAC can correctly condition on instructions and generate corresponding future frames without need of bounding boxes. We further demonstrate that the trained model can make out-of-distribution generalization, be quickly adapted to new object categories and exploit its learnt features for object detection, showing the progression towards higher-level cognitive abilities.
更多查看译文
关键词
concept grounding,prediction,action-capsules
AI 理解论文
溯源树
样例
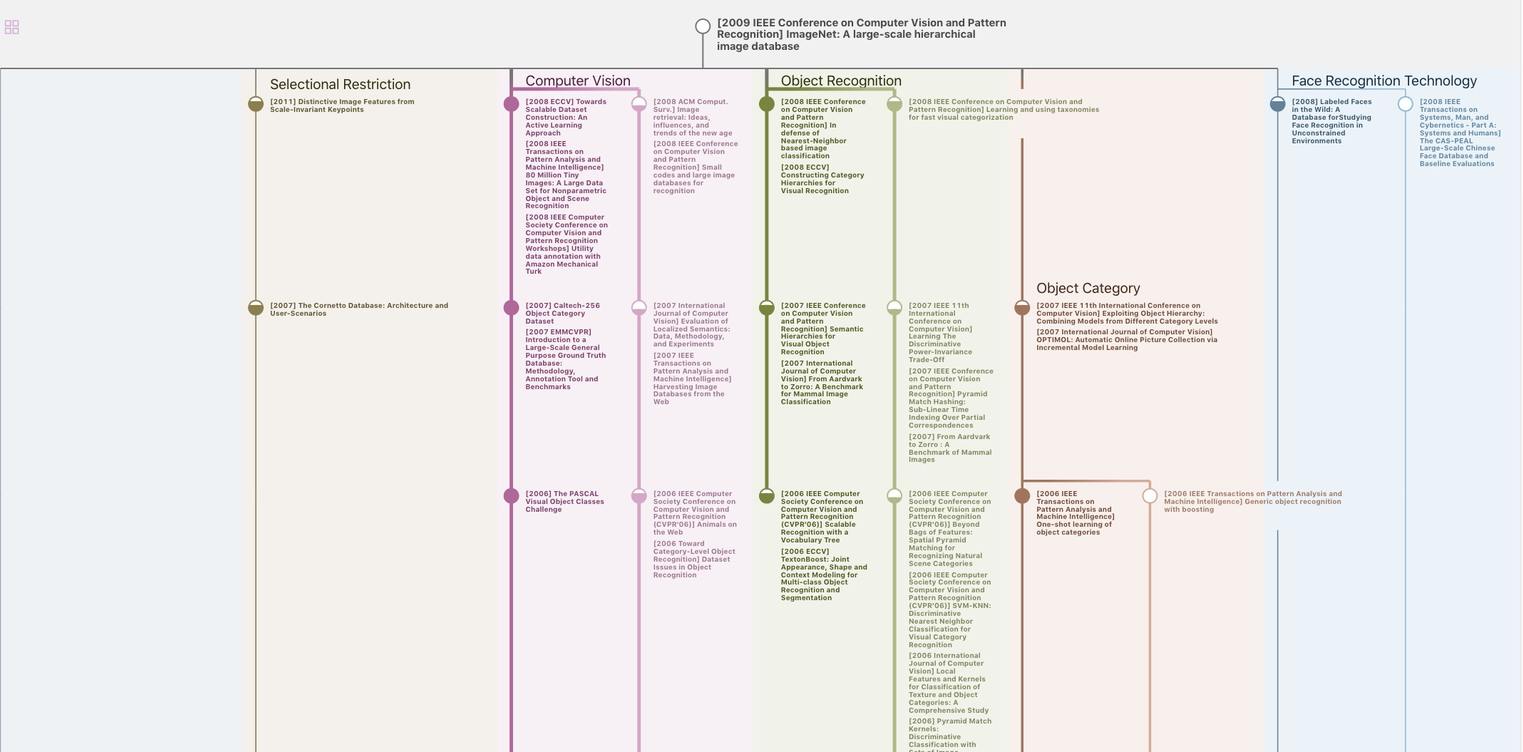
生成溯源树,研究论文发展脉络
Chat Paper
正在生成论文摘要