Knowledge-wrapping method for prediction and evaluation of material removal behavior in robotic belt grinding
MECHANICAL SYSTEMS AND SIGNAL PROCESSING(2024)
摘要
Reliable prediction and evaluation of material removal (MR) is a continuing pursuit in the grinding process. Mechanistic and empirical MR models always suffer from inaccuracies and restricted applicability, whereas data-driven approaches remain deficient in sample dependence, generalization, and physical interpretability. This motivates us to develop a knowledge-wrapping method (KWM) to predict and characterize the material removal behavior in robotic belt grinding. A physical material removal profile model (PHY) that suits the robotic belt grinding is first presented by appealing to the Archard law and Hertzian theory. Next, the knowledge-wrapping matrix is designed to wrap the physical constraints into a form of design matrix by transforming the mechanistic and empirical models into a linear system. The knowledge-wrapping matrix and the experimental data are then connected via a likelihood function with the consideration of measuring noise, yielding a hybrid-driven learning process that preserves interpretability from PHY. Comparative experiments and MR mechanism interpretations are finally presented to demonstrate the effectiveness and superiority of the proposed method.
更多查看译文
关键词
Robotic grinding,Material removal,Physics-data driven,Knowledge learning
AI 理解论文
溯源树
样例
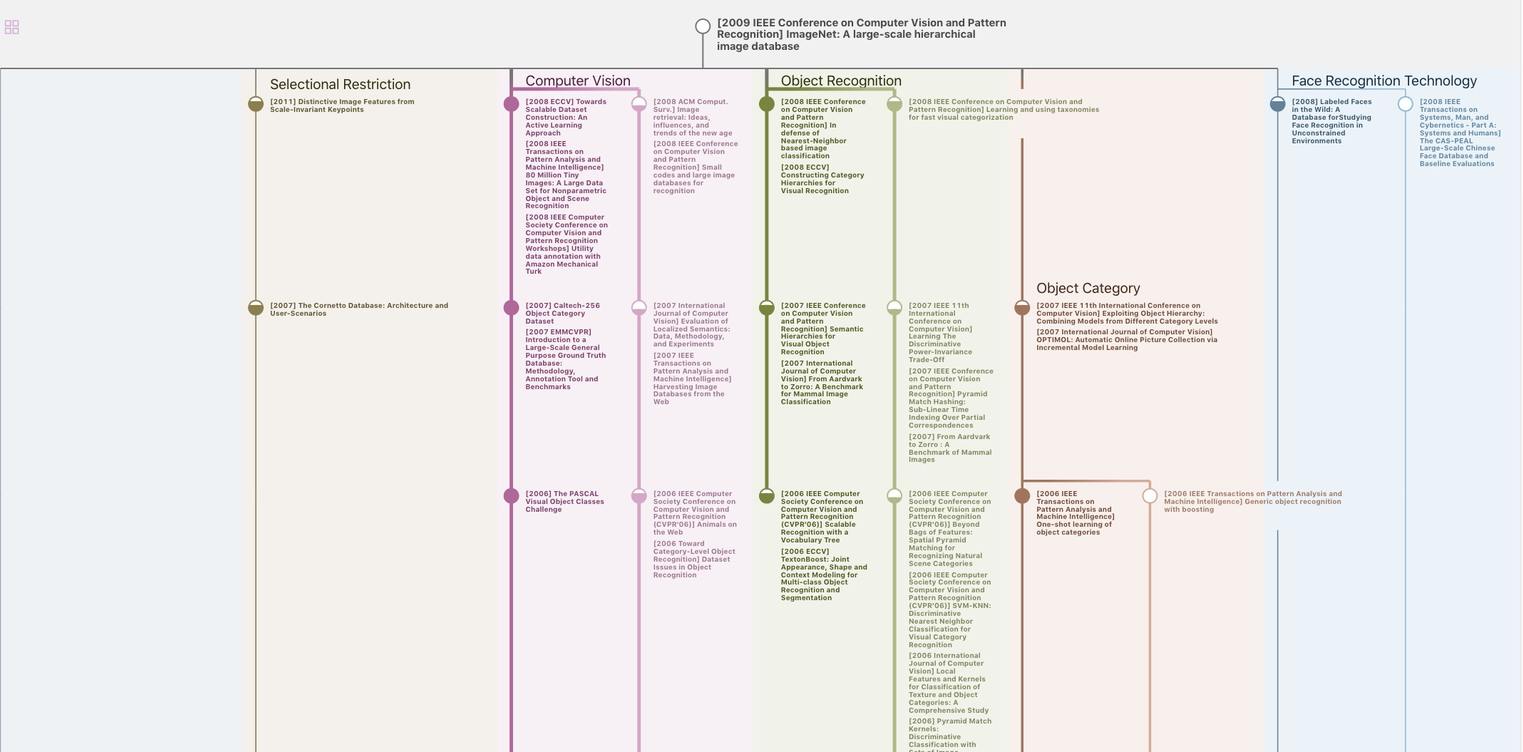
生成溯源树,研究论文发展脉络
Chat Paper
正在生成论文摘要