Semi-Supervised Active Learning for Semantic Segmentation in Unknown Environments Using Informative Path Planning
IEEE ROBOTICS AND AUTOMATION LETTERS(2024)
摘要
Semantic segmentation enables robots to perceive and reason about their environments beyond geometry. Most of such systems build upon deep learning approaches. As autonomous robots are commonly deployed in initially unknown environments, pre-training on static datasets cannot always capture the variety of domains and limits the robot's perception performance during missions. Recently, self-supervised and fully supervised active learning methods emerged to improve robotic vision. These approaches rely on large in-domain pre-training datasets or require substantial human labelling effort. We propose a planning method for semi-supervised active learning of semantic segmentation that substantially reduces human labelling requirements compared to fully supervised approaches. We leverage an adaptive map-based planner guided towards the frontiers of unexplored space with high model uncertainty collecting training data for human labelling. A key aspect of our approach is to combine the sparse high-quality human labels with pseudo labels automatically extracted from highly certain environment map areas. Experimental results show that our method reaches segmentation performance close to fully supervised approaches with drastically reduced human labelling effort while outperforming self-supervised approaches.
更多查看译文
关键词
Semantics,Robots,Labeling,Semantic segmentation,Uncertainty,Planning,Adaptation models,Deep learning for visual perception,motion and path planning,semantic scene understanding
AI 理解论文
溯源树
样例
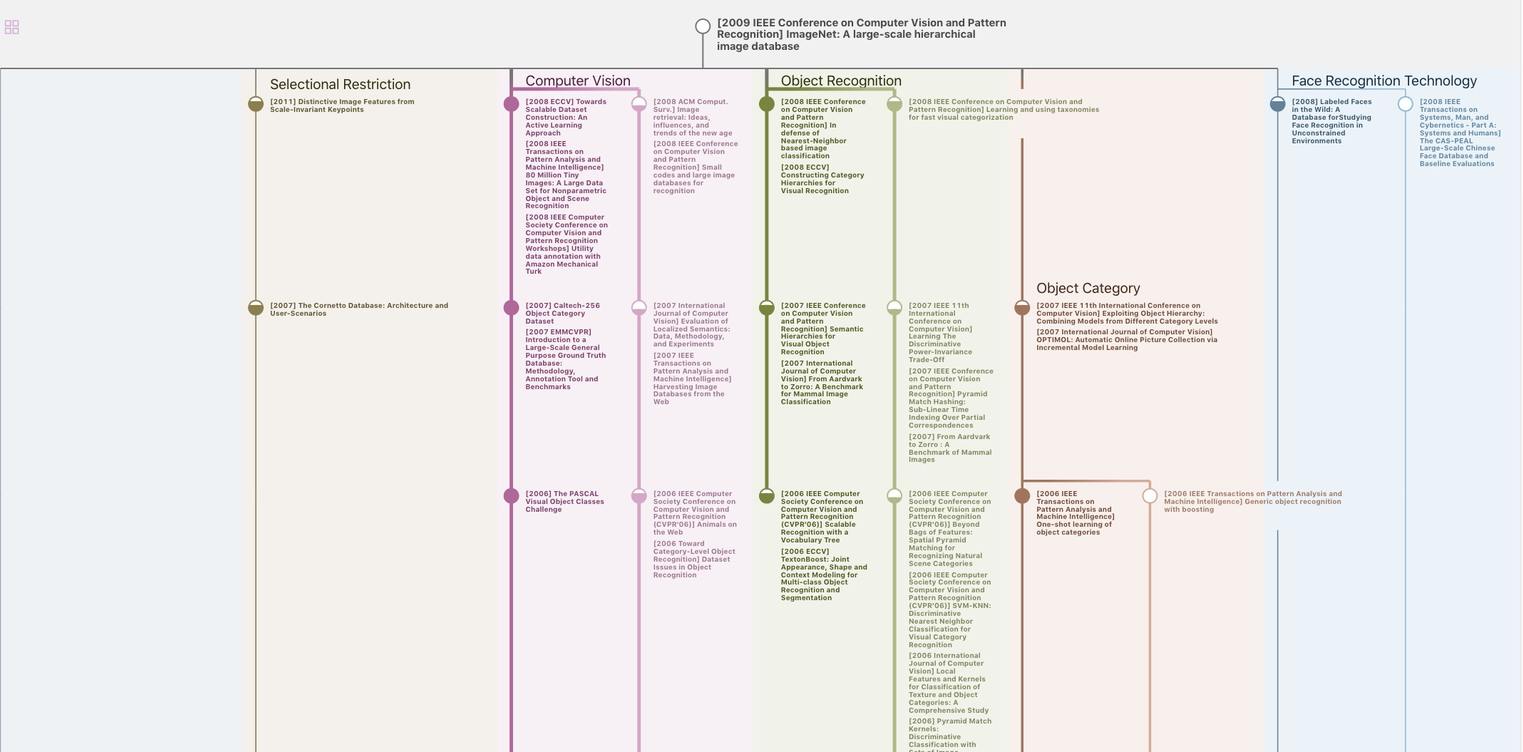
生成溯源树,研究论文发展脉络
Chat Paper
正在生成论文摘要