A broader study of cross-domain few-shot object detection
APPLIED INTELLIGENCE(2023)
摘要
Latest studies on few-shot object detection (FSOD) mainly focuses on achieving better performance in novel class through few-shot fine-tuning. This approach is based on the large amount of annotated data in base class to pre-train models. However, in many scenarios it is difficult or impossible to gather substantial data for the base class of the same domain as the novel class. This leads to the cross-domain few-shot object detection problem. Existing research on scenarios of cross-domain few-shot object detection is only limited to generalization where the base and novel class are from highly similar domains of natural images (i.e., from COCO to VOC). In this respect, we propose the Broader Study of Cross-Domain Few-Shot Object Detection (BSCD-FSOD) benchmark. The generalization is thus possible both from a natural image domain to another, for instance from COCO to ExDark (low-light images) and from a natural image domain to a dissimilar one, for instance from COCO to Clipar1k (artistic media images), to NEU-DET (industrial defect images) or to DOTA (satellite images). We have performed extensive experiments on the proposed benchmark to evaluate state-of-art transfer learning approaches. Research results show that, previous state-of-art methods are no longer state-of-art. Pretraining models on COCO instead of VOC can help them learn a wider feature distribution. The less the domains are similar to those of natural images, the more trainable network components fine-tuning thus requires. The performance of all methods is correlated to both the target domain and the degree of similarity between source and target domain. The results we get show the diversity of data distribution and validate the value of our benchmark, which will offer guidance for future researches.
更多查看译文
关键词
Few-shot object detection, Cross-domain, Transfer learning, Benchmark
AI 理解论文
溯源树
样例
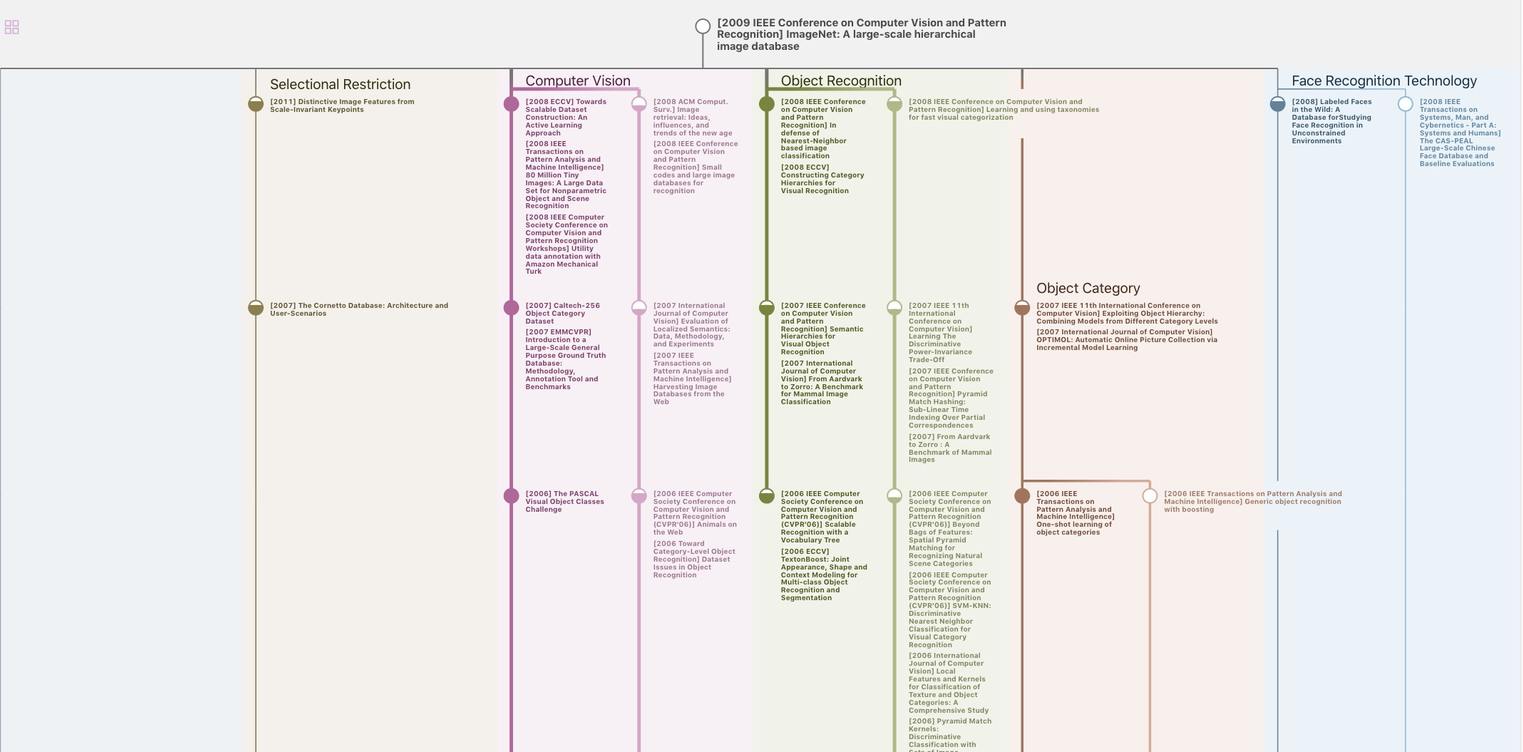
生成溯源树,研究论文发展脉络
Chat Paper
正在生成论文摘要