Enhancing active disturbance rejection design via deep reinforcement learning and its application to autonomous vehicle
EXPERT SYSTEMS WITH APPLICATIONS(2024)
摘要
Taking the velocity regulation of autonomous driving as an example, this paper developed an enhancing active disturbance rejection design employing the deep reinforcement learning-deep deterministic policy gradient (DDPG). In this scheme, the active disturbance rejection control (ADRC) is adopted to online estimate and compensate disturbances and uncertainties, and feasible regions of control parameters are obtained through the Lyapunov method. Then DDPG is combined with ADRC to online adaptively tune control parameters in response to the changing environments, where safety, comfort, and energy-saving are considered in the reward design, and mapping relation between the defined action and state is constructed for the maximal reward. Besides, numerical simulations demonstrate the better performance and stronger robustness of the enhancing design when facing uncertainties, sensor noise, and mechanical faults, and comfort and energy consumption have also been improved to some extent compared with the general ADRC and model predictive control (MPC).
更多查看译文
关键词
Autonomous vehicle,Active disturbance rejection control (ADRC),Stability analysis,Deep reinforcement learning,Enhancing design
AI 理解论文
溯源树
样例
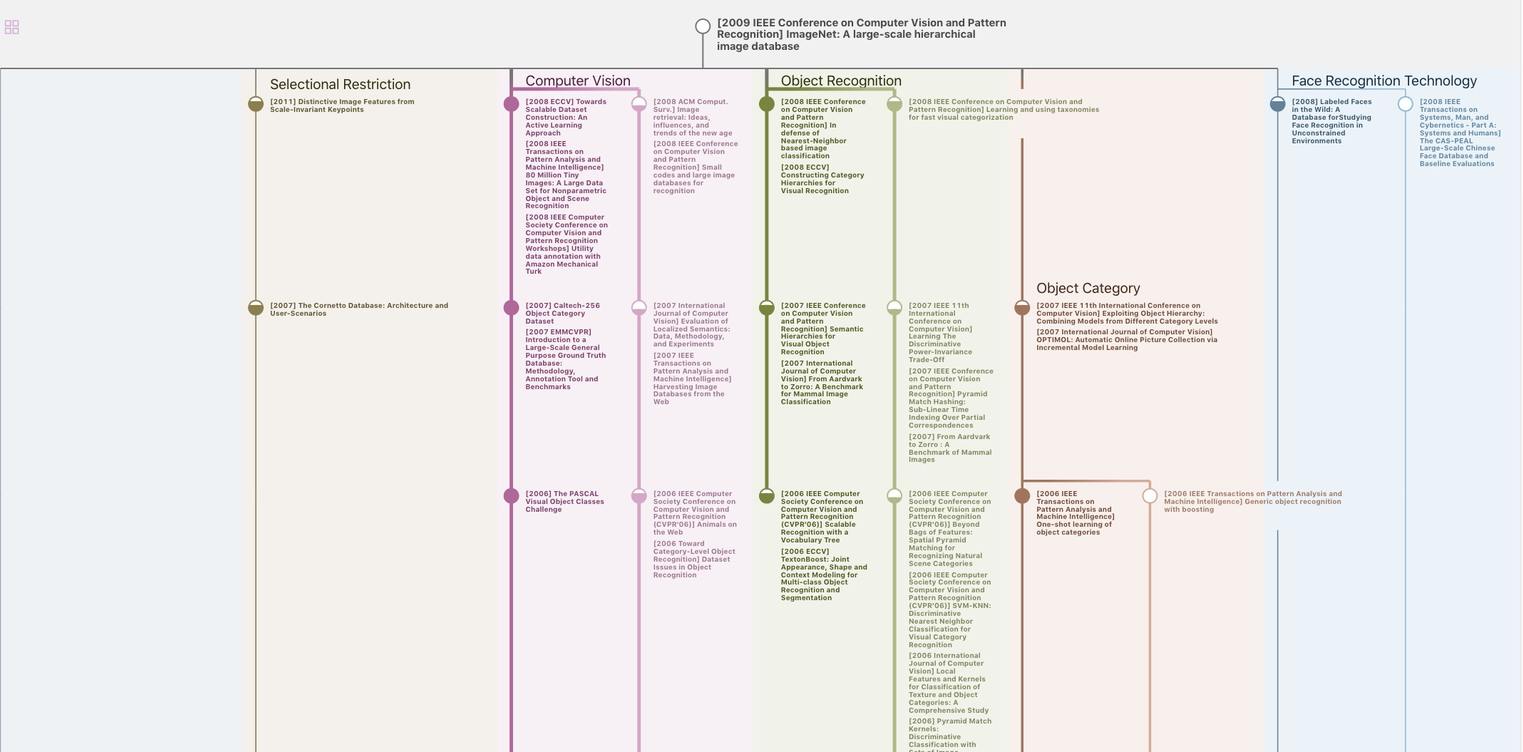
生成溯源树,研究论文发展脉络
Chat Paper
正在生成论文摘要